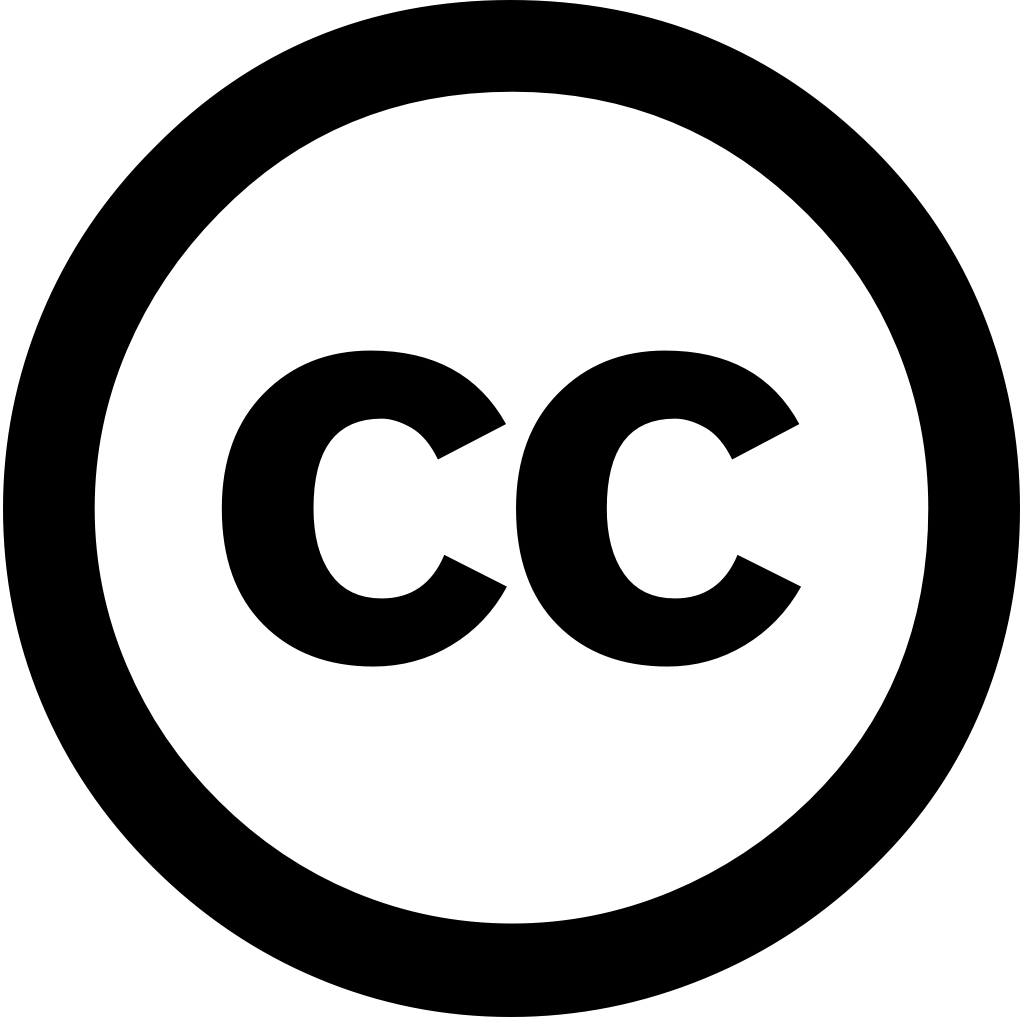
IEEE Access, Journal Year: 2024, Volume and Issue: 12, P. 108248 - 108262
Published: Jan. 1, 2024
Language: Английский
IEEE Access, Journal Year: 2024, Volume and Issue: 12, P. 108248 - 108262
Published: Jan. 1, 2024
Language: Английский
Applied Sciences, Journal Year: 2022, Volume and Issue: 12(19), P. 9820 - 9820
Published: Sept. 29, 2022
Deepfake is utilized in synthetic media to generate fake visual and audio content based on a person’s existing media. The deepfake replaces face voice with make it realistic-looking. Fake generation unethical threat the community. Nowadays, deepfakes are highly misused cybercrimes for identity theft, cyber extortion, news, financial fraud, celebrity obscenity videos blackmailing, many more. According recent Sensity report, over 96% of obscene content, most victims being from United Kingdom, States, Canada, India, South Korea. In 2019, cybercriminals generated chief executive officer call his organization ask them transfer $243,000 their bank account. crimes rising daily. detection big challenge has high demand digital forensics. An advanced research approach must be built protect blackmailing by detecting content. primary aim our study detect using an efficient framework. A novel predictor (DFP) hybrid VGG16 convolutional neural network architecture proposed this study. dataset real faces building techniques. Xception, NAS-Net, Mobile Net, learning techniques employed comparison. DFP achieved 95% precision 94% accuracy detection. Our outperformed other state-of-the-art studies. helps cybersecurity professionals overcome deepfake-related accurately saving blackmailing.
