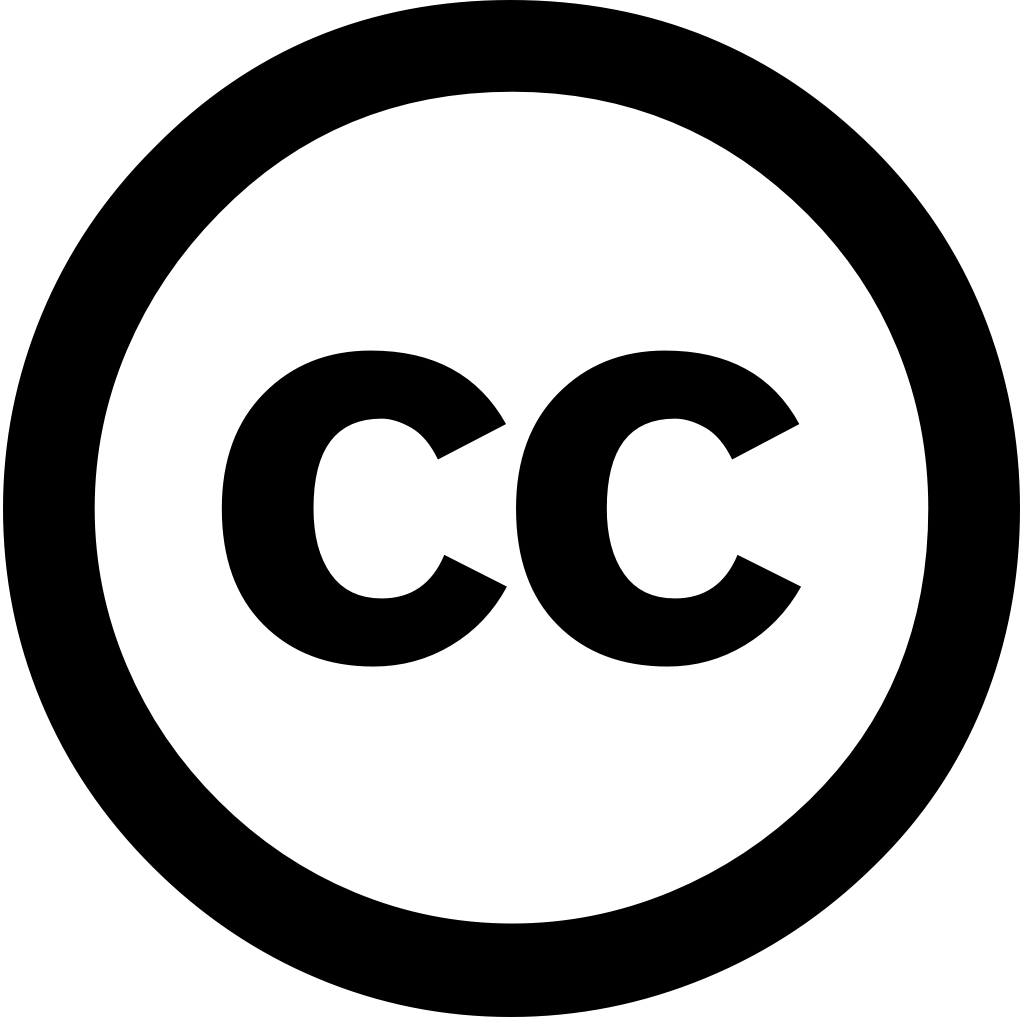
Frontiers in Neuroscience, Journal Year: 2025, Volume and Issue: 18
Published: Jan. 10, 2025
Excessive alcohol consumption negatively impacts physical and psychiatric health, lifestyle, societal interactions. Chronic abuse alters brain structure, leading to use disorder (AUD), a condition requiring early diagnosis for effective management. Current diagnostic methods, primarily reliant on subjective questionnaires, could benefit from objective measures. The study proposes novel EEG-based classification approach, focusing connectivity (EC) derived resting-state EEG signals in combination with support vector machine (SVM) algorithms. EC estimation is performed using the partial directed coherence (PDC) technique. analysis conducted an dataset comprising 35 individuals AUD healthy controls (HCs). methodology evaluates efficacy of features distinguishing between HC subsequently develops assesses technique matrices SVM. proposed demonstrated promising performance, achieving peak accuracy 94.5% area under curve (AUC) 0.988, specifically frequency bands 29, 36, 45, 46, 52. Additionally, feature reduction techniques applied PDC adjacency gamma band further improved outcomes. SVM-based achieved 96.37 ± 0.45%, showcasing enhanced performance through utilization reduced matrices. These results highlight potential developed algorithm as robust tool detection, enhancing precision beyond methods. Incorporating can inform tailored treatment strategies, contributing management AUD.
Language: Английский