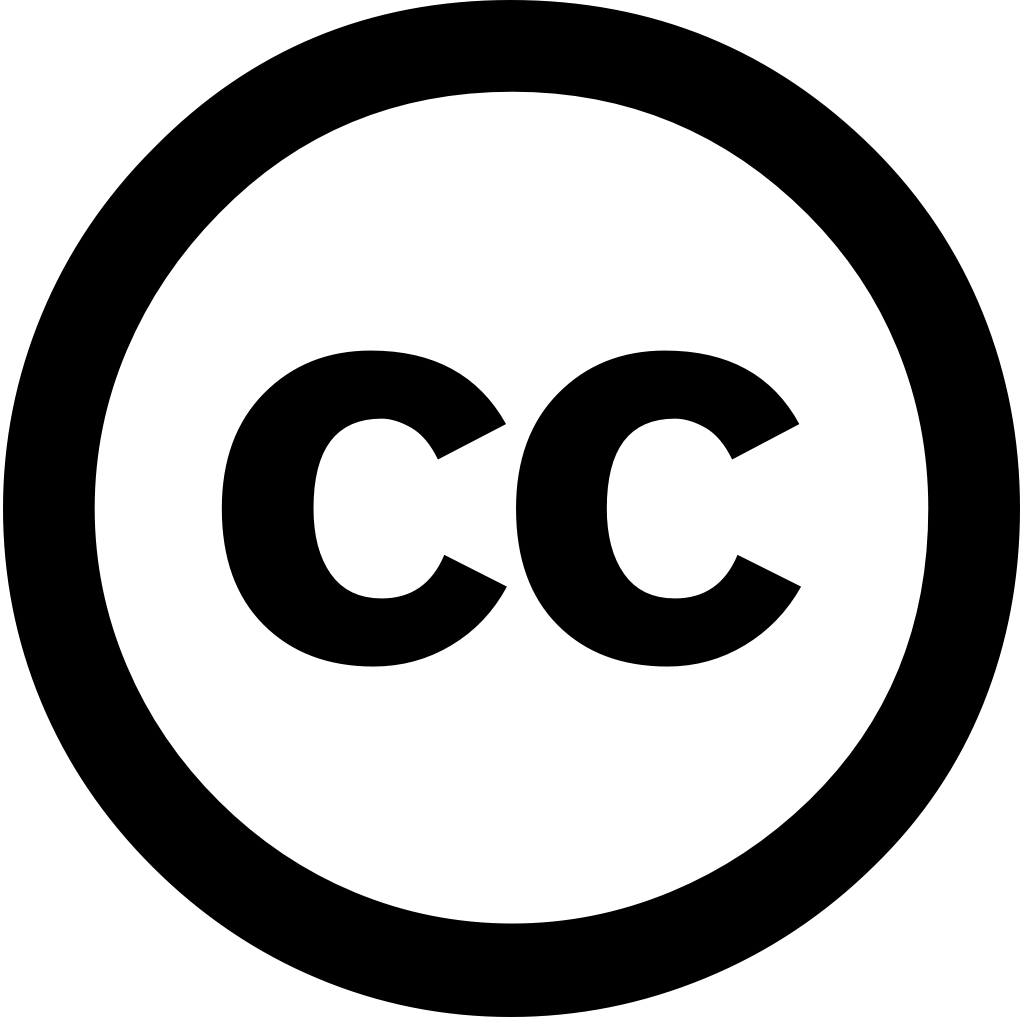
BMC Gastroenterology, Journal Year: 2025, Volume and Issue: 25(1)
Published: April 18, 2025
Language: Английский
BMC Gastroenterology, Journal Year: 2025, Volume and Issue: 25(1)
Published: April 18, 2025
Language: Английский
Frontiers in Oncology, Journal Year: 2025, Volume and Issue: 14
Published: Jan. 7, 2025
Colorectal cancer is one of the leading causes cancer-related mortality in world. Incidence and are predicted to rise globally during next several decades. When detected early, colorectal treatable with surgery medications. This leads requirement for prognostic diagnostic biomarker development. Our study integrates machine learning models protein network analysis identify biomarkers cancer. methodology leverages an extensive collection proteome profiles from both healthy individuals. To a potential high predictive ability, we used three models. enhance interpretability our models, quantify each protein's contribution model's predictions using SHapley Additive exPlanations values. Three classifiers-LASSO, XGBoost, LightGBM were evaluated performance along hyperparameter tuning model grid search, LASSO achieving highest AUC 75% UK Biobank dataset AUCs XGBoost 69.61% 71.42%, respectively. Using values, TFF3, LCN2, CEACAM5 found be key associated cell adhesion inflammation. Protein quantitative trait loci analyze studies provided further evidence involvement TFF1, CEACAM5, SELE cancer, possible connections PI3K/Akt MAPK signaling pathways. By offering insights into diagnostics targeted therapeutics, findings set stage validation.
