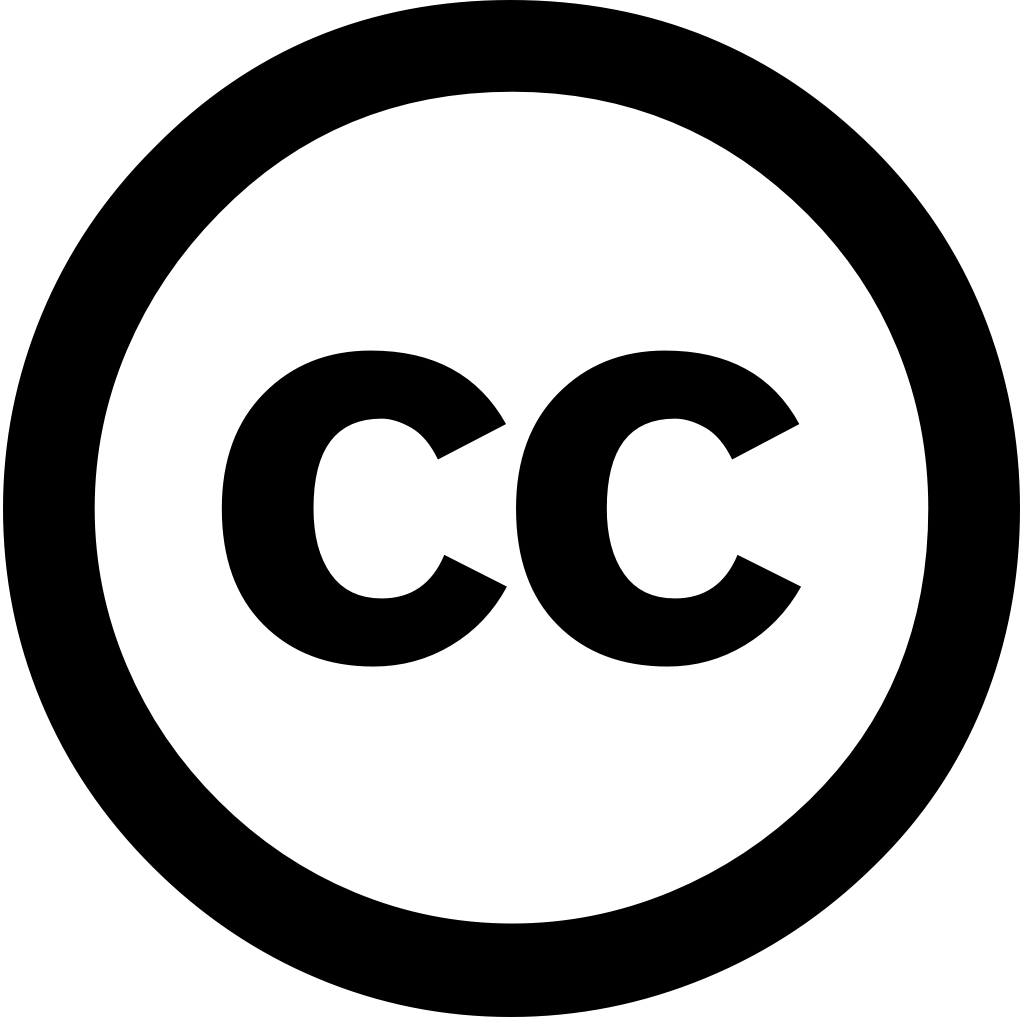
Results in Engineering, Journal Year: 2025, Volume and Issue: unknown, P. 104636 - 104636
Published: March 1, 2025
Language: Английский
Results in Engineering, Journal Year: 2025, Volume and Issue: unknown, P. 104636 - 104636
Published: March 1, 2025
Language: Английский
Bioengineering, Journal Year: 2024, Volume and Issue: 11(10), P. 1016 - 1016
Published: Oct. 12, 2024
Ensemble Learning (EL) has been used for almost ten years to classify heart diseases, but it is still difficult grasp how the “black boxes”, or non-interpretable models, behave inside. Predicting disease crucial healthcare, since allows prompt diagnosis and treatment of patient’s true state. Nonetheless, forecast illness with any degree accuracy. In this study, we have suggested a framework prediction based on Explainable artificial intelligence (XAI)-based hybrid such as LightBoost XGBoost algorithms. The main goals are build predictive models apply SHAP (SHapley Additive expPlanations) LIME (Local Interpretable Model-agnostic Explanations) analysis improve interpretability models. We carefully construct our systems test different ensemble learning algorithms determine which model best (HDP). approach promotes transparency when examining these widespread health issues. By combining XAI, important factors risk signals that underpin co-occurrence made visible. accuracy, precision, recall were evaluate their efficacy. This study highlights healthcare be transparent recommends inclusion XAI medical decisionmaking.
Language: Английский
Citations
6Frontiers in Neurology, Journal Year: 2025, Volume and Issue: 16
Published: March 14, 2025
This study focused on the relationship between heart rate and likelihood of death within 28 days in patients with cerebral infarction without comorbidity atrial fibrillation, using patient data extracted from MIMIC-IV database. involved a retrospective analysis clinical 1,643 individuals who were admitted to ICU. To investigate role determining survival, we applied variety statistical techniques such as Cox regression models, survival Kaplan-Meier plots, spline-based models. In addition, performed analyses by subgroups identify any potential variables that could influence association HR 28-day mortality. univariate multivariate analyses, elevated was strongly associated higher mortality, even after adjusting for confounders age, sex, comorbidities, scores.(HR:1.01, 95%,CI:1.01 ~ 1.02, p = 0.019) showed > 90 beats/min had significantly lower probability survival. Restricted cubic spline (RCS) confirmed nonlinear Subgroup demonstrated an interaction factors hypertension mechanical ventilation status. highlights prognostic significance independent predictor mortality do not have fibrillation.
Language: Английский
Citations
0Results in Engineering, Journal Year: 2025, Volume and Issue: unknown, P. 104636 - 104636
Published: March 1, 2025
Language: Английский
Citations
0