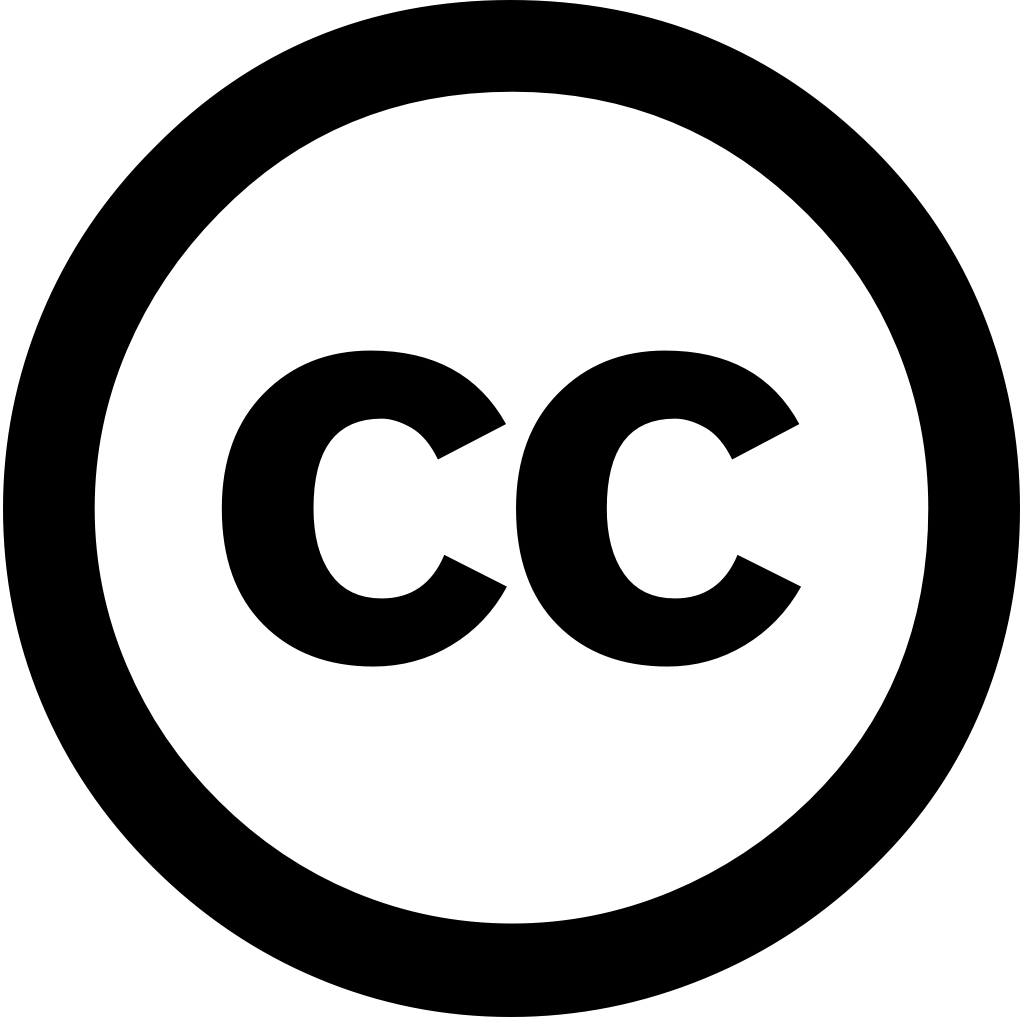
Journal of Infection and Public Health, Journal Year: 2024, Volume and Issue: 18(2), P. 102627 - 102627
Published: Dec. 18, 2024
Language: Английский
Journal of Infection and Public Health, Journal Year: 2024, Volume and Issue: 18(2), P. 102627 - 102627
Published: Dec. 18, 2024
Language: Английский
Asia-Pacific Journal of Ophthalmology, Journal Year: 2024, Volume and Issue: 13(4), P. 100085 - 100085
Published: July 1, 2024
Large language models (LLMs), a natural processing technology based on deep learning, are currently in the spotlight. These closely mimic comprehension and generation. Their evolution has undergone several waves of innovation similar to convolutional neural networks. The transformer architecture advancement generative artificial intelligence marks monumental leap beyond early-stage pattern recognition via supervised learning. With expansion parameters training data (terabytes), LLMs unveil remarkable human interactivity, encompassing capabilities such as memory retention comprehension. advances make particularly well-suited for roles healthcare communication between medical practitioners patients. In this comprehensive review, we discuss trajectory their potential implications clinicians For clinicians, can be used automated documentation, given better inputs extensive validation, may able autonomously diagnose treat future. patient care, triage suggestions, summarization documents, explanation patient's condition, customizing education materials tailored level. limitations possible solutions real-world use also presented. Given rapid advancements area, review attempts briefly cover many that play ophthalmic space, with focus improving quality delivery.
