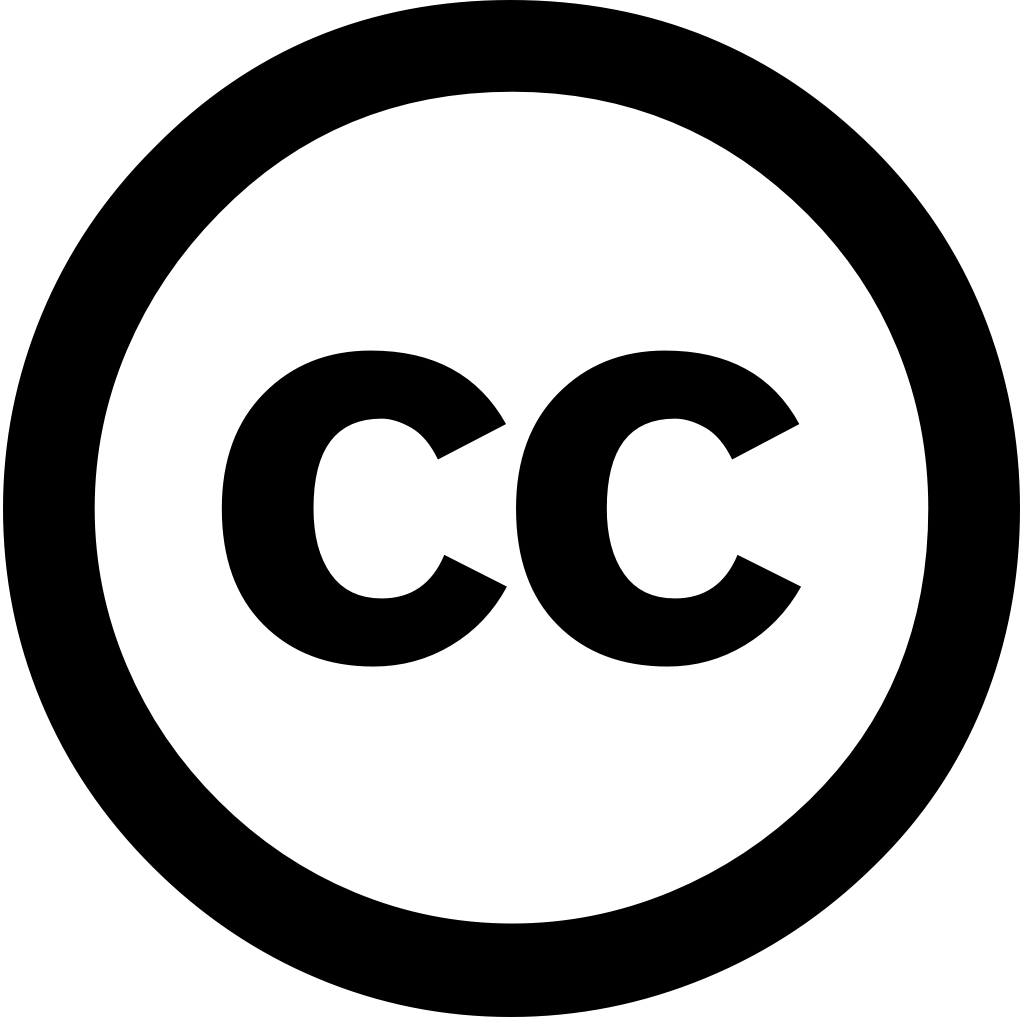
Toxics, Journal Year: 2025, Volume and Issue: 13(4), P. 254 - 254
Published: March 28, 2025
Surface air pollution affects ecosystems and people’s health. However, traditional models have low prediction accuracy. Therefore, a hybrid model for accurately predicting daily surface PM2.5 concentrations was integrated with wavelet (W), convolutional neural network (CNN), bidirectional long short-term memory (BiLSTM), gated recurrent unit (BiGRU). The data meteorological factors pollutants in Guangzhou City from 2014 to 2020 were utilized as inputs the models. W-CNN-BiGRU-BiLSTM demonstrated strong performance during phase, achieving an R (correlation coefficient) of 0.9952, root mean square error (RMSE) 1.4935 μg/m3, absolute (MAE) 1.2091 percentage (MAPE) 7.3782%. Correspondingly, accurate is beneficial control urban planning.
Language: Английский