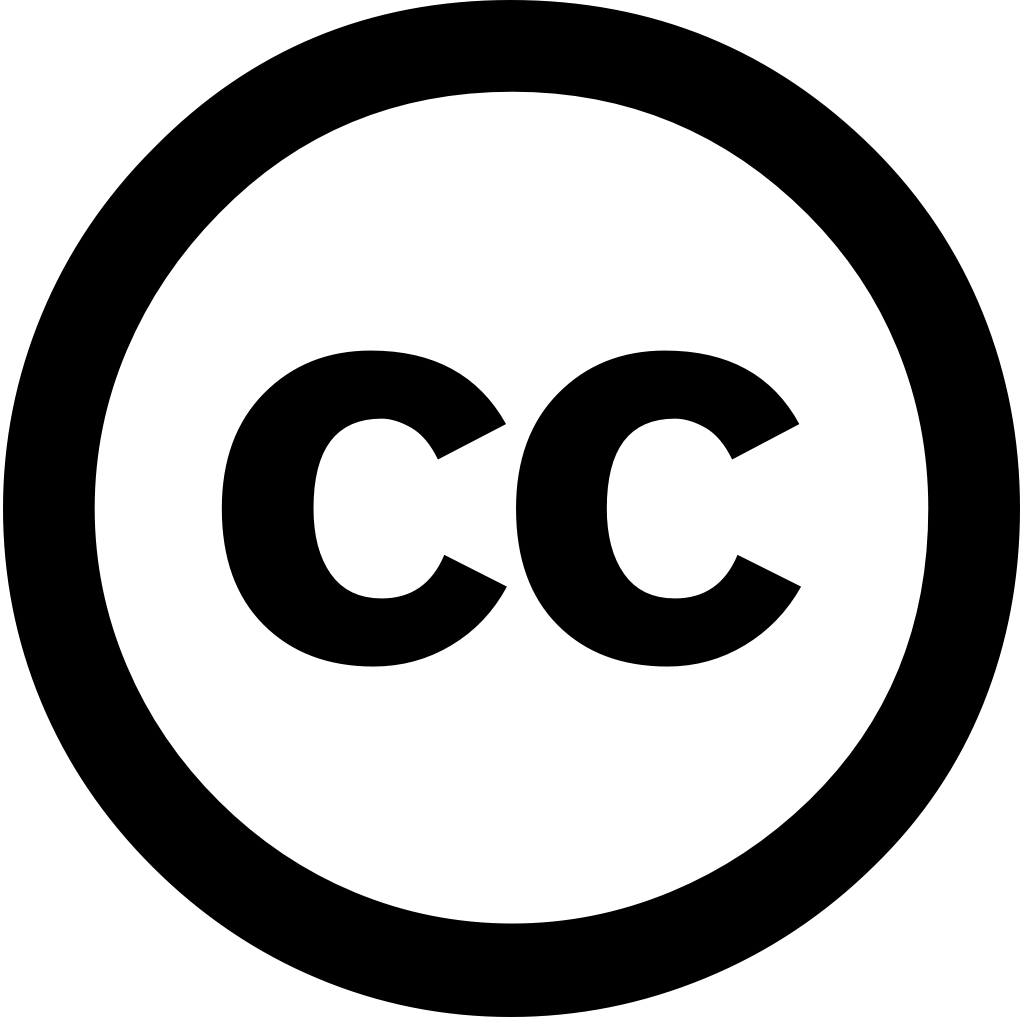
Highlights in Science Engineering and Technology, Journal Year: 2025, Volume and Issue: 134, P. 240 - 254
Published: March 30, 2025
Existing prediction algorithms face challenges in terms of accuracy and training speed, which hinders high-efficiency, high-accuracy power load forecasting. This paper proposes a combined model to address these issues. To overcome the Bidirectional Gated Recurrent Unit (BiGRU)'s limitations capturing long-term dependencies handling complex time-series data, Convolutional Neural Network (CNN) module is introduced extract local features enhance model's feature representation. Additionally, Multi-Head Attention (MH-Attention) incorporated dynamically assign weights different time steps, improving adaptivity focus on key features. For hyperparameter optimization, an Improved Sparrow Search Algorithm (ISSA) proposed, addresses traditional SSA’s tendency fall into optima slow convergence by incorporating adaptive update mechanism hybrid heuristic strategy. The validated using plant dataset from Quanzhou, with results showing excellent forecasting ability: R2=0.9955, RMSE=56.9596, MAE=34.6080. Comparison other models demonstrates improved performance, R2 increasing 0.3%-0.65%, RMSE decreasing 6.38%-35.96%, MAE reducing 26.54%-49.52%. These confirm model’s effectiveness superiority.
Language: Английский