Language: Английский
Citations
2Electronics, Journal Year: 2025, Volume and Issue: 14(5), P. 880 - 880
Published: Feb. 23, 2025
Colorectal cancer (CRC) has a relatively high five-year survival rate compared to other cancers; however, this drops significantly in patients with malignant CRC. One critical factor palliative care decision-making is the ability accurately predict patient survival, six-month period commonly used as threshold. In study, we evaluated performance of five machine learning models—logistic regression, decision tree, random forest, multilayer perceptron, and extreme gradient boosting (XGBoost)—in predicting for CRC using publicly available synthetic dataset containing 11,774 samples 51 features. The models were trained validated five-fold cross-validation, minority oversampling technique (SMOTE) was applied address class imbalance. Among models, XGBoost demonstrated highest performance, achieving 95% accuracy, precision, recall, F1-score, along 90% specificity. Feature importance analysis identified smoking status surgical history key factors influencing model predictions. These findings highlight potential tree-based supporting timely informed decisions, while also providing insights into handling data imbalance optimizing parameters prediction tasks.
Language: Английский
Citations
1Cancers, Journal Year: 2024, Volume and Issue: 16(24), P. 4128 - 4128
Published: Dec. 10, 2024
Modern technologies, particularly artificial intelligence methods such as machine learning, hold immense potential for supporting doctors with cancer diagnostics. This study explores the enhancement of popular learning using a bio-inspired algorithm—the naked mole-rat algorithm (NMRA)—to assess malignancy thyroid tumors. The utilized novel dataset released in 2022, containing data collected at Shengjing Hospital China Medical University. comprises 1232 records described by 19 features. In this research, 10 well-known classifiers, including XGBoost, LightGBM, and random forest, were employed to evaluate A key innovation is application parameter optimization feature selection within individual classifiers. Among models tested, LightGBM classifier demonstrated highest performance, achieving classification accuracy 81.82% an F1-score 86.62%, following two-level algorithm. Additionally, explainability analysis model was conducted SHAP values, providing insights into decision-making process model.
Language: Английский
Citations
5Published: Jan. 1, 2025
Language: Английский
Citations
0Research Square (Research Square), Journal Year: 2025, Volume and Issue: unknown
Published: Feb. 6, 2025
Language: Английский
Citations
0Current Oncology, Journal Year: 2025, Volume and Issue: 32(2), P. 117 - 117
Published: Feb. 18, 2025
Colorectal cancer liver metastasis (CRLM) is a significant contributor to cancer-related illness and death. Neoadjuvant chemotherapy (NAC) an essential treatment approach; however, optimal patient selection remains challenge. This study aimed develop machine learning-based predictive model using hematological biomarkers assess the efficacy of NAC in patients with CRLM. We retrospectively analyzed clinical data 214 CRLM treated XELOX regimen. Blood characteristics before after NAC, as well ratios these biomarkers, were integrated into learning models. Logistic regression, decision trees (DTs), random forest (RF), support vector (SVM), AdaBoost used for modeling. The performance models was evaluated AUROC, F1-score, external validation. DT (AUROC: 0.915, F1-score: 0.621) RF 0.999, 0.857) demonstrated best training cohort. incorporating ratio post-treatment pre-treatment gamma-glutamyl transferase (rGGT) carcinoembryonic antigen (rCEA) formed GCR index, which achieved AUROC 0.853 index showed strong relevance, predicting better responses lower rCEA higher rGGT levels. serves biomarker CRLM, providing valuable reference prognostic assessment patients.
Language: Английский
Citations
0PubMed, Journal Year: 2025, Volume and Issue: 18(1), P. 29 - 29
Published: April 11, 2025
Language: Английский
Citations
0SLAS TECHNOLOGY, Journal Year: 2025, Volume and Issue: 32, P. 100295 - 100295
Published: April 17, 2025
Colorectal cancer (CRC) is one of the most prominent cancers globally, with its incidence rising among younger adults due to improved screening practices. However, existing algorithms for CRC prediction are frequently trained on datasets that primarily reflect older persons, thus limiting their usefulness in more diverse populations. Additionally, part nutrition deterrence and management gaining significant attention, although computational approaches analyzing impact diet remain underdeveloped. This research introduces Nutritional Impact Prediction Framework (NICRP-Framework), which combines Natural Language Processing (NLP) techniques Adaptive Tunicate Swarm Optimized Large Models (ATSO-LLMs) present important insights into care across The colorectal dietary lifestyle dataset, encompassing >1000 participants, collected from multiple regions sources. dataset includes structured unstructured data, including textual descriptions food ingredients. These processed using standardization techniques, such as stop word removal, lowercasing, punctuation elimination. Relevant terms then extracted visualized a cloud. also contained an imbalanced binary outcome, rebalanced utilizing random oversampling. ATSO-LLMs employed analyze identifying key nutritional factors forecasting non-CRC phenotypes based patterns. results show combining NLP-derived features significantly enhances accuracy (98.4 %), sensitivity (97.6 %) specificity (96.9 F1-Score (96.2 minimal misclassification rates. framework represents transformative advancement life science by offering new, data-driven approach understanding determinants CRC, empowering healthcare professionals make precise predictions adapted interventions
Language: Английский
Citations
0BMC Gastroenterology, Journal Year: 2025, Volume and Issue: 25(1)
Published: April 18, 2025
Language: Английский
Citations
0