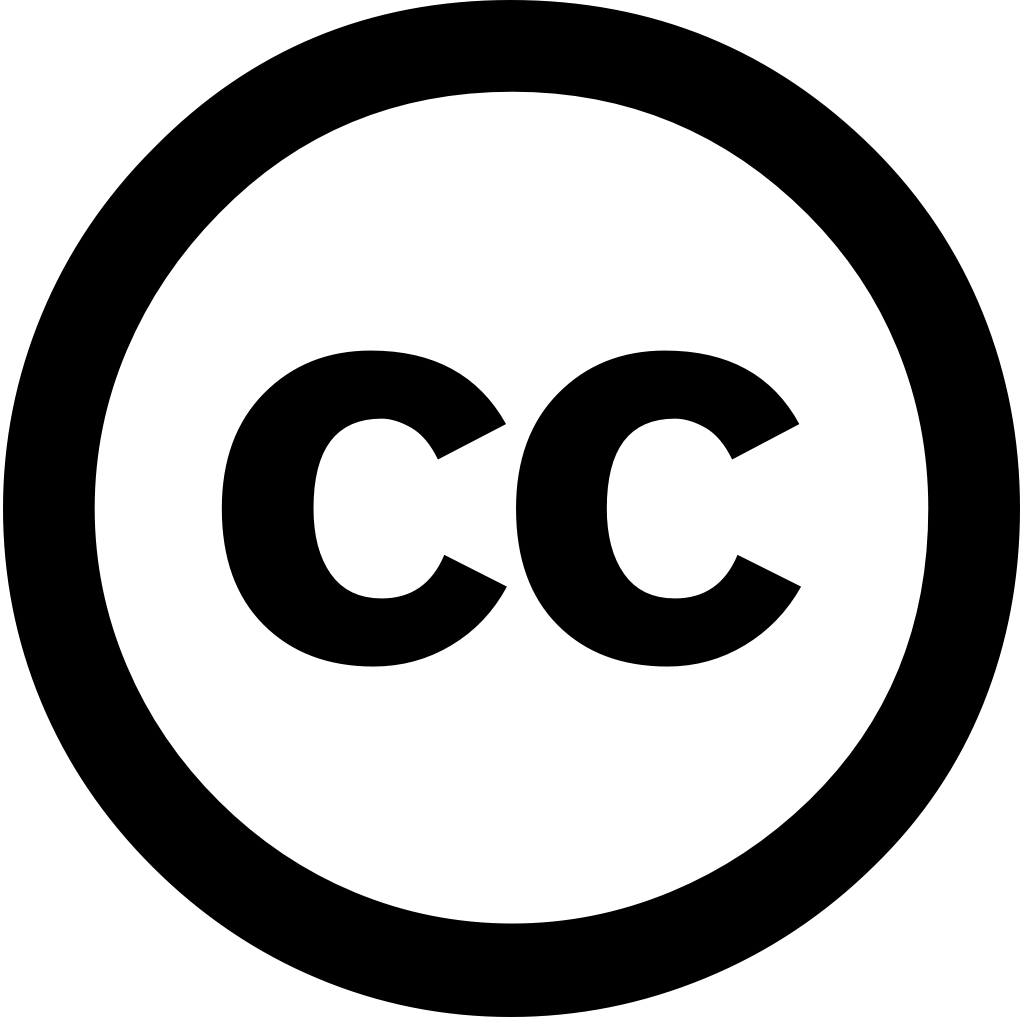
Atmospheric chemistry and physics, Journal Year: 2025, Volume and Issue: 25(7), P. 3919 - 3928
Published: April 8, 2025
Abstract. Aerosol acidity (or pH) plays a crucial role in atmospheric chemistry, influencing the interaction of air pollutants with ecosystems and climate. pH shows large temporal variations, while driving factors chemical profiles versus meteorological conditions are not fully understood due to their intrinsic complexity. Here, we propose new framework quantify factor importance, which incorporated an interpretive structural modeling (ISM) approach time series analysis. In particular, hierarchical relationship is established based on multiphase buffer theory ISM. A long-term (2018–2023) observation dataset Changzhou, China, analyzed this framework. We found variation dominated by seasonal random trend varies little despite emission changes. This overall effect decreasing PM2.5, increasing temperature increased alkali-to-acid ratios. Temperature controlling through effective acid dissociation constant Ka∗, non-ideality cni gas–particle partitioning. Random variations aerosol water contents Ka∗ cni. provides quantitative understanding at different levels, important acidity-related process studies policy-making.
Language: Английский