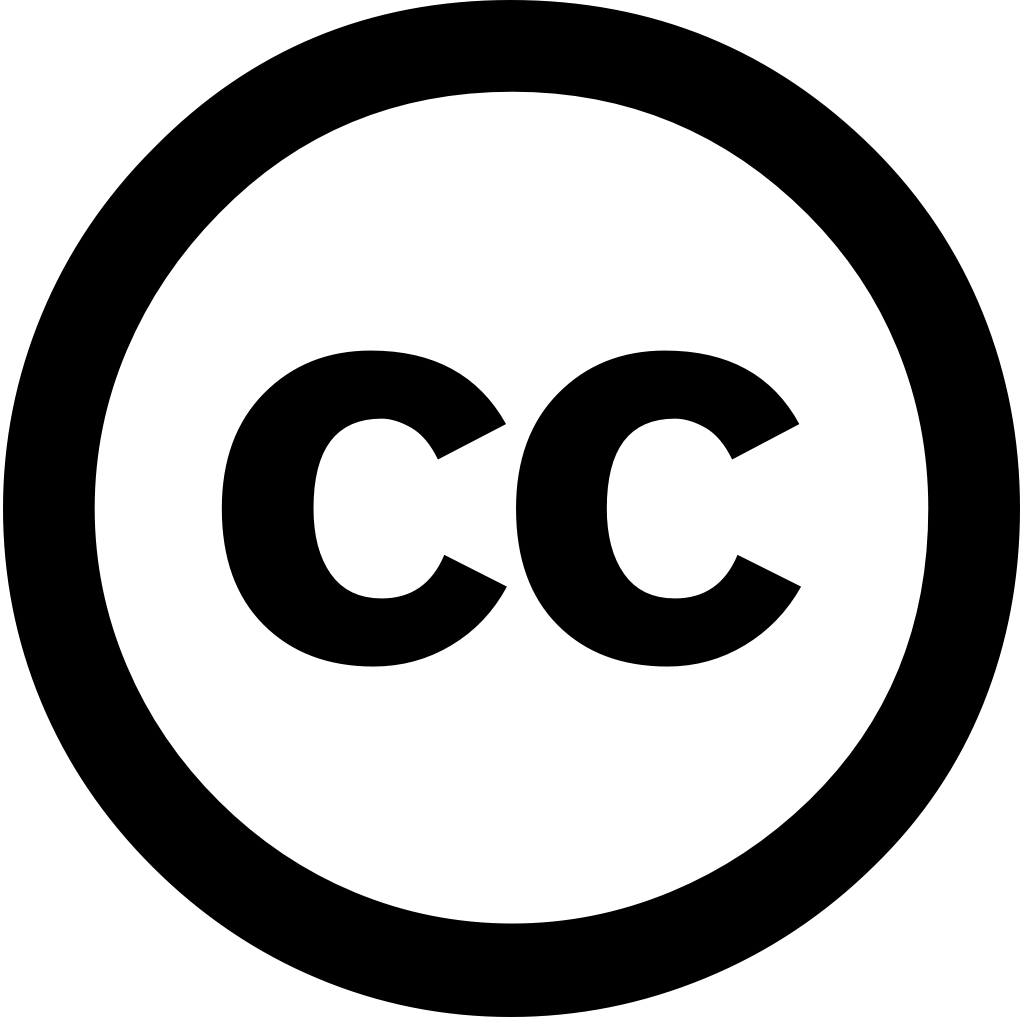
Clinical and Experimental Obstetrics & Gynecology, Journal Year: 2024, Volume and Issue: 51(12)
Published: Dec. 18, 2024
Language: Английский
Clinical and Experimental Obstetrics & Gynecology, Journal Year: 2024, Volume and Issue: 51(12)
Published: Dec. 18, 2024
Language: Английский
npj Digital Medicine, Journal Year: 2025, Volume and Issue: 8(1)
Published: Jan. 2, 2025
In resource-constrained countries like India, mammography-based breast screening is challenging to implement. This state-wide study, funded by the Government of Punjab, evaluated use Thermalytix, a low-cost, radiation-free AI tool, for cancer screening. Community health workers, trained raise awareness, mobilized women aged 30 and above Thermalytix triaged into five risk categories based on thermal images, with high-risk recalled diagnostic imaging. Over 18 months, 15,069 were screened across 183 locations in Punjab. The median age was 41 years, 69.9% asymptomatic. Of 460 testing positive (recall rate 3.1%), 268 underwent follow-up imaging, 27 confirmed cancer, yielding detection 0.18%. predictive value biopsy performed 81.81%, interval 21 days, therapy initiation within days. study demonstrates potential effective population-level low-resource settings.
