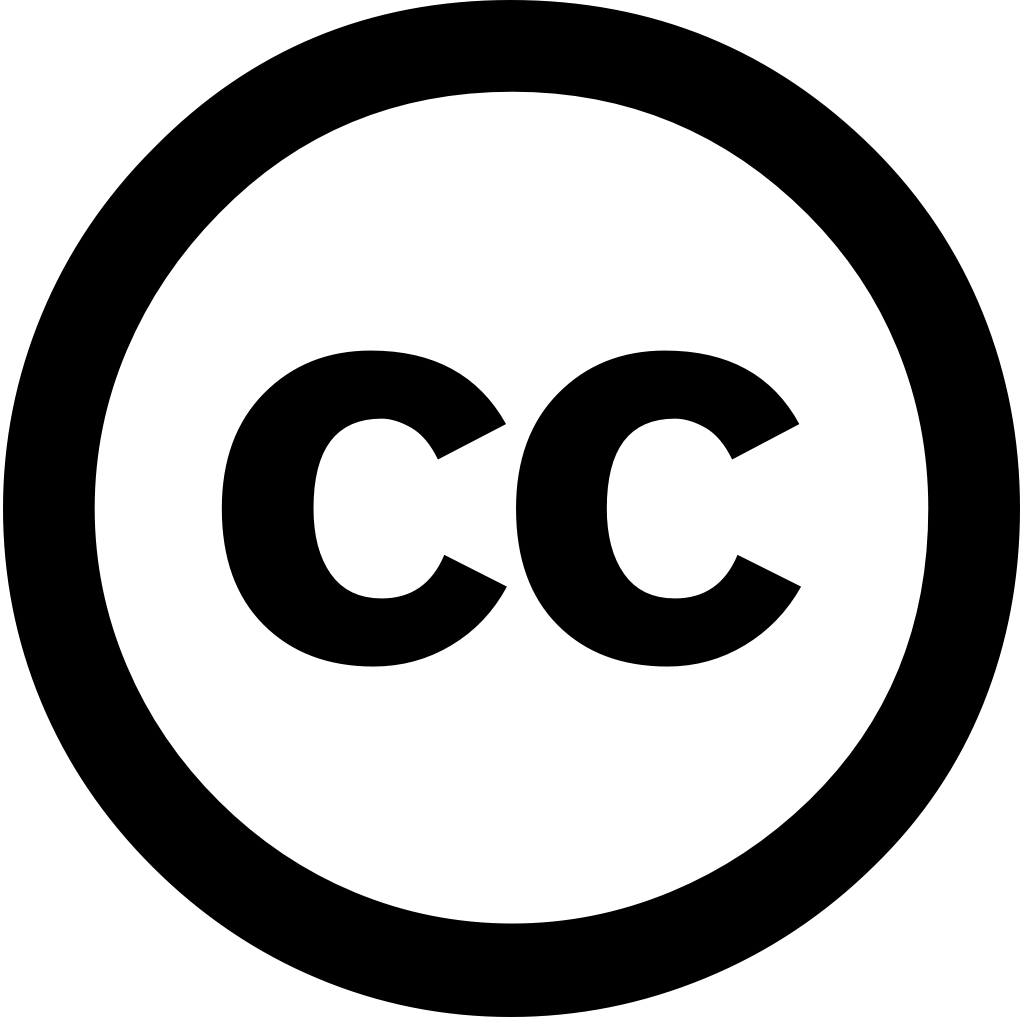
Smart Agricultural Technology, Journal Year: 2024, Volume and Issue: 8, P. 100512 - 100512
Published: Aug. 1, 2024
Language: Английский
Smart Agricultural Technology, Journal Year: 2024, Volume and Issue: 8, P. 100512 - 100512
Published: Aug. 1, 2024
Language: Английский
Phytopathology, Journal Year: 2024, Volume and Issue: 114(5), P. 855 - 868
Published: April 9, 2024
Disaster plant pathology addresses how natural and human-driven disasters impact diseases the requirements for smart management solutions. Local to global drivers of disease change in response disasters, often creating environments more conducive disease. Most have indirect effects on health through factors such as disrupted supply chains damaged infrastructure. There is also potential direct from pathogen or vector dispersal due floods, hurricanes, human migration driven by war. Pulse stressors hurricanes war require rapid responses, whereas press climate leave time adaptation but may ultimately cause broader challenges. Smart solutions can be deployed digital agriculture decision support systems supporting disaster preparedness optimized humanitarian aid across scales. Here, we use framework synthesize outline maintain food security catastrophic scenarios. We recommend actions improving before following including (i) strengthening regional cooperation, (ii) capacity building implementation new technologies, (iii) effective clean seed that act quickly replace lost (iv) resilient biosecurity infrastructure risk assessment ready implementation, (v) adapt rapidly unexpected [Formula: see text] Copyright © 2024 The Author(s). This an open access article distributed under CC BY 4.0 International license.
Language: Английский
Citations
6Environment Development and Sustainability, Journal Year: 2024, Volume and Issue: unknown
Published: June 29, 2024
Language: Английский
Citations
6IOP Conference Series Earth and Environmental Science, Journal Year: 2024, Volume and Issue: 1285(1), P. 012026 - 012026
Published: Jan. 1, 2024
Abstract The food demand is ever increasing each year and to meet this precision agricultural approach using machine learning tools play an important role. Precision irrigation systems integrate cutting-edge technologies, such as sensors, controllers, data analytics internet, achieve sustainability in agriculture maximize water use so improve crop production while minimizing wastage climate impact. main purpose of article find out the precise requirements for a particular area land by soil moisture sensors. These sensors provide real-time that transmitted central control unit, which utilizes driven algorithms analyze levels controls supply. Furthermore, model developed offers remote monitoring capabilities, enabling farmers access manage system from anywhere mobile or web application. This feature allows remotely adjust schedules, receive alerts notifications, track consumption, promoting convenient efficient management resources. Thus effective techniques irrigation, controlling quality, will accomplish optimizing usage intern optimizes yield.
Language: Английский
Citations
5Horticulturae, Journal Year: 2024, Volume and Issue: 10(4), P. 336 - 336
Published: March 28, 2024
Accurate and rapid determination of moisture content is essential in crop production decision-making for irrigation. Near-infrared (NIR) spectroscopy has been shown to be a promising method determining various agricultural products, including herbs vegetables. This study tested the hypothesis that NIR effective accurately measuring Genovese basil (Ocimum basilicum L.), with objective developing respective calibration model. Spectral data were obtained from total 120 leaf samples over period six days. These included freshly harvested detached leaves, as well those left ambient air 1–6 Five spectra taken each using handheld spectrophotometer, which covers first second overtones spectral region: 950–1650 nm. After acquisition, leaves weighed fresh mass then put an oven 72 h at 80 °C determine dry weight calculate reference content. The model was developed multivariate analysis MATLAB, preprocessing regression modeling. 75% used training 25% validation. final demonstrates strong performance metrics. root mean square error (RMSEC) 2.9908, cross-validation (RMSECV) 3.2368, prediction (RMSEP) reaches 2.4675. coefficients (R2C) (R2CV) are consistent, values 0.829 0.80, respectively. model’s predictive ability indicated by coefficient (R2P) 0.86. range ratio (RER) stands 11.045—highlighting its performance. Our investigation, spectrophotometry, confirms NIR’s usefulness determination. offers valuable insights irrigation management.
Language: Английский
Citations
5Smart Agricultural Technology, Journal Year: 2024, Volume and Issue: 8, P. 100512 - 100512
Published: Aug. 1, 2024
Language: Английский
Citations
5