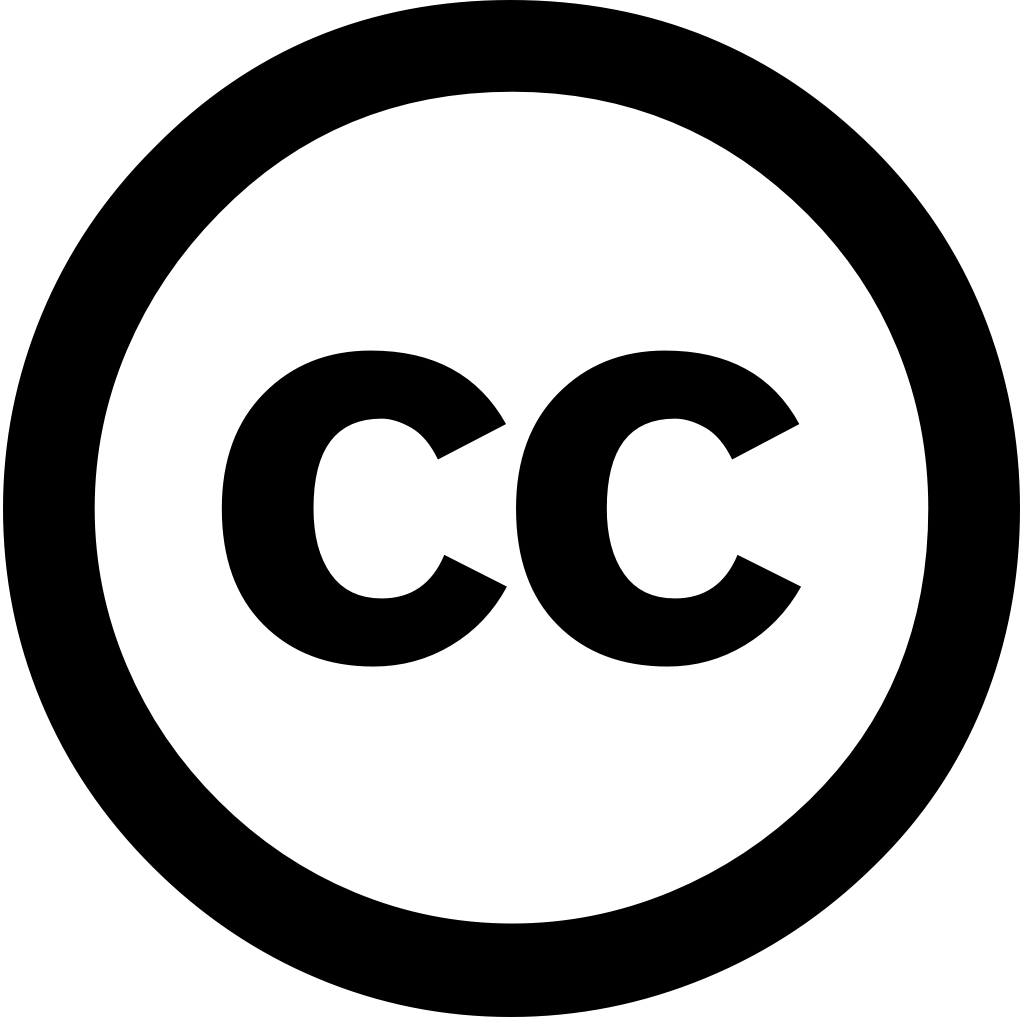
Applied Sciences, Journal Year: 2025, Volume and Issue: 15(6), P. 3077 - 3077
Published: March 12, 2025
Skin cancer is one of the most common and life-threatening diseases. In current era, early detection remains a significant challenge, particularly in remote underserved regions with limited internet access. Traditional skin systems often depend on image classification using deep learning models that require constant connectivity to access, creating barriers areas poor infrastructure. To address this limitation, CNN provides an innovative solution by enabling on-device machine low-computing Internet Things (IoT) devices. This study evaluates performance convolutional neural network (CNN) model trained 10,000 dermoscopic images spanning seven classes from Harvard Lesion dataset. Unlike previous research, which seldom offers detailed evaluations IoT hardware, work benchmarks multiple single-board computers (SBCs), including devices like Raspberry Pi Jetson Nano. The evaluation focuses accuracy hardware efficiency, analyzing impact varying training dataset sizes assess model’s scalability effectiveness resource-constrained simulation results demonstrate feasibility deploying accurate efficient directly low-power hardware. show our proposed method achieves 98.25%, fastest being 5, time 0.01 s.
Language: Английский