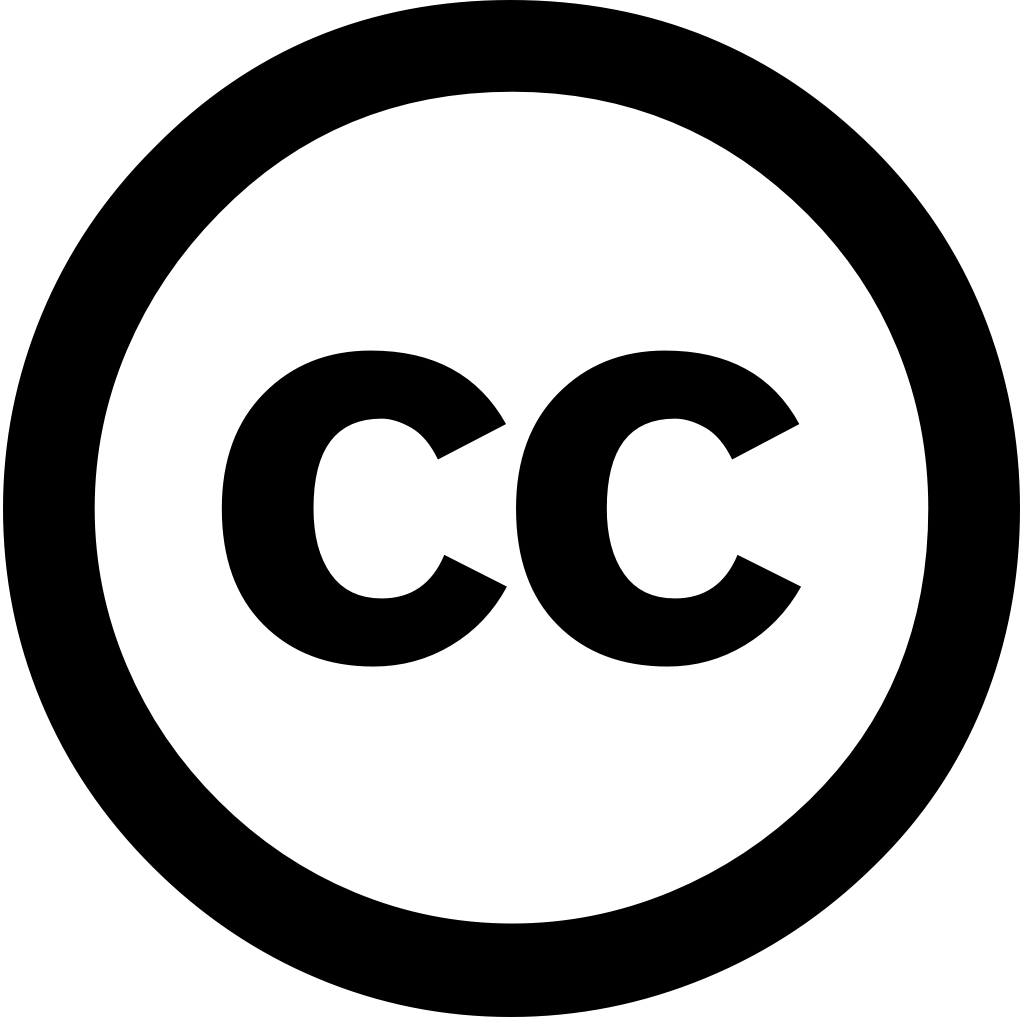
BMC Medical Informatics and Decision Making, Journal Year: 2024, Volume and Issue: 24(1)
Published: Oct. 4, 2024
Language: Английский
BMC Medical Informatics and Decision Making, Journal Year: 2024, Volume and Issue: 24(1)
Published: Oct. 4, 2024
Language: Английский
Computational and Structural Biotechnology Journal, Journal Year: 2022, Volume and Issue: 20, P. 4733 - 4745
Published: Jan. 1, 2022
Detection and Classification of a brain tumor is an important step to better understanding its mechanism. Magnetic Reasoning Imaging (MRI) experimental medical imaging technique that helps the radiologist find region. However, it time taking process requires expertise test MRI images, manually. Nowadays, advancement Computer-assisted Diagnosis (CAD), machine learning, deep learning in specific allow more reliably identify tumors. The traditional methods used tackle this problem require handcrafted feature for classification purposes. Whereas can be designed way not any extraction while achieving accurate results. This paper proposes two models both binary (normal abnormal) multiclass (meningioma, glioma, pituitary) We use publicly available datasets include 3064 152 respectively. To build our models, we first apply 23-layers convolution neural network (CNN) dataset since there large number images training purpose. when dealing with limited volumes data, which case second dataset, proposed "23-layers CNN" architecture faces overfitting problem. address issue, transfer combine VGG16 along reflection "23 layers architecture. Finally, compare those reported literature. Our results indicate achieve up 97.8% 100% accuracy employed datasets, respectively, exceeding all other state-of-the-art models. source codes are at: (https://github.com/saikat15010/Brain-Tumor-Detection).
