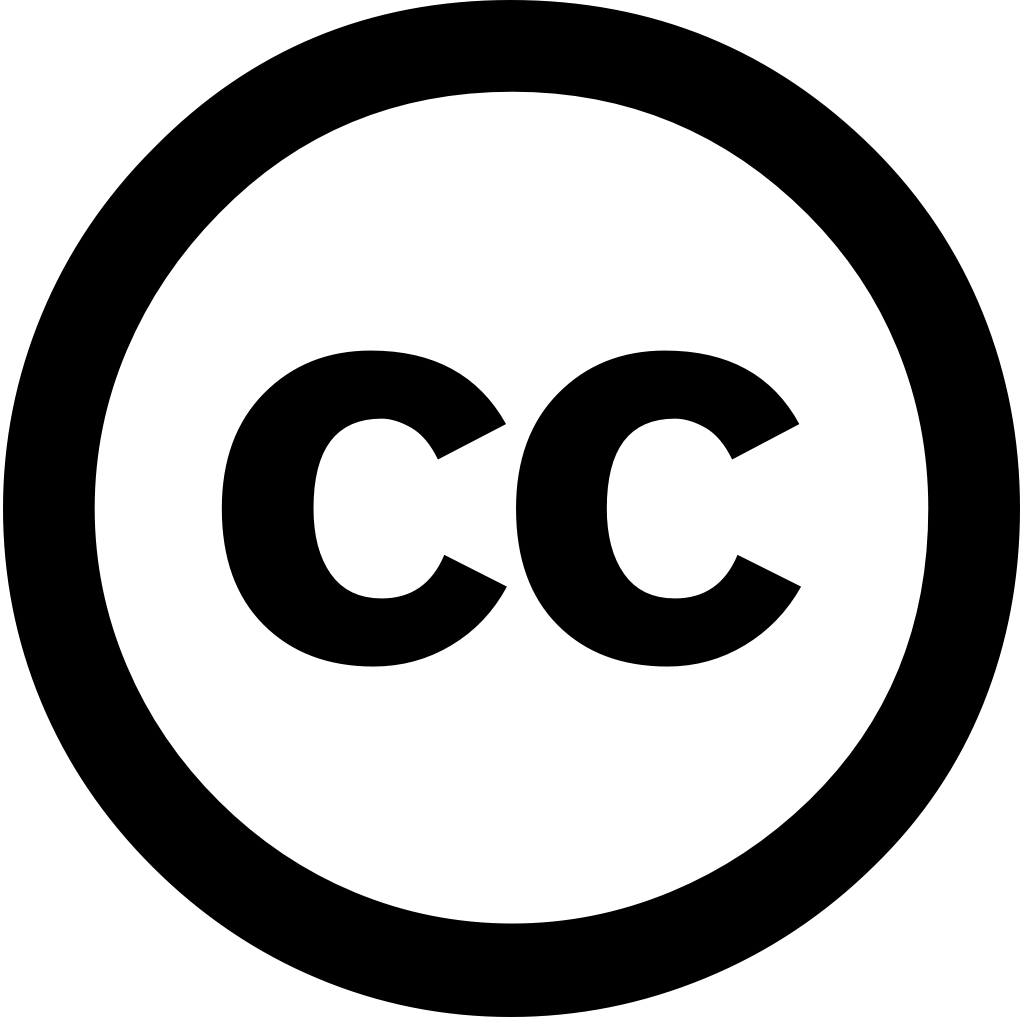
Applied Sciences, Journal Year: 2025, Volume and Issue: 15(4), P. 1818 - 1818
Published: Feb. 10, 2025
The dense text detection and segmentation of Chinese characters has always been a research hotspot due to the complex background diverse scenarios. In field education, handwritten is affected by noise, texture interference, etc. Especially in low-quality text, character overlap or occlusion makes boundaries blurred, which increases difficulty segmentation; this paper, an improved EAST network CEE (Components-ECA-EAST Network), fuses attention mechanism with feature pyramid structure, proposed based on analysis structure mini-components. ECA (Efficient Channel Attention) incorporated during extraction phase; fusion stage, convolutional features are extracted from self-constructed mini-component dataset then fused cascade manner, finally, Dice Loss used as regression task loss function. above improvements comprehensively improve performance detecting segmenting mini-components subtle strokes characters; model was tested accuracy 84.6% mAP 77.6%, improvement 7.4% 8.4%, respectively, compared original model; constructed well suited for applications such writing grade examinations, represent important exploration development educational intelligence.
Language: Английский