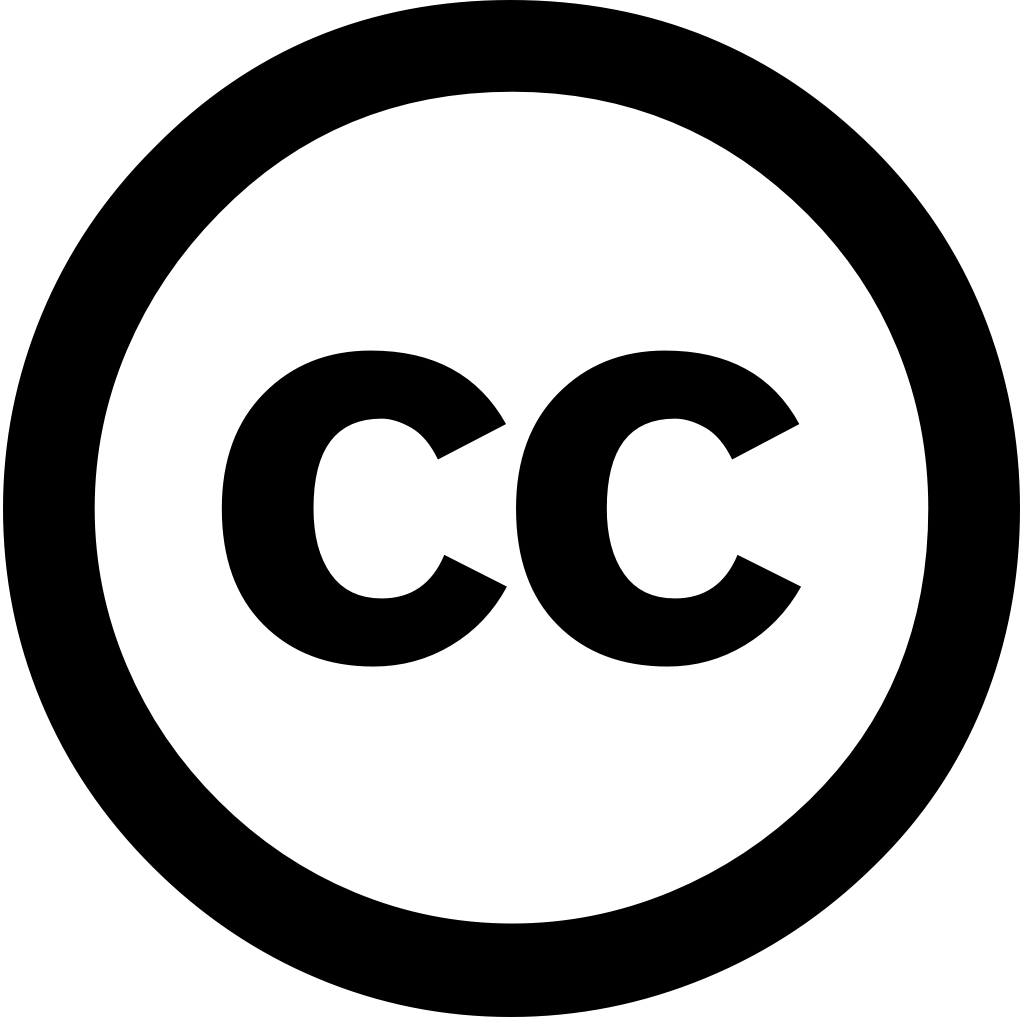
Gut, Journal Year: 2022, Volume and Issue: 71(7), P. 1259 - 1265
Published: April 13, 2022
Language: Английский
Gut, Journal Year: 2022, Volume and Issue: 71(7), P. 1259 - 1265
Published: April 13, 2022
Language: Английский
Biotechnology Advances, Journal Year: 2021, Volume and Issue: 49, P. 107739 - 107739
Published: March 29, 2021
Language: Английский
Citations
525Computational and Structural Biotechnology Journal, Journal Year: 2021, Volume and Issue: 19, P. 3735 - 3746
Published: Jan. 1, 2021
Increased availability of high-throughput technologies has generated an ever-growing number omics data that seek to portray many different but complementary biological layers including genomics, epigenomics, transcriptomics, proteomics, and metabolomics. New insight from these have been obtained by machine learning algorithms produced diagnostic classification biomarkers. Most biomarkers date however only include one omic measurement at a time thus do not take full advantage recent multi-omics experiments now capture the entire complexity systems. Multi-omics integration strategies are needed combine knowledge brought each layer. We summarized most methods/ frameworks into five strategies: early, mixed, intermediate, late hierarchical. In this mini-review, we focus on challenges existing paying special attention applications.
