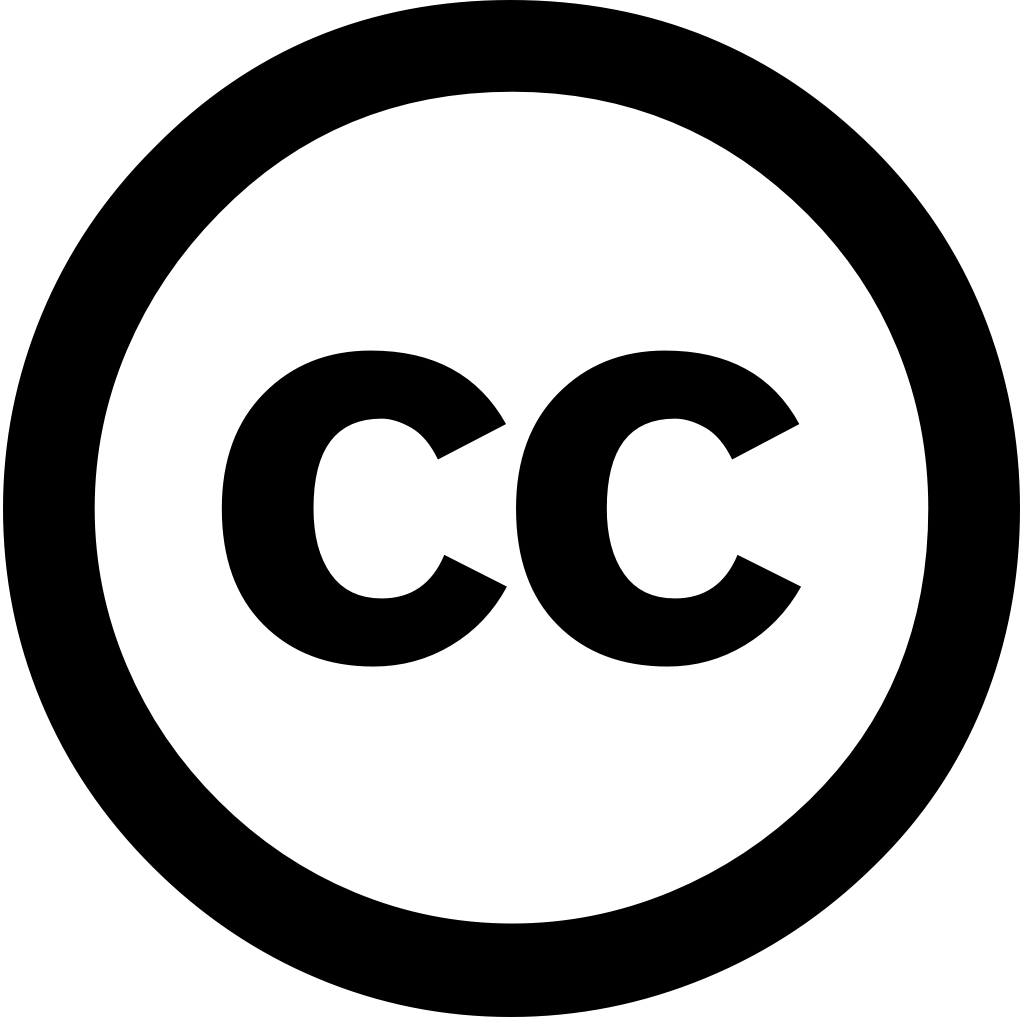
Ecological Informatics, Journal Year: 2024, Volume and Issue: unknown, P. 102889 - 102889
Published: Nov. 1, 2024
Language: Английский
Ecological Informatics, Journal Year: 2024, Volume and Issue: unknown, P. 102889 - 102889
Published: Nov. 1, 2024
Language: Английский
Ecological Informatics, Journal Year: 2025, Volume and Issue: unknown, P. 102995 - 102995
Published: Jan. 1, 2025
Language: Английский
Citations
3Remote Sensing, Journal Year: 2024, Volume and Issue: 16(9), P. 1564 - 1564
Published: April 28, 2024
Flash droughts tend to cause severe damage agriculture due their characteristics of sudden onset and rapid intensification. Early detection the response vegetation flash is utmost importance in mitigating effects droughts, as it can provide a scientific basis for establishing an early warning system. The commonly used method determining time drought, based on index or correlation between precipitation anomaly growth anomaly, leads late irreversible drought vegetation, which may not be sufficient use analyzing earning. evapotranspiration-based (ET-based) indices are effective indicator identifying monitoring drought. This study proposes novel approach that applies cross-spectral analysis ET-based index, i.e., Evaporative Stress Anomaly Index (ESAI), forcing vegetation-based Normalized Vegetation (NVAI), response, both from medium-resolution remote sensing data, estimate lag vitality status An experiment was carried out North China during March–September period 2001–2020 using products at 1 km spatial resolution. results show average water availability estimated by over 5.9 days, shorter than measured widely (26.5 days). main difference phase lies fundamental processes behind definitions two methods, subtle dynamic fluctuation signature signal (vegetation-based index) correlates with (ET-based versus impact indicated negative NDVI anomaly. varied types irrigation conditions. rainfed cropland, irrigated grassland, forest 5.4, 5.8, 6.1, 6.9 respectively. Forests have longer grasses crops deeper root systems, mitigate impacts droughts. Our method, innovative earlier impending impacts, rather waiting occur. information detected stage help decision makers developing more timely strategies ecosystems.
Language: Английский
Citations
5Neural Computing and Applications, Journal Year: 2025, Volume and Issue: unknown
Published: Feb. 15, 2025
Language: Английский
Citations
0Weather and Climate Extremes, Journal Year: 2025, Volume and Issue: unknown, P. 100762 - 100762
Published: March 1, 2025
Language: Английский
Citations
0Climate, Journal Year: 2024, Volume and Issue: 12(8), P. 119 - 119
Published: Aug. 10, 2024
This study addresses the critical issue of drought zoning in Canada using advanced deep learning techniques. Drought, exacerbated by climate change, significantly affects ecosystems, agriculture, and water resources. Canadian Drought Monitor (CDM) data provided government ERA5-Land daily were utilized to generate a comprehensive time series mean monthly precipitation air temperature for 199 sample locations from 1979 2023. These processed Google Earth Engine (GEE) environment used develop Convolutional Neural Network (CNN) model estimate CDM values, thereby filling gaps historical data. The CanESM5 model, as assessed IPCC Sixth Assessment Report, was employed under four change scenarios predict future conditions. Our CNN forecasts values up 2100, enabling accurate zoning. results reveal significant trends changes, indicating areas most vulnerable droughts, while shows slow increasing trend. analysis indicates that extreme scenarios, certain regions may experience increase frequency severity necessitating proactive planning mitigation strategies. findings are policymakers stakeholders designing effective management adaptation programs.
Language: Английский
Citations
2Ecological Informatics, Journal Year: 2024, Volume and Issue: unknown, P. 102889 - 102889
Published: Nov. 1, 2024
Language: Английский
Citations
0