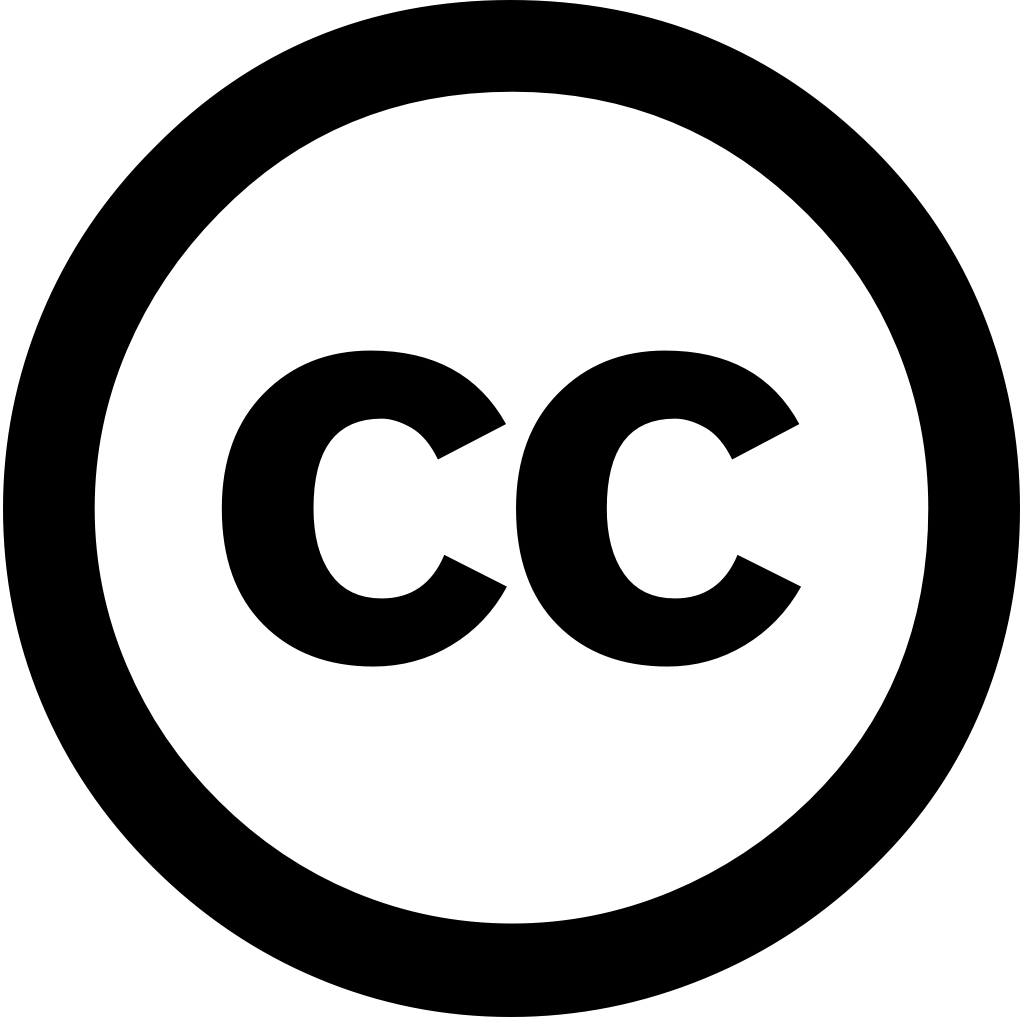
Machines, Journal Year: 2024, Volume and Issue: 12(12), P. 879 - 879
Published: Dec. 4, 2024
The safe operation of aero-engines is crucial for ensuring flight safety, and effective fault detection methods are fundamental to achieving this objective. In paper, we propose a novel approach that integrates an auto-encoder with long short-term memory (LSTM) networks self-attention mechanism the anomaly aero-engine time-series data. dataset utilized in study was simulated from real data injected information. A model developed utilizing normal samples training faulty testing. LSTM processes through encoder–decoder architecture, extracting latent representations reconstructing original inputs. Furthermore, captures long-range dependencies significant features within sequences, thereby enhancing accuracy model. Comparative analyses traditional auto-encoder, as well one-class support vector machines (OC-SVM) isolation forests (IF), reveal experimental results substantiate feasibility effectiveness proposed method, highlighting its potential value engineering applications.
Language: Английский