Language: Английский
Citations
7Future Internet, Journal Year: 2024, Volume and Issue: 16(7), P. 219 - 219
Published: June 22, 2024
AI chatbots have emerged as powerful tools for providing text-based solutions to a wide range of everyday challenges. Selecting the appropriate chatbot is crucial optimising outcomes. This paper presents comprehensive comparative analysis five leading chatbots: ChatGPT, Bard, Llama, Ernie, and Grok. The based on systematic review 28 scholarly articles. indicates that developed by OpenAI, excels in educational, medical, humanities, writing applications but struggles with real-time data accuracy lacks open-source flexibility. powered Google, leverages internet problem solving shows potential competitive quiz environments, albeit performance variability inconsistencies responses. an model from Meta, demonstrates significant promise medical contexts, natural language processing, personalised educational tools, yet it requires substantial computational resources. Baidu, specialises Chinese tasks, thus localised advantages may not extend globally due restrictive policies. Grok, Xai still its early stages, engaging, interactions, humour, mathematical reasoning capabilities, full remains be evaluated through further development empirical testing. findings underscore context-dependent utility each absence singularly superior chatbot. Future research should expand include wider fields, explore practical applications, address concerns related privacy, ethics, security, responsible deployment these technologies.
Language: Английский
Citations
5Frontiers in Artificial Intelligence, Journal Year: 2025, Volume and Issue: 8
Published: Feb. 13, 2025
Introduction The rapid evolution of the Internet Things (IoT) and Artificial Intelligence (AI) has opened new possibilities for public healthcare. Effective integration these technologies is essential to ensure precise efficient healthcare delivery. This study explores application IoT-enabled, AI-driven systems detecting managing Dry Eye Disease (DED), emphasizing use prompt engineering enhance system performance. Methods A specialized mechanism was developed utilizing OpenAI GPT-4.0 ERNIE Bot-4.0 APIs assess urgency medical attention based on 5,747 simulated patient complaints. Bidirectional Encoder Representations from Transformers (BERT) machine learning model employed text classification differentiate urgent non-urgent cases. User satisfaction evaluated through composite scores derived Service Experiences (SE) Medical Quality (MQ) assessments. Results comparison between prompted non-prompted queries revealed a significant accuracy increase 80.1% 99.6%. However, this improvement accompanied by notable rise in response time, resulting decrease SE (95.5 84.7) but substantial MQ (73.4 96.7). These findings indicate trade-off user satisfaction. Discussion highlights critical role improving AI-based services. While enhanced achievable, careful must be given balancing time Future research should optimize structures, explore dynamic prompting approaches, prioritize real-time evaluations address identified challenges maximize potential IoT-integrated AI applications.
Language: Английский
Citations
0Frontiers in Artificial Intelligence, Journal Year: 2025, Volume and Issue: 8
Published: March 12, 2025
The integration of artificial intelligence (AI) into ophthalmic diagnostics has the potential to significantly enhance diagnostic accuracy and interpretability, thereby supporting clinical decision-making. However, a major challenge in AI-driven medical applications is lack transparency, which limits clinicians' trust automated recommendations. This study investigates application machine learning techniques by integrating knowledge graphs with contrastive utilizing "clinical profile" prompts refine performance ophthalmology-specific large language model, MeEYE, built on CHATGLM3-6B architecture. approach aims improve model's ability capture clinically relevant features while enhancing both explainability predictions. employs novel methodological framework that incorporates domain-specific through enhances feature representation using learning. MeEYE model fine-tuned structured knowledge, enabling it better distinguish subtle yet significant features. Additionally, are incorporated further contextual understanding precision. proposed method evaluated comprehensive benchmarking, including quantitative assessments case studies, ensure its efficacy real-world diagnosis. experimental findings demonstrate improves interpretability. Comparative analyses against baseline models reveal identification conditions higher precision clarity. Furthermore, generate transparent AI recommendations substantiated rigorous evaluation, highlighting for implementation. results underscore importance explainable diagnostics, particularly ophthalmology, where transparency critical acceptance utility. By incorporating advanced techniques, not only but also ensures AI-generated insights interpretable reliable These suggest frameworks can address key challenges ultimately contributing improved patient outcomes. Future research should explore adaptability this across various domains advance AI-assisted systems.
Language: Английский
Citations
0Journal of Neuro-Ophthalmology, Journal Year: 2024, Volume and Issue: 44(3), P. 308 - 318
Published: July 5, 2024
Neuro-ophthalmology frequently requires a complex and multi-faceted clinical assessment supported by sophisticated imaging techniques in order to assess disease status. The current approach diagnosis substantial expertise time. emergence of AI has brought forth innovative solutions streamline enhance this diagnostic process, which is especially valuable given the shortage neuro-ophthalmologists. Machine learning algorithms, particular, have demonstrated significant potential interpreting data, identifying subtle patterns, aiding clinicians making more accurate timely while also supplementing nonspecialist evaluations neuro-ophthalmic disease.
