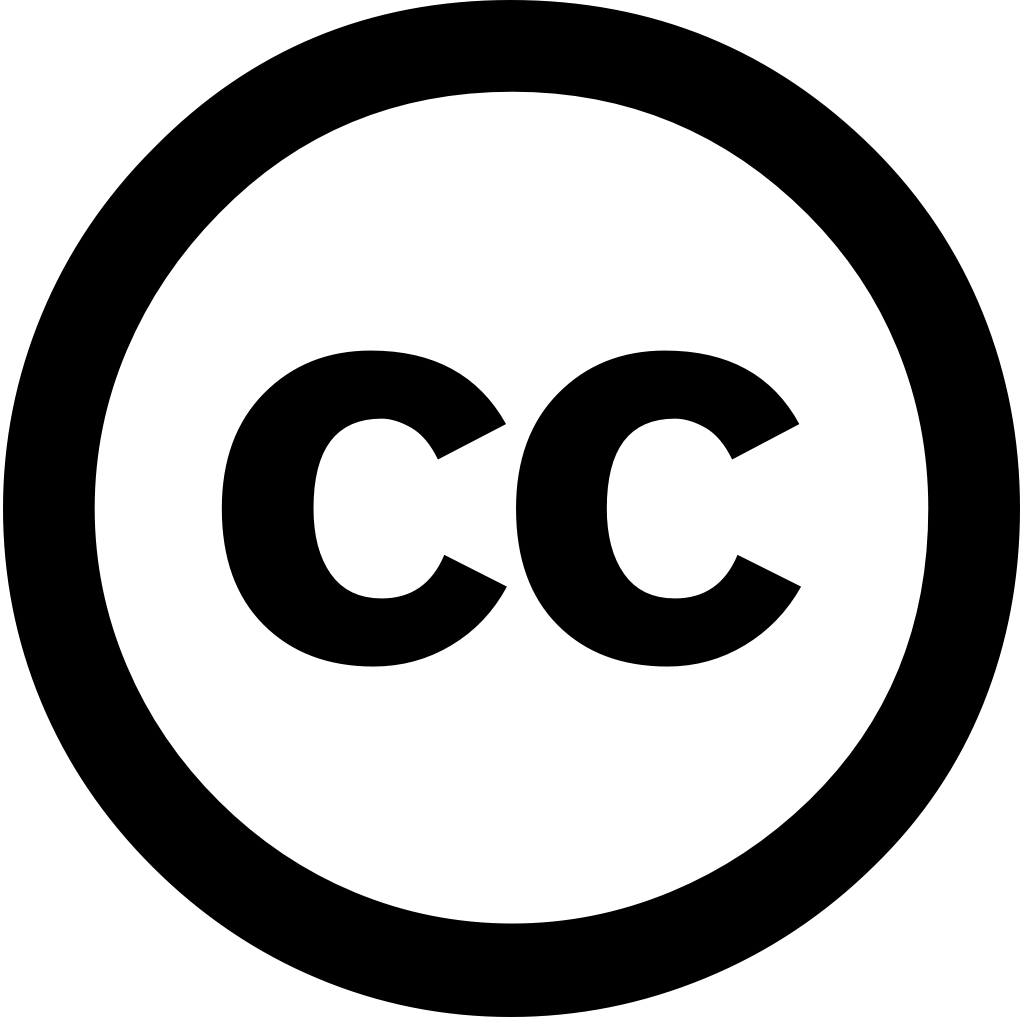
Heliyon, Journal Year: 2024, Volume and Issue: 10(18), P. e37916 - e37916
Published: Sept. 1, 2024
Language: Английский
Heliyon, Journal Year: 2024, Volume and Issue: 10(18), P. e37916 - e37916
Published: Sept. 1, 2024
Language: Английский
Remote Sensing, Journal Year: 2023, Volume and Issue: 15(14), P. 3534 - 3534
Published: July 13, 2023
This study provides a comprehensive review of the efforts utilized in measurement water quality parameters (WQPs) with focus on total dissolved solids (TDS) and suspended (TSS). The current method used TDS TSS includes conventional field gravimetric approaches. These methods are limited due to associated cost labor, spatial coverages. Remote Sensing (RS) applications have, however, been over past few decades as an alternative overcome these limitations. Although they also present underlying atmospheric interferences images, radiometric spectral resolution issues. Studies WQPs RS, therefore, require knowledge utilization best mechanisms. use RS for retrieval TDS, TSS, their forms has explored many studies using images from airborne sensors onboard unmanned aerial vehicles (UAVs) satellite such those Landsat, Sentinel-2, Aqua, Terra platforms. properties serve inputs deep learning analysis statistical, machine models. Methods retrieve WQP measurements dependent optical inland bodies. While is optically active parameter, inactive low signal–noise ratio. detection visible, near-infrared, infrared bands some process that (usually) co-occurs changes affecting active. revealed significant improvements incorporating approaches estimating WQPs. findings reveal improved spatiotemporal potential effectively detect For effective monitoring we recommend employing correction mechanisms reduce image interference, exploration fusion microwave bands, high-resolution hyperspectral ML models, calibration validation observed data measured methods. Further could development new technology UAVs produce real-time situ TSS. presented this aid consolidating understanding advancement single repository thereby offering stakeholders, researchers, decision-makers, regulatory bodies go-to information resource enhance mitigation impairments.
