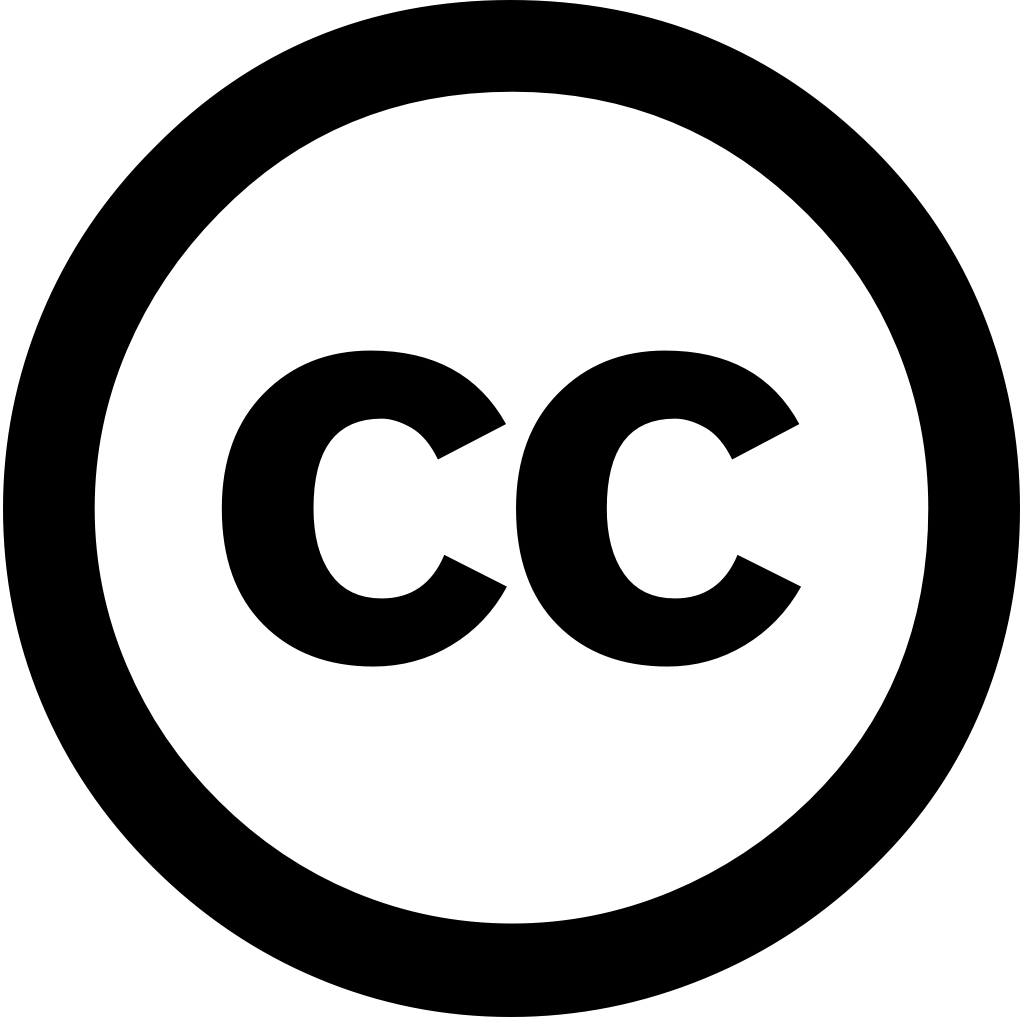
bioRxiv (Cold Spring Harbor Laboratory), Journal Year: 2024, Volume and Issue: unknown
Published: Nov. 15, 2024
Abstract Accurate survival prediction is vital for optimizing treatment strategies in clinical practice. The advent of high-throughput multi-omics data and computational methods has enabled machine learning (ML) models analysis. However, handling high-dimensional omics remains challenging. This study introduces the Cancer Patient Survival Model (CPSM), an R package developed to provide individualized predictions through a fully integrated reproducible pipeline. CPSM encompasses nine modules that streamline modeling workflow, organized into four key stages: (1) Data Preprocessing Normalization, (2) Feature Selection, (3) Prediction Development, (4) Visualization. visual tools facilitate interpretation predictions, enhancing decision-making. By providing end-to-end solution integration analysis, not only enhances precision but also aids discovering clinically relevant biomarkers. Availability Implementation Package freely available at GitHub URL: https://github.com/hks5august/CPSM
Language: Английский