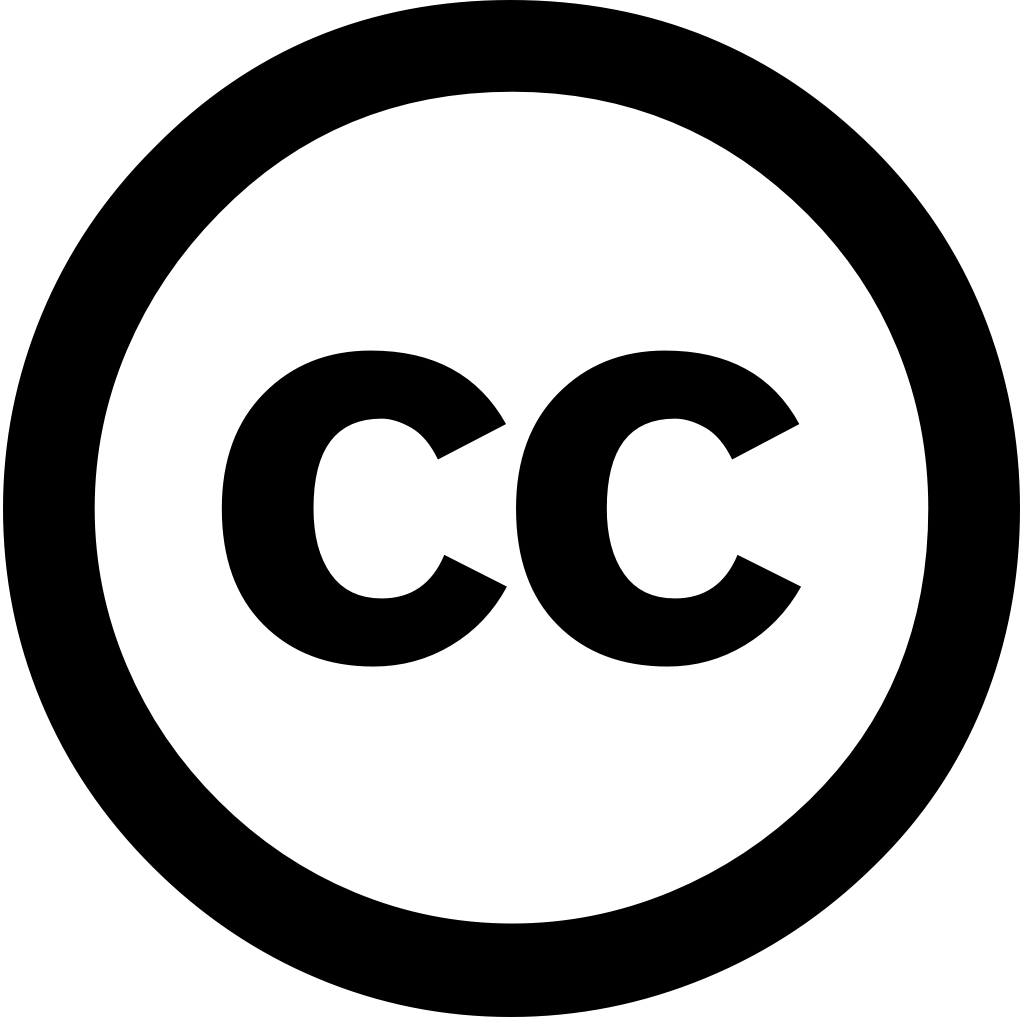
Agriculture, Journal Year: 2025, Volume and Issue: 15(7), P. 765 - 765
Published: April 2, 2025
Accurate apple yield estimation is essential for effective orchard management, market planning, and ensuring growers’ income. However, complex conditions, such as dense foliage occlusion overlapping fruits, present challenges to large-scale estimation. This study introduces APYOLO, an enhanced detection algorithm based on improved YOLOv11, integrated with the DeepSORT tracking improve both accuracy operational speed. APYOLO incorporates a multi-scale channel attention (MSCA) mechanism prior distribution intersection over union (EnMPDIoU) loss function enhance target localization recognition under environments. Experimental results demonstrate that outperforms original YOLOv11 by improving [email protected], [email protected]–0.95, accuracy, recall 2.2%, 2.1%, 0.8%, 2.3%, respectively. Additionally, combination of unique ID region line (ROL) strategy in further boosts 84.45%, surpassing performance method alone. provides more precise efficient system estimation, offering strong technical support intelligent refined management.
Language: Английский