Language: Английский
Citations
81CAAI Transactions on Intelligence Technology, Journal Year: 2024, Volume and Issue: 9(4), P. 790 - 804
Published: Jan. 4, 2024
Abstract Detecting brain tumours is complex due to the natural variation in their location, shape, and intensity images. While having accurate detection segmentation of would be beneficial, current methods still need solve this problem despite numerous available approaches. Precise analysis Magnetic Resonance Imaging (MRI) crucial for detecting, segmenting, classifying medical diagnostics. a vital component diagnosis, it requires precise, efficient, careful, reliable image techniques. The authors developed Deep Learning (DL) fusion model classify reliably. models require large amounts training data achieve good results, so researchers utilised augmentation techniques increase dataset size models. VGG16, ResNet50, convolutional deep belief networks extracted features from MRI Softmax was used as classifier, set supplemented with intentionally created images addition genuine ones. two DL were combined proposed generate model, which significantly increased classification accuracy. An openly accessible internet test model's performance, experimental results showed that achieved accuracy 98.98%. Finally, compared existing methods, outperformed them significantly.
Language: Английский
Citations
15Multimedia Tools and Applications, Journal Year: 2024, Volume and Issue: 83(36), P. 84095 - 84120
Published: April 6, 2024
Abstract This study aims to improve the performance of organic recyclable waste through deep learning techniques. Negative impacts on environmental and Social development have been observed relating poor segregation schemes. Separating from can lead a faster more effective recycling process. Manual classification is time-consuming, costly, less accurate Automated in proposed work uses Improved Deep Convolutional Neural Network (DCNN). The dataset 2 class category with 25077 images divided into 70% training 30% testing images. metrics used are Accuracy, Missed Detection Rate (MDR), False (FDR). results DCNN compared VGG16, VGG19, MobileNetV2, DenseNet121, EfficientNetB0 after transfer learning. Experimental show that image accuracy model reaches 93.28%.
Language: Английский
Citations
8Scientific Reports, Journal Year: 2023, Volume and Issue: 13(1)
Published: Sept. 5, 2023
A new system based on binary Deep Learning (DL) convolutional neural networks has been developed to recognize specific retinal abnormality signs Optical Coherence Tomography (OCT) images useful for clinical practice. Images from the local hospital database were retrospectively selected 2017 2022. labeled by two specialists and included central fovea cross-section OCTs. Nine models using Visual Geometry Group 16 architecture distinguish healthy versus abnormal retinas identify eight different signs. total of 21,500 OCT screened, 10,770 OCTs in study. The achieved high accuracy identifying pathological signs, ranging 93 99%. Accurately detecting is crucial patient care. This study aimed related pathologies, aiding ophthalmologists diagnosis. high-accuracy identified making it a diagnostic aid. Labelled remain challenge, but our approach reduces dataset creation time shows DL models' potential improve ocular pathology diagnosis decision-making.
Language: Английский
Citations
16IEEE Access, Journal Year: 2023, Volume and Issue: 11, P. 54760 - 54772
Published: Jan. 1, 2023
Language: Английский
Citations
14Computers, Journal Year: 2024, Volume and Issue: 13(2), P. 36 - 36
Published: Jan. 28, 2024
In the past ten years, rates of forest fires around world have increased significantly. Forest greatly affect ecosystem by damaging vegetation. are caused several causes, including both human and natural causes. Human causes lie in intentional irregular burning operations. Global warming is a major cause fires. The early detection reduces rate their spread to larger areas speeding up extinguishing with help equipment materials for detection. this research, an system proposed called Defender Fusion. This achieved high accuracy long-term monitoring site using Intermediate Fusion VGG16 model Enhanced Consumed Energy-Leach protocol (ECP-LEACH). receives RGB (red, green, blue) IR (infrared) images from drones detect System provides regulation energy consumption achieves so that detected early. was trained on FLAME 2 dataset obtained 99.86%, superior rest models track input together. A simulation Python language demonstrate real time performed.
