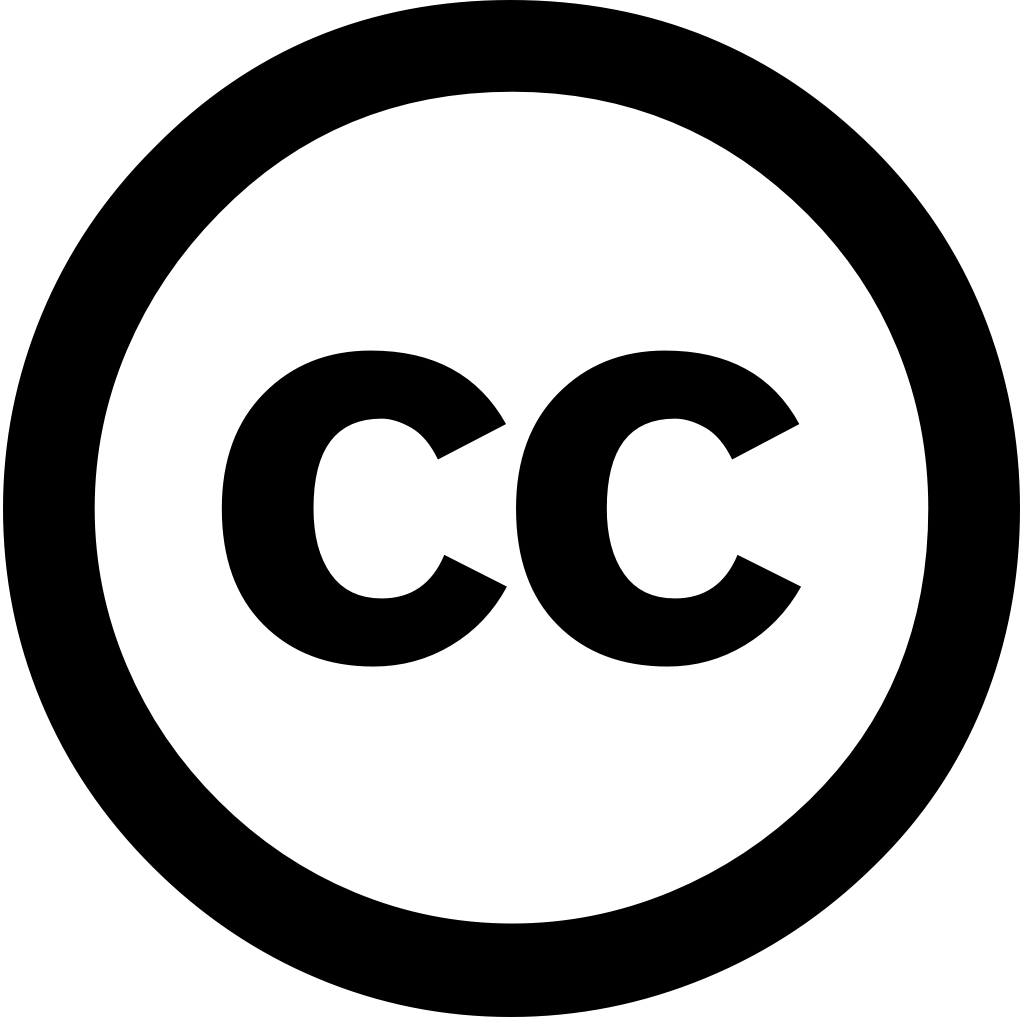
Agriculture, Journal Year: 2025, Volume and Issue: 15(7), P. 789 - 789
Published: April 6, 2025
Efficient pest control in orchards is crucial for preserving crop quality and maximizing yield. A key factor optimizing automated variable-rate spraying accurate tree canopy size estimation, which helps reduce pesticide overuse while minimizing environmental health risks. This study evaluates the performance of two advanced convolutional neural networks, PP-LiteSeg fully networks (FCNs), segmenting canopies varying sizes—small, medium, large—using short-term dense-connection (STDC1 STDC2) as backbones. dataset 305 field-collected images was used model training evaluation. The results show that FCNs with STDC backbones outperform PP-LiteSeg, delivering superior semantic segmentation accuracy background classification. STDC1-based excels precision spraying, achieving an Intersection-over-Union up to 0.75, Recall 0.85, Precision approximately 0.85. Meanwhile, STDC2-based demonstrates greater optimization stability faster convergence, making it more suitable resource-constrained environments. Notably, significantly enhances canopy-background differentiation, a classification 0.9942. In contrast, struggles small detection, leading reduced accuracy. These findings highlight potential apple recognition, advancing agriculture promoting sustainable application through improved strategies.
Language: Английский