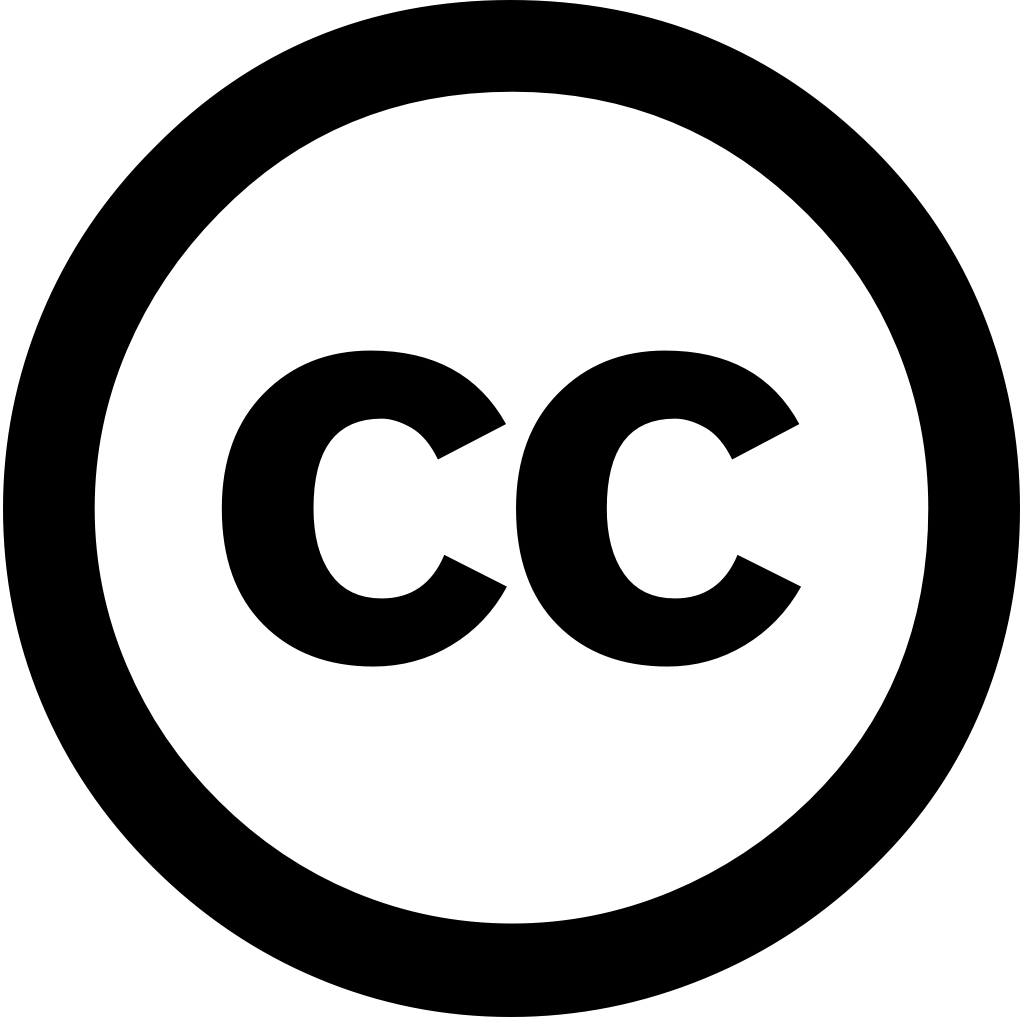
Foods, Journal Year: 2025, Volume and Issue: 14(10), P. 1680 - 1680
Published: May 9, 2025
To address the problem of detecting foreign bodies in Pu-erh tea, this study proposes an intelligent detection method based on improved YOLOv10 network. By introducing MPDIoU loss function, network is optimized to effectively enhance positioning accuracy model complex background and improve small target objects. Using AssemFormer optimize structure, network’s ability perceive objects its process global information are improved. Rectangular Self-Calibrated Module, prediction bounding box optimized, further improving classification target-positioning abilities scenes. The results showed that Box, Cls, Dfl functions CV-YOLOv10-AR-M One-to-Many Head task were, respectively, 14.60%, 19.74%, 20.15% lower than those In One-to-One task, they decreased by 10.42%, 29.11%, 20.15%, respectively. Compared with original network, accuracy, recall rate, mAP were increased 5.35%, 11.72% 8.32%, improves model’s attention sizes, backgrounds, detailed information, providing effective technical support for quality control agricultural field.
Language: Английский