Language: Английский
Citations
109International Journal of Computational and Experimental Science and Engineering, Journal Year: 2025, Volume and Issue: 11(1)
Published: Feb. 3, 2025
Depletion of dissolved oxygen in the water is a serious threat to fish and other aquatic organisms, it causes aerobic stress disease fish. Detection crucial maintain better growth spawning fishes. Recently many studies proposed deep learning-based quality analysis techniques, but these techniques inadequate handling complex data. Because has both spatial temporal characteristics, this makes most learning models inadequate. To handle such multifaceted data we ConvRec, architecture that incorporates CNN (Convolution neural network) LSTM (Long-short term structures. component extracts feature domain from different locations while captures features hence model can learn correlations between movement parameters classify aqua ponds. In work use two dataset are unlabelled collected using IoT (Internet things) devices. ConvRec model, usus fine-grained annotation points have effect empowering detect relevant traits associated with It be therefore ascertained yields high degrees accuracy 99.2% 99.65%, on “ponds” “waterx” datasets respectively past only 98.2% 98.1% same datasets. These results demonstrate not promising for estimating health during deficiency also take part reducing negative impact low levels
Language: Английский
Citations
3Applied Sciences, Journal Year: 2023, Volume and Issue: 13(17), P. 9588 - 9588
Published: Aug. 24, 2023
Several sectors have embraced Cloud Computing (CC) due to its inherent characteristics, such as scalability and flexibility. However, despite these advantages, security concerns remain a significant challenge for cloud providers. CC introduces new vulnerabilities, including unauthorized access, data breaches, insider threats. The shared infrastructure of systems makes them attractive targets attackers. integration robust mechanisms becomes crucial address challenges. One mechanism is an Intrusion Detection System (IDS), which fundamental in safeguarding networks environments. An IDS monitors network traffic system activities. In recent years, researchers explored the use Machine Learning (ML) Deep (DL) approaches enhance performance IDS. ML DL algorithms demonstrated their ability analyze large volumes make accurate predictions. By leveraging techniques, IDSs can adapt evolving threats, detect previous attacks, reduce false positives. This article proposes novel model based on like Radial Basis Function Neural Network (RBFNN) Random Forest (RF). RF classifier used feature selection, RBFNN algorithm intrusion Moreover, datasets Bot-IoT NSL-KDD been utilized validate our suggested approach. To evaluate impact approach imbalanced dataset, we relied Matthew’s Correlation Coefficient (MCC) normalized measure. Our method achieves accuracy (ACC) higher than 92% using minimum features, managed increase MCC from 28% 93%. contributions this study are twofold. Firstly, it presents that leverages algorithms, demonstrating improved ACC minimal features substantial Secondly, addresses challenges specific environments, offering promising solution systems. integrating proposed into providers benefit enhanced measures, effectively mitigating access potential breaches. utilization RBFNN, has shown remarkable detecting intrusions strengthening overall posture CC.
