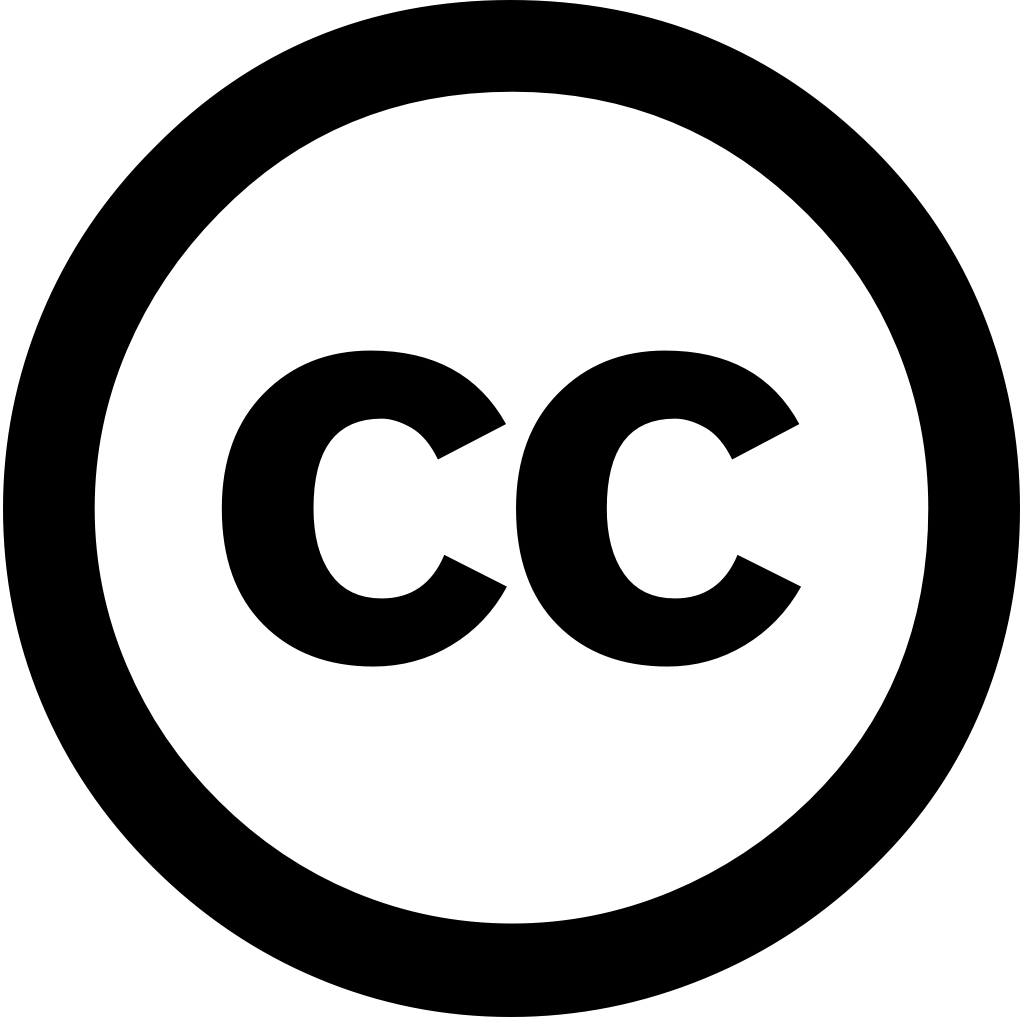
Applied Sciences, Journal Year: 2025, Volume and Issue: 15(7), P. 4037 - 4037
Published: April 7, 2025
The increasing adoption of blockchain technology has presented significant challenges in maintaining the security and reliability smart contracts. This study addresses problem identifying flaws contracts, which may result monetary damages diminished confidence systems. A Hybrid Quantum–Classical Deep Neural Network (HQCDNN) approach was proposed, combining quantum computing principles with classical deep learning methods to identify various vulnerability types, including access control, arithmetic, front-running, reentrancy, time manipulation, denial service, unchecked low calls. SmartBugs Wild Dataset used for training, TF-IDF employed as a preprocessing technique optimized hybrid architectures. Experiments were conducted using architectures 2-qubit 4-qubit layers, alongside neural network (DNN) model comparative analysis. HQCDNN attained accuracy levels ranging from 96.4% 78.2% F1-scores between 96.6% 80.2%, showcasing enhanced performance compared models referenced literature. These results highlight capability HQCDNNs improve identification Future work could focus on evaluating actual devices expanding its application larger datasets further validation.
Language: Английский