Language: Английский
Citations
3Journal of Medical Internet Research, Journal Year: 2024, Volume and Issue: 26, P. e60226 - e60226
Published: Oct. 15, 2024
Background Artificial intelligence (AI) chatbots such as ChatGPT are expected to impact vision health care significantly. Their potential optimize the consultation process and diagnostic capabilities across range of ophthalmic subspecialties have yet be fully explored. Objective This study aims investigate performance AI in recommending outpatient registration diagnosing eye diseases within clinical case profiles. Methods cross-sectional used cases from Chinese Standardized Resident Training–Ophthalmology (2nd Edition). For each case, 2 profiles were created: patient with history (Hx) examination (Hx+Ex). These served independent queries for GPT-3.5 GPT-4.0 (accessed March 5 18, 2024). Similarly, 3 residents posed same a questionnaire format. The accuracy subspecialty was primarily evaluated using Hx top-ranked diagnosis top suggestions (do-not-miss diagnosis) assessed Hx+Ex gold standard judgment published, official diagnosis. Characteristics incorrect diagnoses by also analyzed. Results A total 208 12 analyzed (104 104 profiles). profiles, GPT-3.5, GPT-4.0, showed comparable (66/104, 63.5%; 81/104, 77.9%; 72/104, 69.2%, respectively; P=.07), ocular trauma, retinal diseases, strabismus amblyopia achieving accuracies. both demonstrated higher than (62/104, 59.6% 63/104, 60.6% vs 41/104, 39.4%; P=.003 P=.001, respectively). Accuracy do-not-miss improved (79/104, 76% 68/104, 65.4% 51/104, 49%; P<.001 P=.02, highest accuracies observed glaucoma; lens diseases; eyelid, lacrimal, orbital diseases. recorded fewer top-3 (25/42, 60% 53/63, 84%; P=.005) more partially correct (21/42, 50% 7/63 11%; P<.001) while had completely (27/63, 43% 7/42, 17%; less precise (22/63, 35% 5/42, 12%; P=.009). Conclusions intermediate registration. While underperformed, approached numerically surpassed differential show promise facilitating However, their integration into decision-making requires validation.
Language: Английский
Citations
3Postgraduate Medicine, Journal Year: 2024, Volume and Issue: 136(8), P. 841 - 846
Published: Oct. 17, 2024
To perform a comparative analysis of the three-level triage protocol conducted by nurses and emergency medicine doctors with use ChatGPT, Gemini, Pi, which are recognized artificial intelligence (AI) models widely used in daily life.
Language: Английский
Citations
3BMC Medical Informatics and Decision Making, Journal Year: 2024, Volume and Issue: 24(1)
Published: Nov. 26, 2024
The large language models (LLMs), most notably ChatGPT, released since November 30, 2022, have prompted shifting attention to their use in medicine, particularly for supporting clinical decision-making. However, there is little consensus the medical community on how LLM performance contexts should be evaluated. We performed a literature review of PubMed identify publications between December 1, and April 2024, that discussed assessments LLM-generated diagnoses or treatment plans. selected 108 relevant articles from analysis. frequently used LLMs were GPT-3.5, GPT-4, Bard, LLaMa/Alpaca-based models, Bing Chat. five criteria scoring outputs "accuracy", "completeness", "appropriateness", "insight", "consistency". defining high-quality been consistently by researchers over past 1.5 years. identified high degree variation studies reported findings assessed performance. Standardized reporting qualitative evaluation metrics assess quality can developed facilitate research healthcare.
Language: Английский
Citations
3Cureus, Journal Year: 2024, Volume and Issue: unknown
Published: June 16, 2024
To evaluate the efficiency of three artificial intelligence (AI) chatbots (ChatGPT-3.5 (OpenAI, San Francisco, California, United States), Bing Copilot (Microsoft Corporation, Redmond, Washington, Google Gemini (Google LLC, Mountain View, States)) in assisting ophthalmologist diagnostic approach and management challenging ophthalmic cases compare their performance with that a practicing human specialist. The secondary aim was to assess short- medium-term consistency ChatGPT's responses.
Language: Английский
Citations
2Journal of Chemical Education, Journal Year: 2024, Volume and Issue: 101(9), P. 3666 - 3675
Published: Aug. 7, 2024
The rapid development of artificial intelligence (AI) has transformed chatbots into generative pre-trained transformers (GPTs) capable performing various tasks. use GPTs is expanding to learning, including natural sciences like chemistry. can assist students in understanding and solving chemistry problems. However, there are potential negative impacts using GPTs. This study aims explore Indonesian university students' perspectives on for learning. research used a case method collected data through questionnaires, interviews, GPT usage logs, which were then analyzed thematically. revealed that learning due perceived usefulness, ease use, emotional aspects, benefits, social influence. Students appreciate answers being easy understand, detailed, reliable, fairly accurate, fast, helpful. also recognize be unreliable, difficult not always potentially unethical. evaluate responses by stimulating thoughts, confirming answers, integrating with other sources, directly copying responses, paraphrasing before use. They aware ethical considerations, drawbacks, limitations associated Findings pertaining motives, constraints, the evaluation answer quality its utilization serve as indicators proper application educational contexts. In many countries, Indonesia, where lack regulations, concrete policies necessary ensure integration education.
Language: Английский
Citations
2