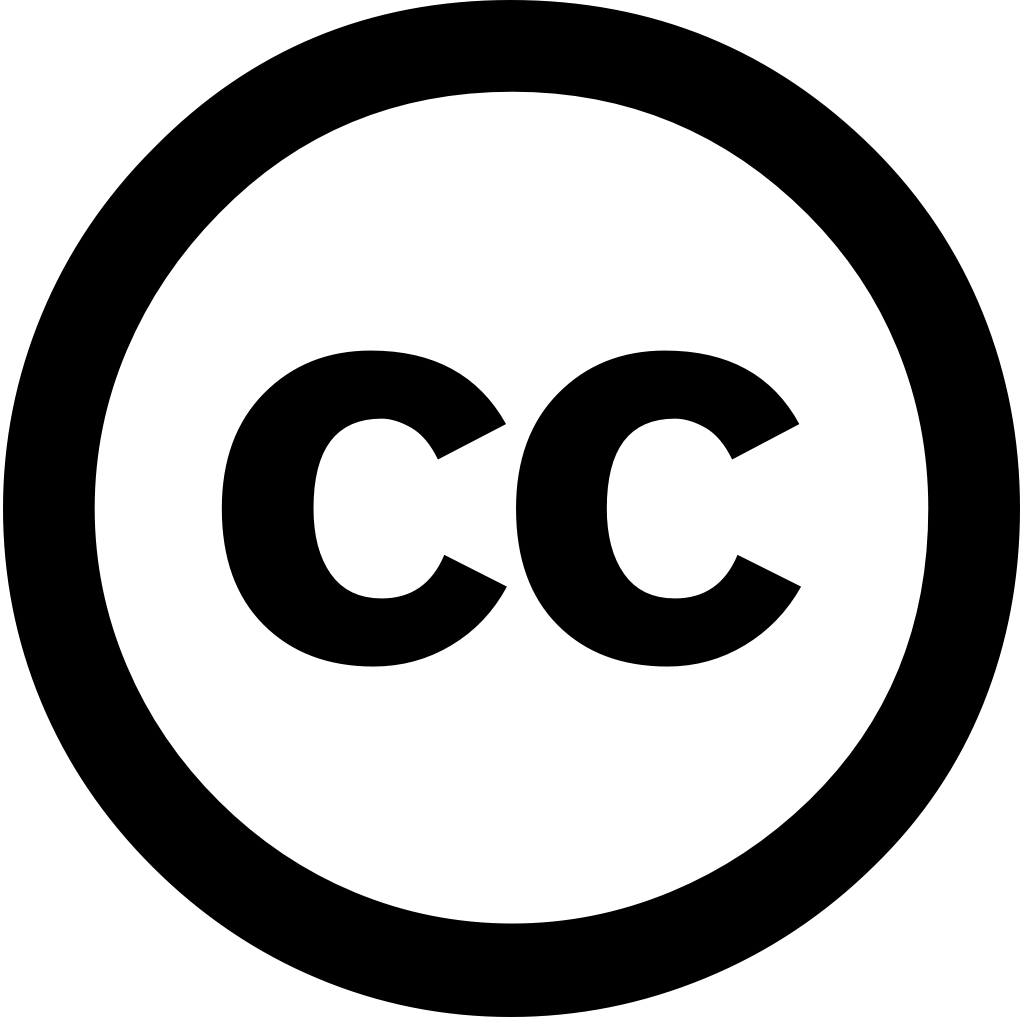
Brain Sciences, Journal Year: 2025, Volume and Issue: 15(5), P. 460 - 460
Published: April 27, 2025
Background: In brain–computer interfaces (BCIs), transformer-based models have found extensive application in motor imagery (MI)-based EEG signal recognition. However, for subject-independent recognition, these face challenges: low sensitivity to spatial dynamics of neural activity and difficulty balancing high temporal resolution features with manageable computational complexity. The overarching objective is address critical issues. Methods: We introduce Mirror Contrastive Learning Sliding Window Transformer (MCL-SWT). Inspired by left/right hand inducing event-related desynchronization (ERD) the contralateral sensorimotor cortex, we develop a mirror contrastive loss function. It segregates feature spaces signals from ERD locations while curtailing variability sharing similar locations. computes self-attention scores over features, enabling efficient capture global dependencies. Results: Evaluated on benchmark datasets MI MCL-SWT achieves classification accuracies 66.48% 75.62%, outperforming State-of-the-Art 2.82% 2.17%, respectively. Ablation studies validate efficacy both sliding window mechanism. Conclusions: These findings underscore MCL-SWT’s potential as robust, interpretable framework By addressing existing challenges, could significantly advance BCI technology development.
Language: Английский