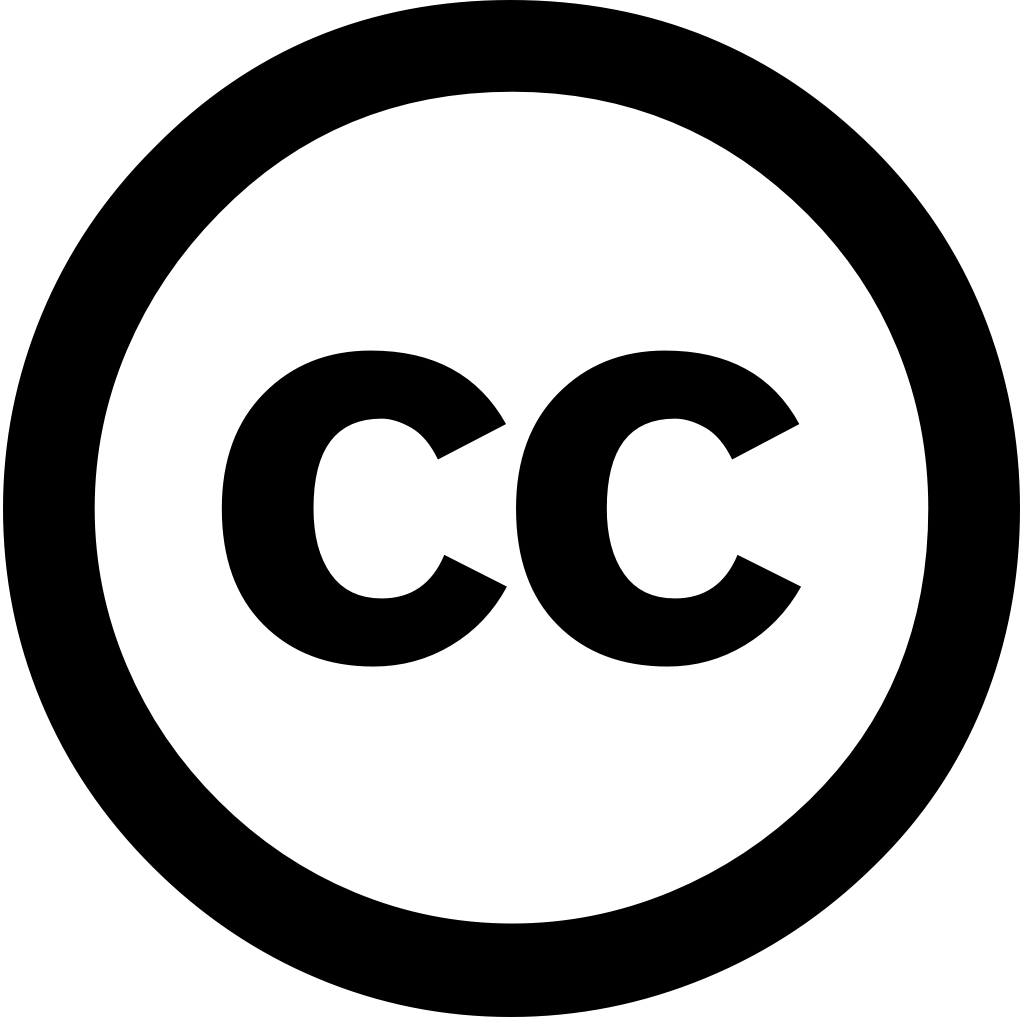
Algorithms, Journal Year: 2024, Volume and Issue: 17(2), P. 60 - 60
Published: Jan. 30, 2024
Efficient yet sufficient exploration remains a critical challenge in reinforcement learning (RL), especially for Markov Decision Processes (MDPs) with vast action spaces. Previous approaches have commonly involved projecting the original space into latent or employing environmental masks to reduce possibilities. Nevertheless, these methods often lack interpretability rely on expert knowledge. In this study, we introduce novel method automatically reducing environments discrete spaces while preserving interpretability. The proposed approach learns state-specific dual purpose: (1) eliminating actions minimal influence MDP and (2) aggregating identical behavioral consequences within MDP. Specifically, concept called Bisimulation Metrics Actions by States (BMAS) quantify of design dedicated mask model ensure their binary nature. Crucially, present practical procedure training model, leveraging transition data collected any RL policy. Our is designed be plug-and-play adaptable all policies, validate its effectiveness, an integration two prominent algorithms, DQN PPO, performed. Experimental results obtained from Maze, Atari, μRTS2 reveal substantial acceleration process noteworthy performance improvements facilitated introduced approach.
Language: Английский