Language: Английский
Citations
174Frontiers in Computational Neuroscience, Journal Year: 2022, Volume and Issue: 16
Published: Sept. 2, 2022
With the quick evolution of medical technology, era big data in medicine is quickly approaching. The analysis and mining these significantly influence prediction, monitoring, diagnosis, treatment tumor disorders. Since it has a wide range traits, low survival rate, an aggressive nature, brain regarded as deadliest most devastating disease. Misdiagnosed tumors lead to inadequate treatment, reducing patient's life chances. Brain detection highly challenging due capacity distinguish between aberrant normal tissues. Effective therapy long-term are made possible for patient by correct diagnosis. Despite extensive research, there still certain limitations detecting because unusual distribution pattern lesions. Finding region with small number lesions can be difficult areas tend look healthy. It directly reduces classification accuracy, extracting choosing informative features challenging. A significant role played automatically classifying early-stage utilizing deep machine learning approaches. This paper proposes hybrid model Convolutional Neural Network-Long Short Term Memory (CNN-LSTM) predicting through Magnetic Resonance Images (MRI). We experiment on MRI image dataset. First, preprocessed efficiently, then, Network (CNN) applied extract from images. proposed predicts accuracy 99.1%, precision 98.8%, recall 98.9%, F1-measure 99.0%.
Language: Английский
Citations
63IEEE Access, Journal Year: 2022, Volume and Issue: 10, P. 112117 - 112133
Published: Jan. 1, 2022
The convolutional neural networks (CNN) have shown promising results for various classification problems over the past years. However, selecting CNN architectures is still challenging as each architecture performs differently with same dataset. This research aims to evaluate dependence of brain MRI on predictive models based complexity data Brain Tumor and Alzheimer's Disease. Our proposed approach has three parts. First part pre-processing which mainly focuses class balancing estimation complexity. second uses stratified k-fold cross-validation more reliable results. last corresponds implementation four applying described methods. paper compares performance rigorous experimentation variants namely S-CNN (CNN trained from scratch), ResNet50, InceptionV3, Xception two image datasets evaluated without use Principal Component Analysis (PCA). work benchmarks by comparing average scores Accuracy, Precision, Recall, F1 score, AUC score five-fold cross-validation.
Language: Английский
Citations
492022 6th International Conference on Electronics, Communication and Aerospace Technology, Journal Year: 2022, Volume and Issue: unknown
Published: Dec. 1, 2022
In both grown-ups and juvenile, brain tumors are the tenth most predominant cause of death rate. There many different sorts tumors, each one has extremely slim odds survival based on texture, location, shape. The worst outcomes can result from incorrect categorization. quantity CNN's hidden neurons convolutional layers is tuned in suggested deep learning classification. effective classifier that a high rank. Since artificial intelligence techniques have become more prevalent, it now possible to detect cancers by using machine algorithms. order create an intensive perception, this work extricates various features models bolsters them as input Adadelta SGD optimizer. incredible execution capability proposed model realised utilizing
Language: Английский
Citations
40Soft Computing, Journal Year: 2023, Volume and Issue: 27(11), P. 7535 - 7542
Published: Feb. 14, 2023
Language: Английский
Citations
29Journal of Healthcare Engineering, Journal Year: 2023, Volume and Issue: 2023(1)
Published: Jan. 1, 2023
Over the past few years, a tremendous change has occurred in computer-aided diagnosis (CAD) technology. The evolution of numerous medical imaging techniques enhanced accuracy preliminary analysis several diseases. Magnetic resonance (MRI) is prevalent technology extensively used evaluating progress spread malignant tissues or abnormalities human body. This article aims to automate computationally efficient mechanism that can accurately identify tumor from MRI images and analyze impact tumor. proposed model robust enough classify tumors with minimal training data. generative variational autoencoder models are reconstructing identical original images, which adequately model. self-learning algorithm learn insights autogenerated images. Incorporating long short-term memory (LSTM) faster processing high dimensional data, making radiologist's task practitioners more comfortable assessing tumor's progress. Self-learning need comparatively less data for training, resource than various state-of-art models. efficiency been assessed using benchmark metrics, obtained results have exhibited an 89.7%. growth presented current study. not pleasing healthcare domain, yet reasonably fair dealing smaller size dataset by use image generation mechanism. study would outline role Future technologies may include sturdy feature engineering optimized activation functions yield better result.
