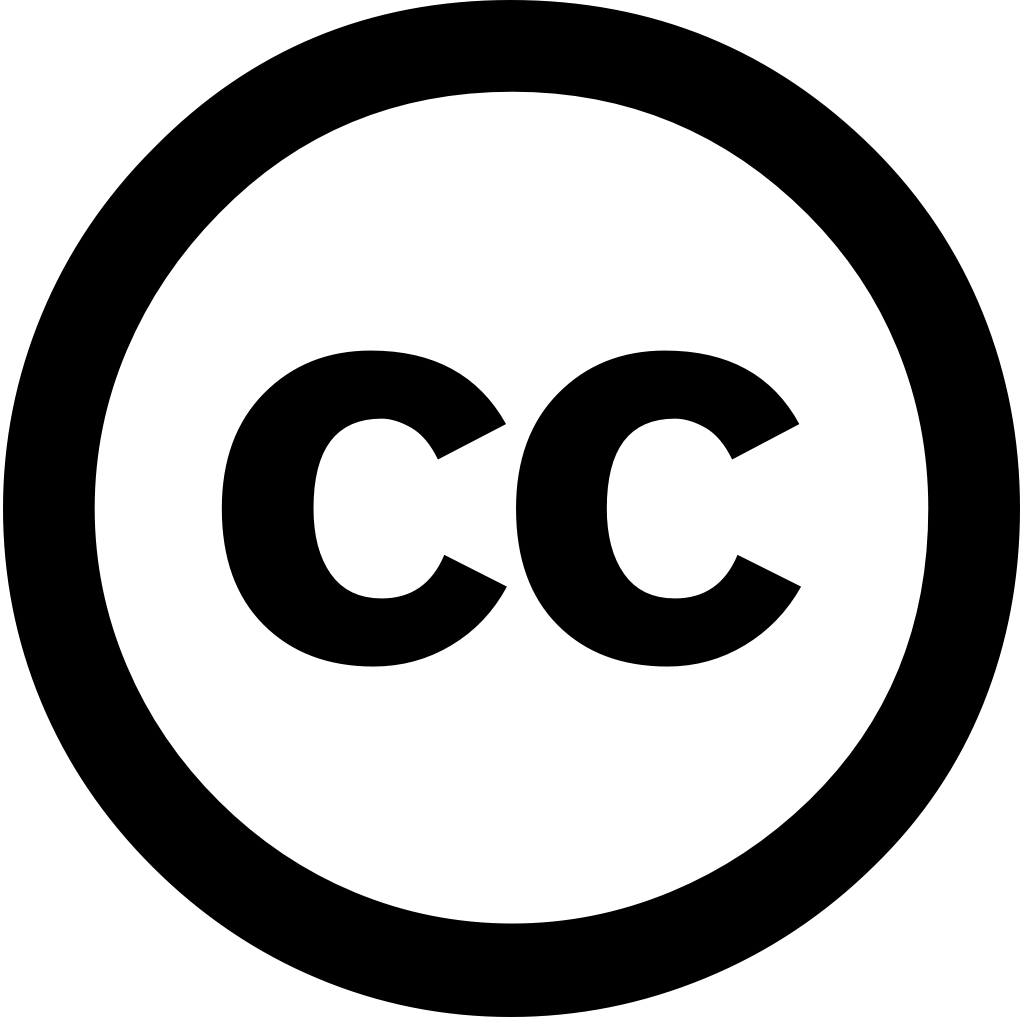
Sensors, Journal Year: 2025, Volume and Issue: 25(6), P. 1895 - 1895
Published: March 18, 2025
Freezing of gait (FOG) is a motor symptom experienced by people with Parkinson’s Disease (PD) where they feel like are glued to the floor. Accurate and continuous detection needed for effective cueing prevent or shorten FOG episodes. A convolutional neural network (CNN) was developed detect episodes in data recorded from an inertial measurement unit (IMU) on PD patient’s ankle under semi-free living conditions. Data were split into two sets: one all movements another walking turning activities relevant detection. The CNN model evaluated using five-fold cross-validation (5Fold-CV), leave-one-subject-out (LOSO-CV), performance metrics such as accuracy, sensitivity, precision, F1-score, AUROC; 24 participants collected, excluding three no For activities, achieved AUROC = 0.9596 5Fold-CV 0.9275 LOSO-CV. When included, dropped 0.8888 0.9017 LOSO-CV; effectively detected movement scenarios but struggled distinguishing other inactive states sitting standing semi-free-living environments.
Language: Английский