Language: Английский
Citations
5Scientific Reports, Journal Year: 2024, Volume and Issue: 14(1)
Published: June 14, 2024
Abstract Advances in computer image recognition have significantly impacted many industries, including healthcare, security and autonomous systems. This paper aims to explore the potential of improving algorithms enhance recognition. Specifically, we will focus on regression methods as a means improve accuracy efficiency identifying images. In this study, analyze various techniques their applications recognition, well resulting performance improvements through detailed examples data analysis. deals with problems related visual processing outdoor unstructured environment. Finally, heterogeneous patterns are converted into same pattern, extracted from fusion features modes. The simulation results show that perception ability complex environment improved.
Language: Английский
Citations
5Journal of Population Therapeutics and Clinical Pharmacology, Journal Year: 2022, Volume and Issue: 28(2)
Published: Jan. 1, 2022
Aim: This study aims at developing an automatic medical image analysis and detection for accurate classification of brain tumors from MRI dataset.The implemented our novel MIDNet18 CNN architecture in comparison with the VGG16 classifying normal images tumor images. Materials methods:The MIDNet-18 comprises 14 convolutional layers, 7 pooling 4 dense layers 1 layer.The dataset used this has two classes: Normal Brain MR Images Tumor Images.This binary consists 2918 as training set, 1458 validation set 212 test set.Independent sample size calculated was each group, keeping GPower 80%.Result: From experimental results, proposed model obtained 98.7% accuracy.Whereas, accuracy 50%.Hence, performance achieved is better than VGG16.Conclusion: The proved to be statistically significant p value <0.001 (Independent t-test) existing VGG16.Brain tumour magnetic resonance using a CNN-based e114
Language: Английский
Citations
22Diagnostics, Journal Year: 2022, Volume and Issue: 12(11), P. 2700 - 2700
Published: Nov. 5, 2022
Background: Hospitals face a significant problem meeting patients’ medical needs during epidemics, especially when the number of patients increases rapidly, as seen recent COVID-19 pandemic. This study designs treatment recommender system (RS) for efficient management human capital and resources such doctors, medicines, in hospitals. We hypothesize that deep learning framework, combined with search paradigms an image can make RS very efficient. Methodology: uses Convolutional neural network (CNN) model feature extraction images discovers most similar patients. The input queries from hospital database chest X-ray images. It similarity metric computation Results: methodology recommends associated to being admitted hospital. performance proposed is verified five different CNN models four measures. ResNet-50 Maxwell–Boltzmann found be proper framework recommendation mean average precision more than 0.90 threshold similarities range 0.7 0.9 highest cosine 0.95. Conclusions: Overall, proven tool peak period pandemics adopted clinical settings.
Language: Английский
Citations
20Sensors, Journal Year: 2024, Volume and Issue: 24(2), P. 549 - 549
Published: Jan. 15, 2024
In virtual reality, augmented or animation, the goal is to represent movement of deformable objects in real world as similar possible world. Therefore, this paper proposed a method automatically extract cloth stiffness values from video scenes, and then they are applied material properties for simulation. We propose use deep learning (DL) models tackle issue. The Transformer model, combination with pre-trained architectures like DenseNet121, ResNet50, VGG16, VGG19, stands leading choice classification tasks. Position-Based Dynamics (PBD) computational framework widely used computer graphics physics-based simulations entities, notably cloth. It provides an inherently stable efficient way replicate complex dynamic behaviors, such folding, stretching, collision interactions. Our model characterizes based on softness-to-stiffness labels accurately categorizes videos using labeling. dataset utilized research derived meticulously designed stiffness-oriented experimental assessment encompasses extensive 3840 videos, contributing multi-label dataset. results demonstrate that our achieves impressive average accuracy 99.50%. These accuracies significantly outperform alternative RNN, GRU, LSTM, Transformer.
Language: Английский
Citations
4