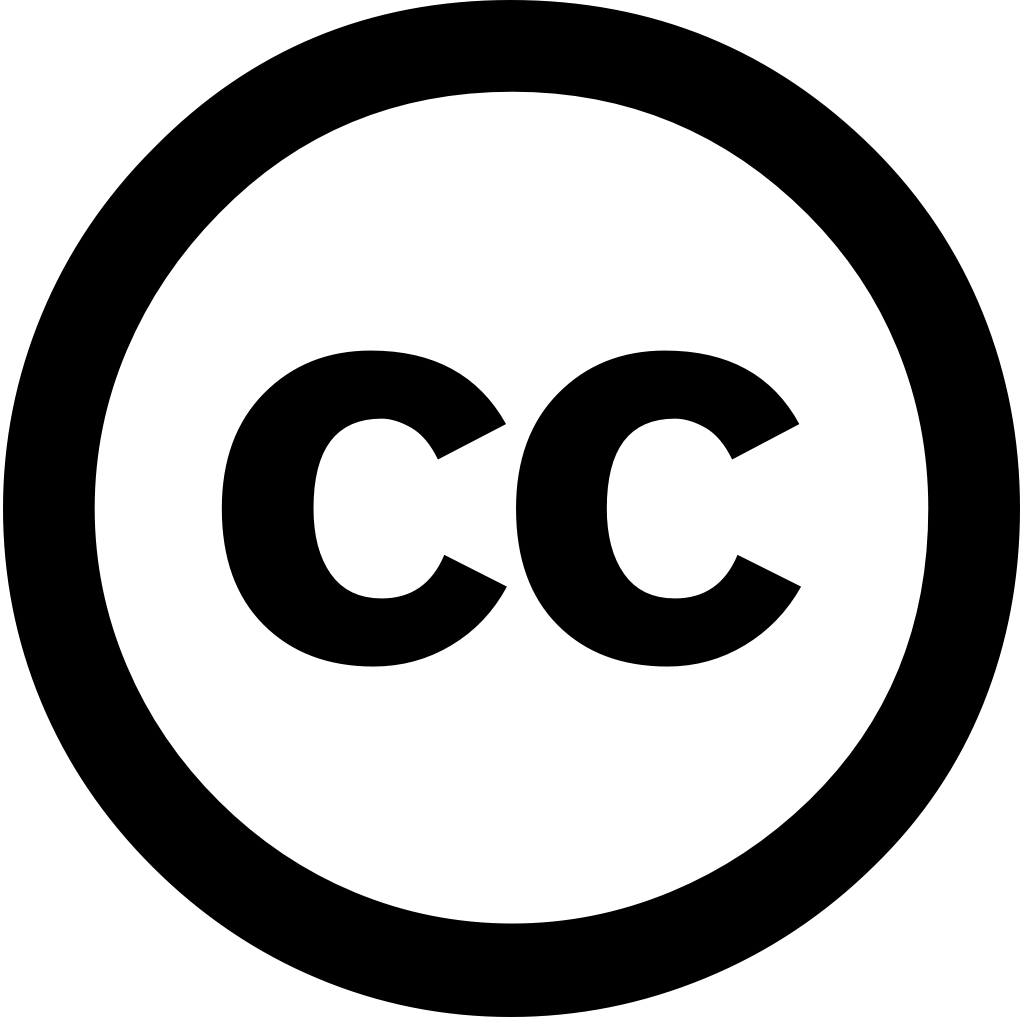
Diagnostics, Journal Year: 2025, Volume and Issue: 15(7), P. 854 - 854
Published: March 27, 2025
Background: Examining chest radiograph images (CXR) is an intricate and time-consuming process, sometimes requiring the identification of many anomalies at same time. Lung segmentation key to overcoming this challenge through different deep learning (DL) techniques. Many researchers are working improve performance efficiency lung models. This article presents a DL-based approach accurately identify mask region in CXR assist radiologists recognizing early signs high-risk diseases. Methods: paper proposes novel technique, Lightweight Residual U-Net, combining strengths convolutional block attention module (CBAM), Atrous Spatial Pyramid Pooling (ASPP) block, module, which consists only 3.24 million trainable parameters. Furthermore, proposed model has been trained using both RELU LeakyReLU activation functions, with yielding superior performance. The study indicates that Dice loss function more effective achieving better results. Results: evaluated on three benchmark datasets: JSRT, SZ, MC, score 98.72%, 97.49%, 99.08%, respectively, outperforming state-of-the-art Conclusions: Using capabilities DL cutting-edge processes, improves current efforts enhance for serious
Language: Английский