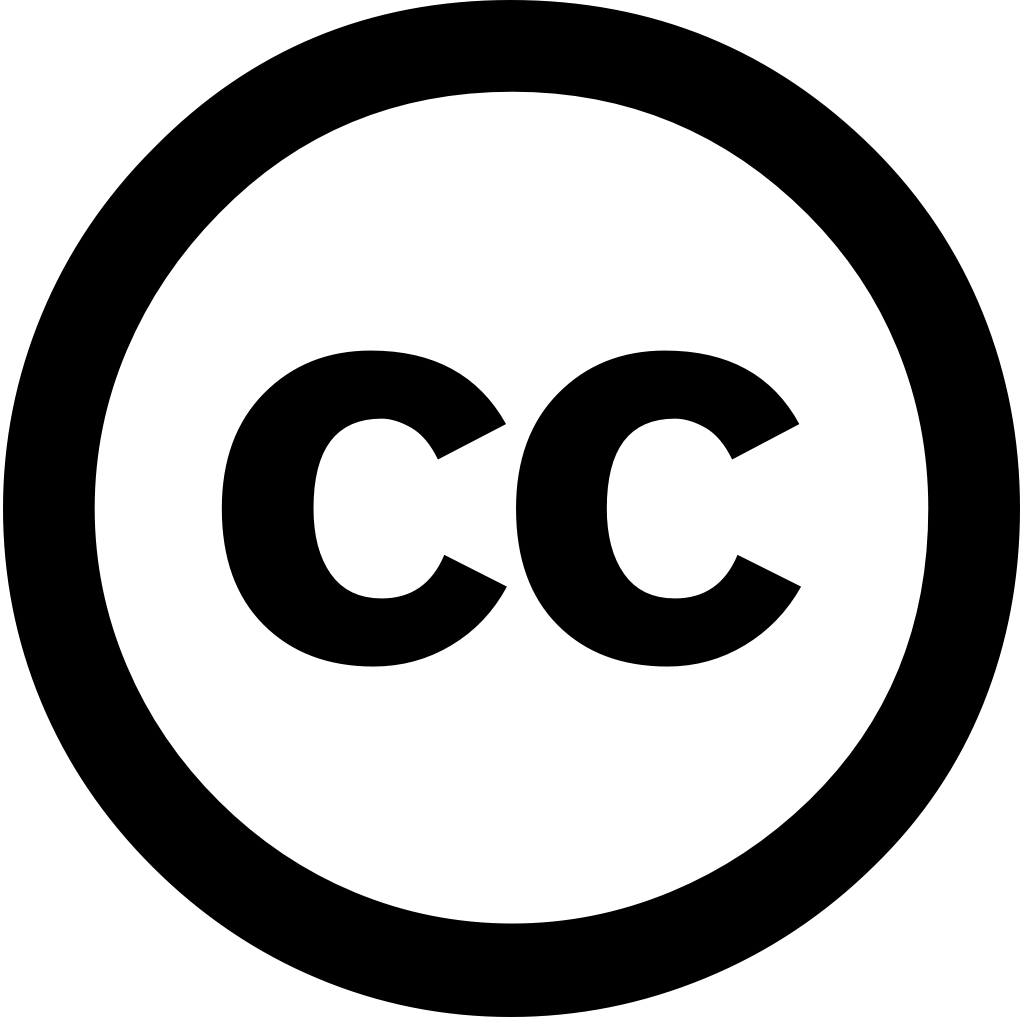
Mathematics, Journal Year: 2025, Volume and Issue: 13(7), P. 1059 - 1059
Published: March 25, 2025
Accuracy, recall, specificity, and precision are key performance measures for binary classifiers. To obtain these measures, the probabilities generated by classifiers must be converted into deterministic labels using a threshold. Exhaustive search methods can computationally expensive, prompting need more efficient solution. We propose an integer linear programming (ILP) formulation to find threshold that maximizes any combination of measures. Simulations experiments on four real-world datasets demonstrate our approach identifies optimal orders magnitude faster than exhaustive search. This work establishes ILP as tool optimizing classifier performance.
Language: Английский