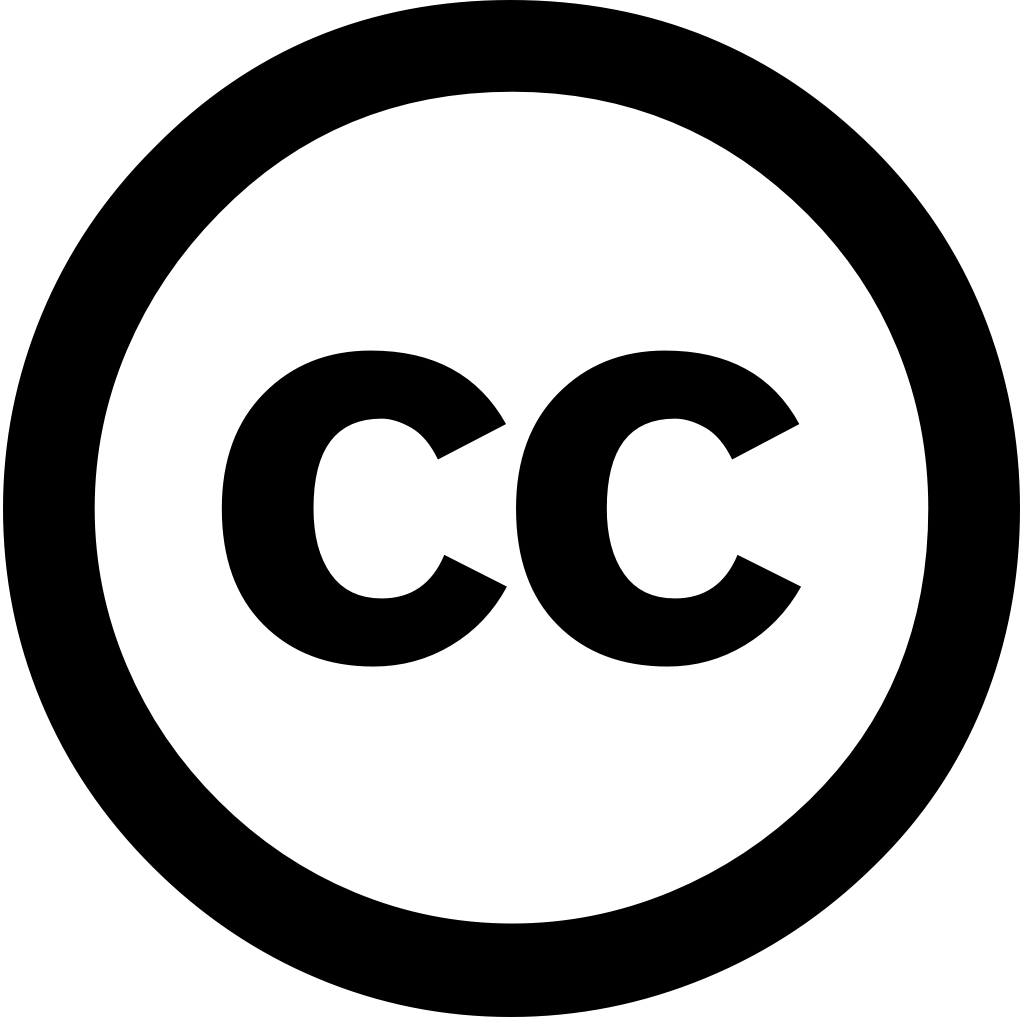
Heliyon, Journal Year: 2024, Volume and Issue: 10(14), P. e34143 - e34143
Published: July 1, 2024
Language: Английский
Heliyon, Journal Year: 2024, Volume and Issue: 10(14), P. e34143 - e34143
Published: July 1, 2024
Language: Английский
Electric Power Systems Research, Journal Year: 2025, Volume and Issue: 244, P. 111584 - 111584
Published: March 3, 2025
Language: Английский
Citations
0Frontiers in Marine Science, Journal Year: 2025, Volume and Issue: 12
Published: May 8, 2025
Introduction Salt spray formation and migration in hot humid marine environments have a significant impact on engineering equipment maintenance. Accurately predicting these phenomena is crucial for reducing corrosion damage. Traditional research methodologies primarily utilize statistical models or physics-based simulations. Although approaches yield satisfactory results within controlled conditions, they often encounter limitations accurately capturing the complexity variability inherent to environments. These methods struggle capture spatiotemporal dependencies of salt migration. Moreover, are typically difficult apply real-time lack ability handle large-scale, dynamic data. Methods This study aims address this issue by proposing OceanLSTM model, which combines temporal modeling capabilities xLSTM with spatial attention mechanism relationships between complex environmental variables, thereby improving accuracy predictions. Results The experiments used several representative environment datasets, including NOAA Marine Aerosol datasets. experimental demonstrate that significantly outperforms traditional evaluation metrics such as F1-score, especially datasets strong dependencies. Discussion provides more precise efficient tool future monitoring prediction, offering important practical applications.
Language: Английский
Citations
0Sensors, Journal Year: 2024, Volume and Issue: 24(17), P. 5589 - 5589
Published: Aug. 29, 2024
Combining medical IoT and artificial intelligence technology is an effective approach to achieve the of equipment. This integration can address issues such as low image quality caused by fluctuations in power potential equipment damage, this study proposes a predictive model, ISSA-TCN-BiLSTM, based on bi-directional long short-term memory network (BiLSTM). Firstly, data other from MRI CT within 6-month period are collected using current fingerprint technology. The key factors affecting active explored Pearson coefficient method. Subsequently, Temporal Convolutional Network (TCN) employed conduct multi-layer convolution operations input temporal feature sequences, enabling learning global information while minimizing interference redundant data. Additionally, bidirectional (BiLSTM) integrated model intermediate features, facilitating accurate prediction quality. improved Sparrow Search Algorithm (ISSA) utilized for hyperparameter optimization TCN-BiLSTM different Experimental results demonstrate that ISSA-TCN-BiLSTM outperforms comparative models terms RMSE, MSE, R2, with values 0.1143, 0.1157, 0.0873, 0.0817, 0.95, 0.96, respectively, exhibits both speed accuracy equipment, providing valuable guidance maintenance diagnostic efficiency enhancement.
Language: Английский
Citations
1Heliyon, Journal Year: 2024, Volume and Issue: 10(14), P. e34143 - e34143
Published: July 1, 2024
Language: Английский
Citations
0