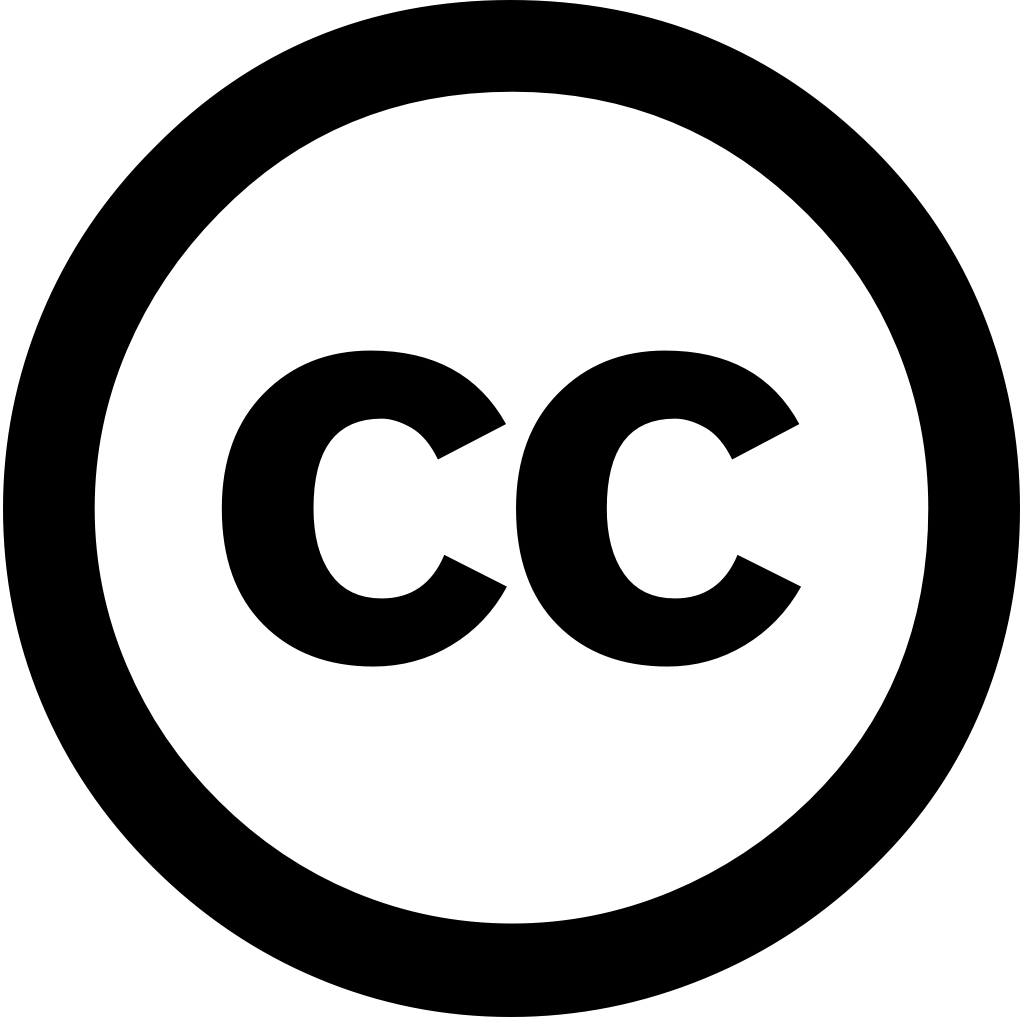
Mathematical Biosciences & Engineering, Journal Year: 2024, Volume and Issue: 21(7), P. 6631 - 6657
Published: Jan. 1, 2024
Facial emotion recognition (FER) is largely utilized to analyze human in order address the needs of many real-time applications such as computer-human interfaces, detection, forensics, biometrics, and human-robot collaboration. Nonetheless, existing methods are mostly unable offer correct predictions with a minimum error rate. In this paper, an innovative facial framework, termed extended walrus-based deep learning Botox feature selection network (EWDL-BFSN), was designed accurately detect emotions. The main goals EWDL-BFSN identify emotions automatically effectively by choosing optimal features adjusting hyperparameters classifier. gradient wavelet anisotropic filter (GWAF) can be used for image pre-processing model. Additionally, SqueezeNet extract significant features. improved optimization algorithm (IBoA) then choose best Lastly, FER classification accomplished through use enhanced optimization-based kernel residual 50 (EK-ResNet50) network. Meanwhile, nature-inspired metaheuristic, walrus (WOA) pick EK-ResNet50 model trained tested publicly available CK+ FER-2013 datasets. Python platform applied implementation, various performance metrics accuracy, sensitivity, specificity, F1-score were analyzed state-of-the-art methods. proposed acquired overall accuracy 99.37 99.25% both datasets proved its superiority predicting over
Language: Английский