Language: Английский
Citations
1Diagnostics, Journal Year: 2025, Volume and Issue: 15(5), P. 549 - 549
Published: Feb. 25, 2025
Background: Early and accurate detection of breast cancer is crucial for improving treatment outcomes survival rates. To achieve this, innovative imaging technologies such as microwave radiometry (MWR)-which measures internal tissue temperature-combined with advanced diagnostic methods like deep learning are essential. Methods: address this need, we propose a hierarchical self-contrastive model analyzing MWR data, called Joint-MWR (J-MWR). J-MWR focuses on comparing temperature variations within an individual by corresponding sub-regions the two breasts, rather than across different samples. This approach enables subtle thermal abnormalities that may indicate potential issues. Results: We evaluated dataset 4932 patients, demonstrating improvements over existing MWR-based neural networks conventional contrastive methods. The achieved Matthews correlation coefficient 0.74 ± 0.02, reflecting its robust performance. Conclusions: These results emphasize intra-subject comparison use to replicate traditional feature extraction techniques, thereby accuracy while maintaining high generalizability.
Language: Английский
Citations
0Salud Ciencia y Tecnología, Journal Year: 2025, Volume and Issue: 5, P. 1518 - 1518
Published: March 18, 2025
This paper presents a robust deep learning framework for thermal breast cancer detection using grayscale images. Leveraging pre-trained VGG16 model, we classify images into 'normal' and 'abnormal' categories, integrating data augmentation techniques to improve model generalization. Grad-CAM visualization elucidates the regions influencing predictions, aiding interpretability. Testing on DMR-IR dataset yielded remarkable AUC-ROC score of 0.97 accuracy exceeding 94%. These findings underscore potential imaging in non-invasive screening, bridging diagnostic with interpretability clinical application.
Language: Английский
Citations
0Technologies, Journal Year: 2024, Volume and Issue: 13(1), P. 7 - 7
Published: Dec. 26, 2024
Breast cancer remains one of the most prevalent and deadly cancers affecting women worldwide. Early detection is crucial, particularly for younger women, as traditional screening methods like mammography often struggle with accuracy in cases dense breast tissue. Infrared thermography offers a non-invasive imaging alternative that enhances early by capturing subtle thermal variations indicative abnormalities. This study investigates compares performance various deep learning machine models analyzing thermographic data to classify tissue healthy, benign, or malignant. To maximize accuracy, preprocessing, feature extraction, dimensionality reduction were implemented isolate distinguishing characteristics across types. Leveraging advanced extraction visualization techniques inspired geospatial methodologies, we evaluated several architectures classical classifiers using DRM-IR Thermography Mendeley datasets. Among tested models, ResNet152 architecture combined Support Vector Machine (SVM) classifier delivered highest performance, achieving 97.62% 95.79% precision, 98.53% recall, 94.52% specificity, an F1 score 97.16%, area under curve (AUC) 99%, latency 0.06 s, CPU utilization 88.66%. These findings underscore potential integrating infrared approaches significantly improve efficiency detection, supporting its role valuable tool diagnosis.
Language: Английский
Citations
0Clinical and Experimental Obstetrics & Gynecology, Journal Year: 2024, Volume and Issue: 51(12)
Published: Dec. 18, 2024
Language: Английский
Citations
0