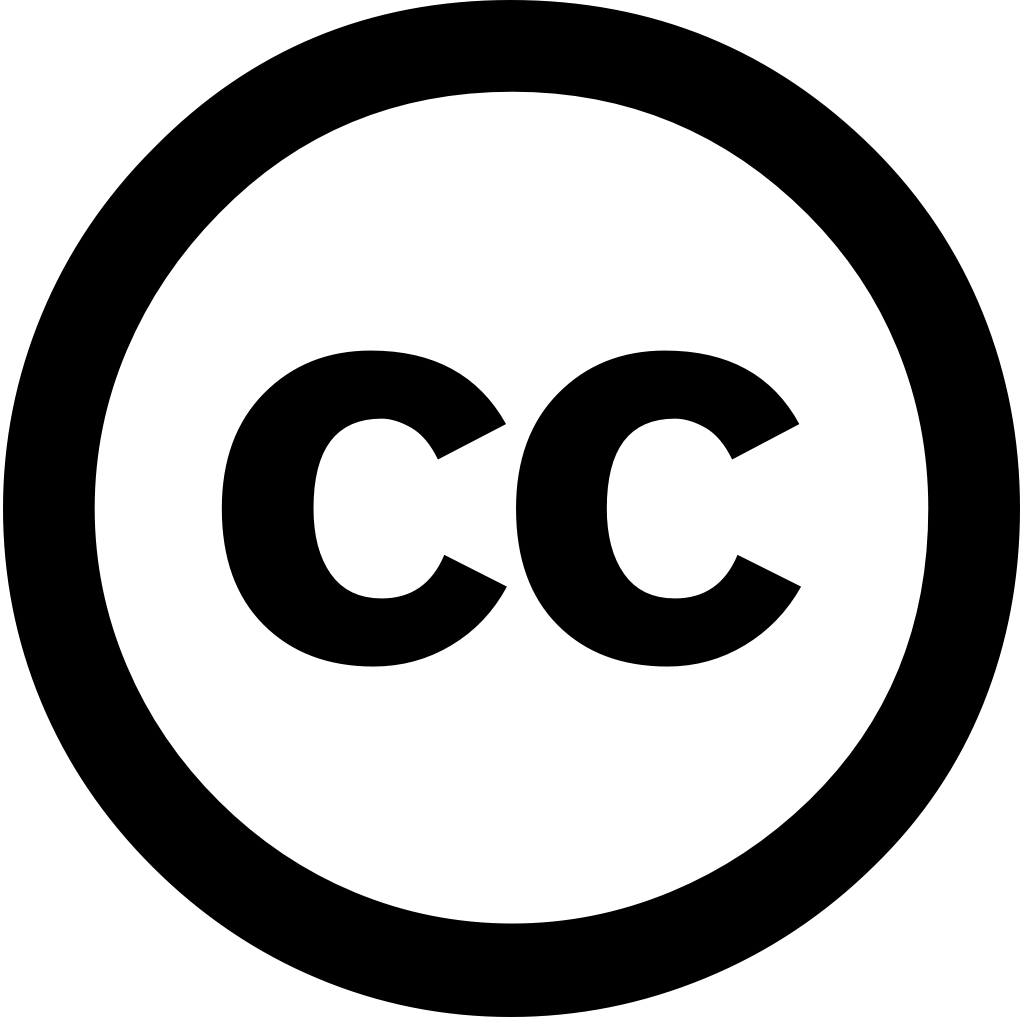
Agriculture, Journal Year: 2025, Volume and Issue: 15(9), P. 943 - 943
Published: April 26, 2025
Traditional pesticide application methods pose systemic threats to sustainable agriculture due inefficient spraying practices and ecological contamination. Although agricultural drones demonstrate potential address these challenges, they face critical limitations in energy-constrained complete coverage path planning for field operations. This study proposes a novel BiLG-D3QN algorithm by integrating deep reinforcement learning with Bi-LSTM Bi-GRU architectures, specifically designed optimize segmented under payload-dependent energy consumption constraints. The methodology encompasses four components: payload-energy modeling, soybean cultivation area identification using Google Earth Engine-derived spatial distribution data, raster map construction, enhanced implementation. Through simulation experiments, the demonstrated superior efficiency, outperforming DDQN 13.45%, D3QN 12.27%, Dueling DQN 14.62%, A-Star 15.59%, PPO 22.15%. Additionally, achieved an average redundancy rate of only 2.45%, which is significantly lower than that (18.89%), (17.59%), (21.54%), (25.12%). These results highlight notable advantages addressing challenges tasks UAV applications.
Language: Английский