Language: Английский
Citations
368Information Fusion, Journal Year: 2020, Volume and Issue: 66, P. 111 - 137
Published: Sept. 15, 2020
Language: Английский
Citations
292Scientific Reports, Journal Year: 2021, Volume and Issue: 11(1)
Published: March 26, 2021
Cox Proportional Hazards (CPH) analysis is the standard for survival in oncology. Recently, several machine learning (ML) techniques have been adapted this task. Although they shown to yield results at least as good classical methods, are often disregarded because of their lack transparency and little no explainability, which key adoption clinical settings. In paper, we used data from Netherlands Cancer Registry 36,658 non-metastatic breast cancer patients compare performance CPH with ML (Random Survival Forests, Support Vector Machines, Extreme Gradient Boosting [XGB]) predicting using [Formula: see text]-index. We demonstrated that our dataset, ML-based models can perform regression ([Formula: text]-index text]), case XGB even better text]). Furthermore, Shapley Additive Explanation (SHAP) values explain models' predictions. concluded difference be attributed XGB's ability model nonlinearities complex interactions. also investigated impact specific features on predictions well corresponding insights. Lastly, showed explainable generate explicit knowledge how make predictions, crucial increasing trust innovative oncology healthcare overall.
Language: Английский
Citations
235Briefings in Bioinformatics, Journal Year: 2021, Volume and Issue: 23(1)
Published: Oct. 7, 2021
Abstract High-throughput next-generation sequencing now makes it possible to generate a vast amount of multi-omics data for various applications. These have revolutionized biomedical research by providing more comprehensive understanding the biological systems and molecular mechanisms disease development. Recently, deep learning (DL) algorithms become one most promising methods in analysis, due their predictive performance capability capturing nonlinear hierarchical features. While integrating translating into useful functional insights remain biggest bottleneck, there is clear trend towards incorporating analysis help explain complex relationships between layers. Multi-omics role improve prevention, early detection prediction; monitor progression; interpret patterns endotyping; design personalized treatments. In this review, we outline roadmap integration using DL offer practical perspective advantages, challenges barriers implementation data.
Language: Английский
Citations
205Trends in cancer, Journal Year: 2019, Volume and Issue: 5(3), P. 157 - 169
Published: Feb. 28, 2019
Language: Английский
Citations
177IEEE Transactions on Biomedical Circuits and Systems, Journal Year: 2020, Volume and Issue: 14(6), P. 1138 - 1159
Published: Nov. 6, 2020
The advent of dedicated Deep Learning (DL) accelerators and neuromorphic processors has brought on new opportunities for applying both Spiking Neural Network (SNN) algorithms to healthcare biomedical applications at the edge.This can facilitate advancement medical Internet Things (IoT) systems Point Care (PoC) devices.In this paper, we provide a tutorial describing how various technologies including emerging memristive devices, Field Programmable Gate Arrays (FPGAs), Complementary Metal Oxide Semiconductor (CMOS) be used develop efficient DL solve wide variety diagnostic, pattern recognition, signal processing problems in healthcare.Furthermore, explore spiking complement their counterparts signals.The is augmented with case studies vast literature neural network hardware as applied domain.We benchmark platforms by performing sensor fusion task combining electromyography (EMG) signals computer vision.Comparisons are made between embedded AI terms inference latency energy.Finally, our analysis field share perspective advantages, disadvantages, challenges, that introduce domains.
Language: Английский
Citations
177Scientific Reports, Journal Year: 2021, Volume and Issue: 11(1)
Published: June 29, 2021
Abstract The age of precision medicine demands powerful computational techniques to handle high-dimensional patient data. We present MultiSurv, a multimodal deep learning method for long-term pan-cancer survival prediction. MultiSurv uses dedicated submodels establish feature representations clinical, imaging, and different omics data modalities. A fusion layer aggregates the representations, prediction submodel generates conditional probabilities follow-up time intervals spanning several decades. is first non-linear non-proportional that leverages In addition, can missing data, including single values complete was applied from 33 cancer types yields accurate curves. quantitative comparison with previous methods showed Multisurv achieves best results according time-dependent metrics. also generated visualizations learned representation which revealed insights on characteristics heterogeneity.
Language: Английский
Citations
154Computational and Structural Biotechnology Journal, Journal Year: 2020, Volume and Issue: 18, P. 1466 - 1473
Published: Jan. 1, 2020
With the evolution of biotechnology and introduction high throughput sequencing, researchers have ability to produce analyze vast amounts genomics data. Since big data, most bioinformatics algorithms are based on machine learning methodologies, lately deep learning, identify patterns, make predictions model progression or treatment a disease. Advances in created an unprecedented momentum biomedical informatics given rise new computational biology research areas. It is evident that models can provide higher accuracies specific tasks than state art methodologies. Given growing trend application architectures research, this mini review we outline prominent models, highlight possible pitfalls discuss future directions. We foresee accelerating changes area genomics, especially for multi-scale multimodal data analysis precision medicine.
Language: Английский
Citations
142Computational and Structural Biotechnology Journal, Journal Year: 2021, Volume and Issue: 19, P. 4003 - 4017
Published: Jan. 1, 2021
Resistance to therapy remains a major cause of cancer treatment failures, resulting in many cancer-related deaths. can occur at any time during the treatment, even beginning. The current plan is dependent mainly on subtypes and presence genetic mutations. Evidently, mutation does not always predict therapeutic response vary for different subtypes. Therefore, there an unmet need predictive models match patient with specific drug or combination. Recent advancements using artificial intelligence have shown great promise preclinical settings. However, despite massive improvements computational power, building clinically useable challenging due lack meaningful pharmacogenomic data. In this review, we provide overview recent prediction machine learning, which most widely used branch intelligence. We describe basics learning algorithms, illustrate their use, highlight challenges clinical practice.
Language: Английский
Citations
121