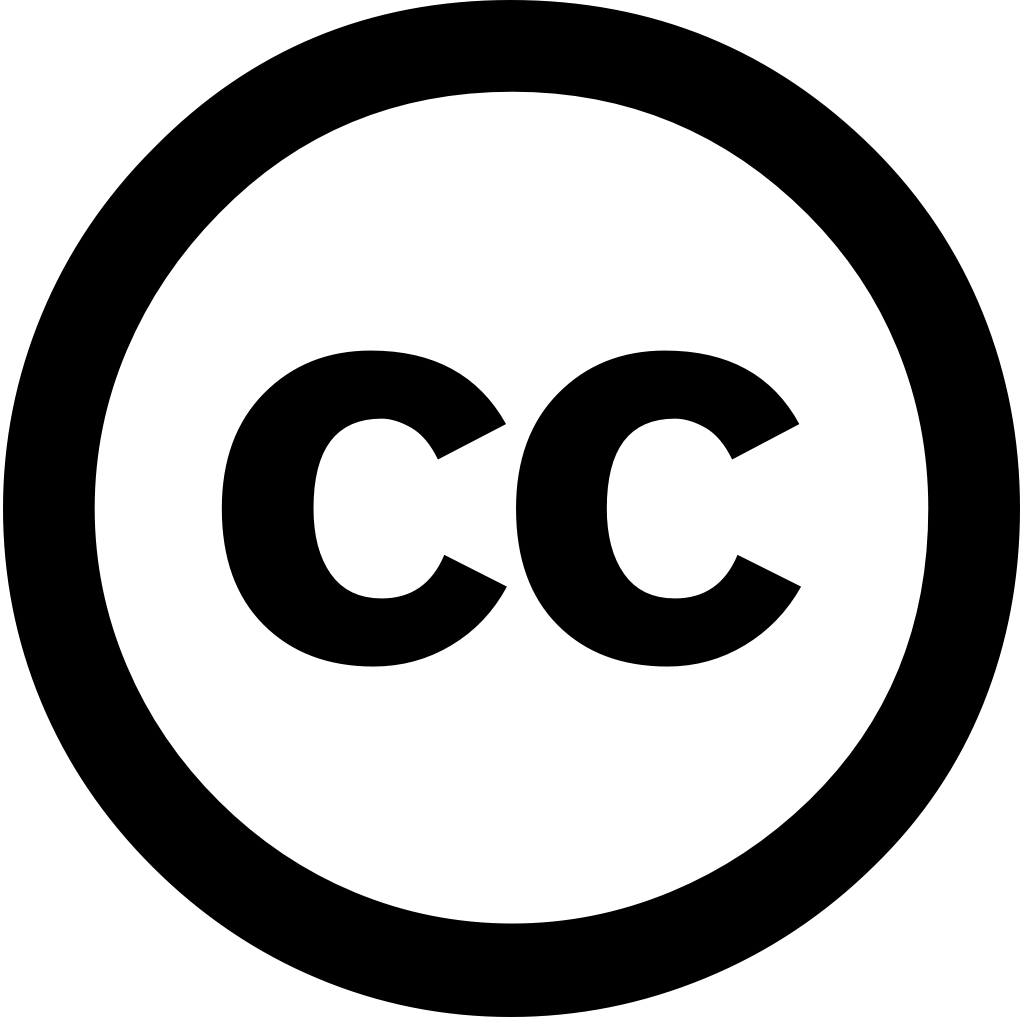
Foods, Journal Year: 2025, Volume and Issue: 14(8), P. 1379 - 1379
Published: April 17, 2025
Precise detection of meat freshness levels is essential for food consumer safety and real-time quality monitoring. This study aims to achieve the high-accuracy chilled mutton by integrating hyperspectral imaging with deep learning methods. Although data can effectively capture changes in freshness, sparse raw spectra require optimal processing strategies minimize redundancy. Therefore, this employs a multi-stage approach enhance purity feature spectra. Meanwhile, address issues such as overlapping categories, imbalanced sample distributions, insufficient intermediate features, we propose Dual-Branch Hierarchical Spectral Feature-Aware Network (DBHSNet) detection. First, at interaction stage, PBCA module addresses drawback that global local branches conventional dual-branch framework tend perceive spectral features independently. By enabling effective information exchange bidirectional flow between two branches, injecting positional into each band, model’s awareness sequential bands enhanced. Second, fusion task-driven MSMHA introduced dynamics variation accumulation different metabolites. leveraging multi-head attention cross-scale fusion, model more captures both overall trends fine-grained details. Third, classification output dynamic loss weighting set according training epochs relative losses balance performance, mitigating impact insufficiently discriminative features. The results demonstrate DBHSNet enables precise assessment achieving up 7.59% higher accuracy than methods under same preprocessing conditions, while maintaining superior weighted metrics. Overall, offers novel provides valuable support monitoring cold-chain systems.
Language: Английский