Language: Английский
Citations
27Biomedical Signal Processing and Control, Journal Year: 2024, Volume and Issue: 91, P. 106093 - 106093
Published: Feb. 7, 2024
Language: Английский
Citations
14International journal of intelligent engineering and systems, Journal Year: 2024, Volume and Issue: 17(2), P. 632 - 644
Published: Feb. 28, 2024
In the rapidly evolving landscape of medical imaging, our proposed work presents an innovative and efficient approach to brain tumor detection through advanced deep learning methodologies.Central methodology is strategic utilization pre-trained weights from formidable MBConv-Finetuned-B0 model, initially honed on expansive ImageNet dataset, providing a foundation rich in general visual knowledge.Our subsequent fine-tuning process targets specific layers relevant detection, introducing two distinct convolutional layers, MBConv 6, 55, 30, meticulously added base model.These are intricately designed extract refine features tumors, ensuring nuanced understanding pathology enhancing model's discrimination accuracy.The flexibility exemplified by thoughtful consideration options: one that adjusts all model another selectively fine-tunes only layers.We conduct detailed comparative analysis, including homogeneity median feature values, placing direct comparison with established techniques such as Ensemble Transfer Learning Quantum Variational Classifier (ETL & QVC), Ultra-Light Deep (ULDL) Model, Convolutional Neural Network (DCNN), Image Processing (DLIP).The results showcase proficiency, achieving accuracy 94%, precision 84%, recall 92%, F1 score 88%, AUC-ROC 96%.Notably, demonstrates superior performance terms (vE Homogeneity: 0.93, vN 0.91, Enhancement 0.97) values (Median vE Feature Value: 0.82, Median 0.87, 0.80), comprehensive its effectiveness capturing subtle nuances images.
Language: Английский
Citations
13Scientific Reports, Journal Year: 2024, Volume and Issue: 14(1)
Published: April 25, 2024
Abstract The use of various kinds magnetic resonance imaging (MRI) techniques for examining brain tissue has increased significantly in recent years, and manual investigation each the resulting images can be a time-consuming task. This paper presents an automatic brain-tumor diagnosis system that uses CNN detection, classification, segmentation glioblastomas; latter stage seeks to segment tumors inside glioma MRI images. structure developed multi-unit consists two stages. first is responsible tumor detection classification by categorizing into normal, high-grade (glioblastoma), low-grade glioma. uniqueness proposed network lies its different levels features, including local global paths. second segmentation, skip connections residual units are used during this step. Using 1800 extracted from BraTS 2017 dataset, was found achieve maximum accuracy 99%. then evaluated using Dice score, specificity, sensitivity. results showed suggested deep-learning-based ranks highest among variety strategies reported literature.
Language: Английский
Citations
13IEEE Access, Journal Year: 2024, Volume and Issue: 12, P. 40204 - 40212
Published: Jan. 1, 2024
Continuous advancements in deep learning are affecting various research areas, especially on applications the medical sector. A computer-aided diagnosis system that utilizes is used for classifying and detecting brain tumors magnetic resonance imaging. Regarding tumors, main diagnostic indicators patient symptoms outcomes of Frequent changes these have raised serious concerns about potential misdiagnoses. Implementing systems can support methods rely visual assessments physicians, potentially reducing misdiagnosis rates. In this study, we propose an enhanced algorithm optimized tumor classification. We removed noise from imaging results by applying Gaussian filters, employed GridMask to improve generalization performances models. Then, applied Patterned-GridMask, which a method proposed reduce issue being obscured standard GridMask. Under application performance improvement up 6% was demonstrated across four models experiments: ViT-B/16, MaxViT-B, TresNet-M, EfficientNetV2-M, with highest represented accuracy F1-score 97.74% 97.75%, respectively. Using system, improved be obtained, thereby resulting more accurate rates early detection, better outcomes, appropriate treatment selection.
Language: Английский
Citations
11