Language: Английский
Citations
31Environmental and Sustainability Indicators, Journal Year: 2025, Volume and Issue: unknown, P. 100620 - 100620
Published: Jan. 1, 2025
Language: Английский
Citations
1Water, Journal Year: 2023, Volume and Issue: 15(13), P. 2439 - 2439
Published: July 2, 2023
Total dissolved solids (TDS) concentration determination in water bodies is sophisticated, time-consuming, and involves expensive field sampling laboratory processes. TDS has, however, been linked to electrical conductivity (EC) temperature. Compared monitoring concentrations, EC temperature simpler, inexpensive, takes less time. This study, therefore, applied several machine learning (ML) approaches estimate Lake Mead using data. Standalone models including the support vector (SVM), linear regressors (LR), K-nearest neighbor model (KNN), artificial neural network (ANN), ensemble such as bagging, gradient boosting (GBM), extreme (XGBoost), random forest (RF), extra trees (ET) were used this study. The models’ performance evaluated metrics aimed at providing a holistic assessment of each model. Metrics include coefficient (R2), mean absolute error (MAE), percent relative (PMARE), root square (RMSE), scatter index (SI), Nash–Sutcliffe efficiency (NSE) coefficient, bias (PBIAS). Results obtained showed varying training, testing, external validation stage models, with R2 0.77–1.00, RMSE 2.28–37.68 mg/L, an MAE 0.14–22.67 PMARE 0.02–3.42%, SI 0.00–0.06, NSE PBIAS 0.30–0.97 across all for three datasets. We utilized rankings assess found LR be best-performing on datasets among (R2 0.82 33.09 mg/L), possibly due established existence relationship between EC, although may not always linear. Similarly, we XGBoost based 0.81 34.19 mg/L. Assessing overall datasets, revealed GBM produce superior ranks, its ability reduce overfitting improve generalizations. findings from study could employed assisting resources managers stakeholders effective management ensure their sustainability.
Language: Английский
Citations
19Journal of Hydrology, Journal Year: 2024, Volume and Issue: 641, P. 131767 - 131767
Published: Aug. 3, 2024
Language: Английский
Citations
5Case Studies in Chemical and Environmental Engineering, Journal Year: 2024, Volume and Issue: 10, P. 100822 - 100822
Published: June 27, 2024
Monitoring river water quality is crucial for safeguarding public health, protecting ecosystems, and ensuring economic sustainability. It helps detect contaminants, ensures drinking safety, facilitates early intervention environmental protection legal compliance. The objective of this study to evaluate multiple machine learning algorithms analyze parameters in computing index (WQI) classification thereof, aiming devise a reliable method forecasting with high accuracy. In study, fourteen classifiers applied include Support Vector Machine (SVM), Random Forest (RF), Logistic Regression (LR), Decision Tree (DT), Multilayer Perceptron (MLP), K-Nearest Neighbor (KNN), Naïve Bayes, Gradient boosting, AdaBoost, Bagging, Extra Trees, Quadratic Discriminant Analysis (QDA), XGBoost, CATBoost. A total 1096 sample data was used where each consists nineteen analytical parameters. To assess the performance various classifiers, several evaluation techniques were utilized including confusion matrices, reports detailing precision accuracy ratios, Receiver Operating Characteristic (ROC) curves. also utilizes explainable AI (LIME SHAP) provide clear insights into decision-making processes classify quality. results indicated that all ML models demonstrate satisfactory predicting WQI. Among used, Boosting achieves highest Accuracy (99.64 %), Precision (0.95), Recall (0.96), F1-Score indicating its superior ability correctly instances suggesting balanced across different metrics. analysis presented article holds promise providing accurate researchers, thereby enhancing monitoring effectiveness through application techniques.
Language: Английский
Citations
4Water science and technology library, Journal Year: 2025, Volume and Issue: unknown, P. 297 - 322
Published: Jan. 1, 2025
Language: Английский
Citations
0Environment Development and Sustainability, Journal Year: 2025, Volume and Issue: unknown
Published: Feb. 3, 2025
Language: Английский
Citations
0Elsevier eBooks, Journal Year: 2025, Volume and Issue: unknown, P. 89 - 109
Published: Jan. 1, 2025
Language: Английский
Citations
0