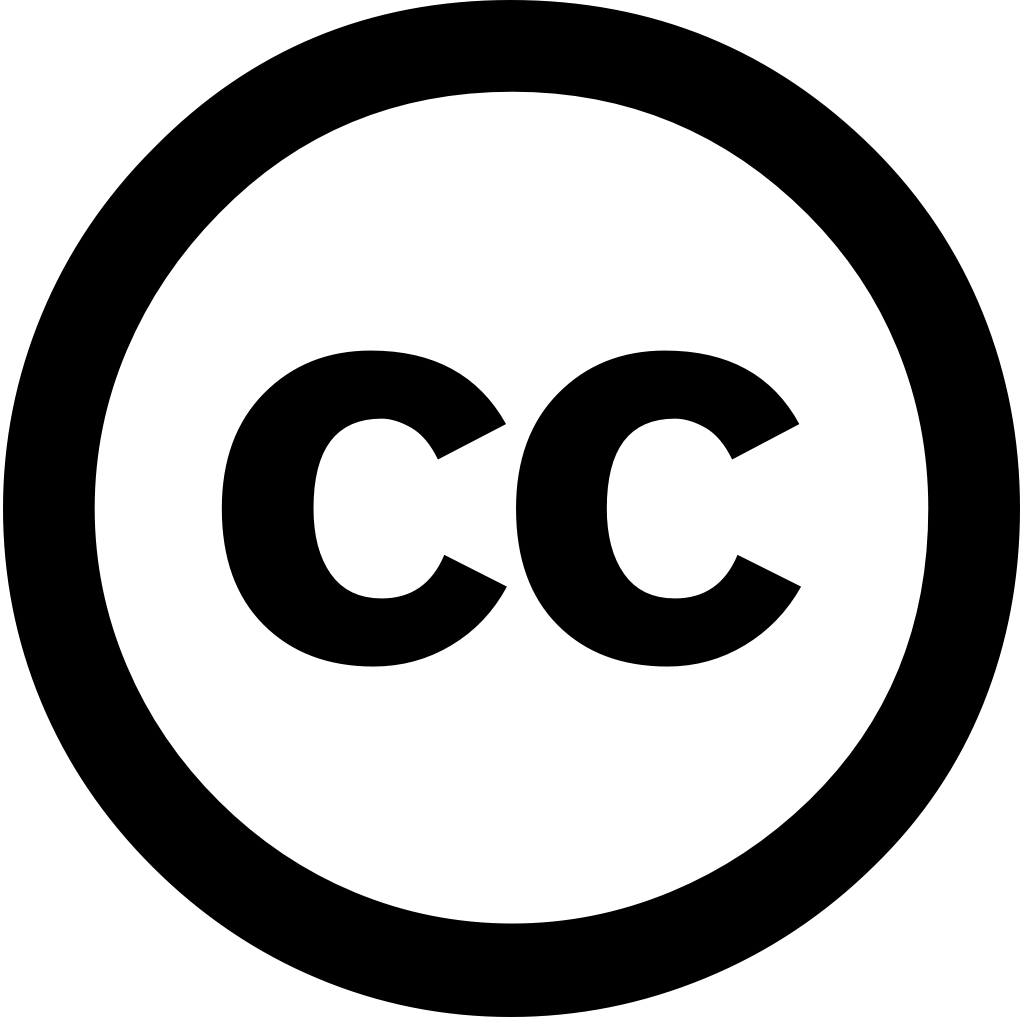
Mathematics, Journal Year: 2024, Volume and Issue: 12(23), P. 3794 - 3794
Published: Nov. 30, 2024
The rapid growth of e-commerce has transformed customer behaviors, demanding deeper insights into how demographic factors shape online user preferences. This study performed a threefold analysis to understand the impact these changes. Firstly, this investigated (e.g., age, gender, education) influence e-customer preferences in Serbia. From sample n = 906 respondents, conditional dependencies between demographics and were tested. hypothetical framework 24 tested hypotheses, successfully rejected 8/24 (with p < 0.05), suggesting high association with purchase frequency reasons for quitting purchase. However, although reported test statistics suggested an association, understanding interactions categories profiles was still required. Therefore, second part considers MCA-HCPC (Multiple Correspondence Analysis Hierarchical Clustering on Principal Components) identify profiles. revealed three main clusters: (1) young, female, unemployed e-customers driven mainly by reviews; (2) retirees older adults infrequent purchases, hesitant buy without experiencing product person; (3) employed, highly educated, male, middle-aged who prioritize fast accurate delivery over price. In third stage, clusters are used as labels Machine Learning (ML) classification tasks. Particularly, Gradient Boosting (GBM), Decision Tree (DT), k-Nearest Neighbors (kNN), Gaussian Naïve Bayes (GNB), Random Forest (RF), Support Vector (SVM) used. results that GBM, RF, SVM had performance identifying Lastly, after performing Permutation Feature Importance (PFI), findings work status, education, income determinants shaping developing marketing strategies.
Language: Английский