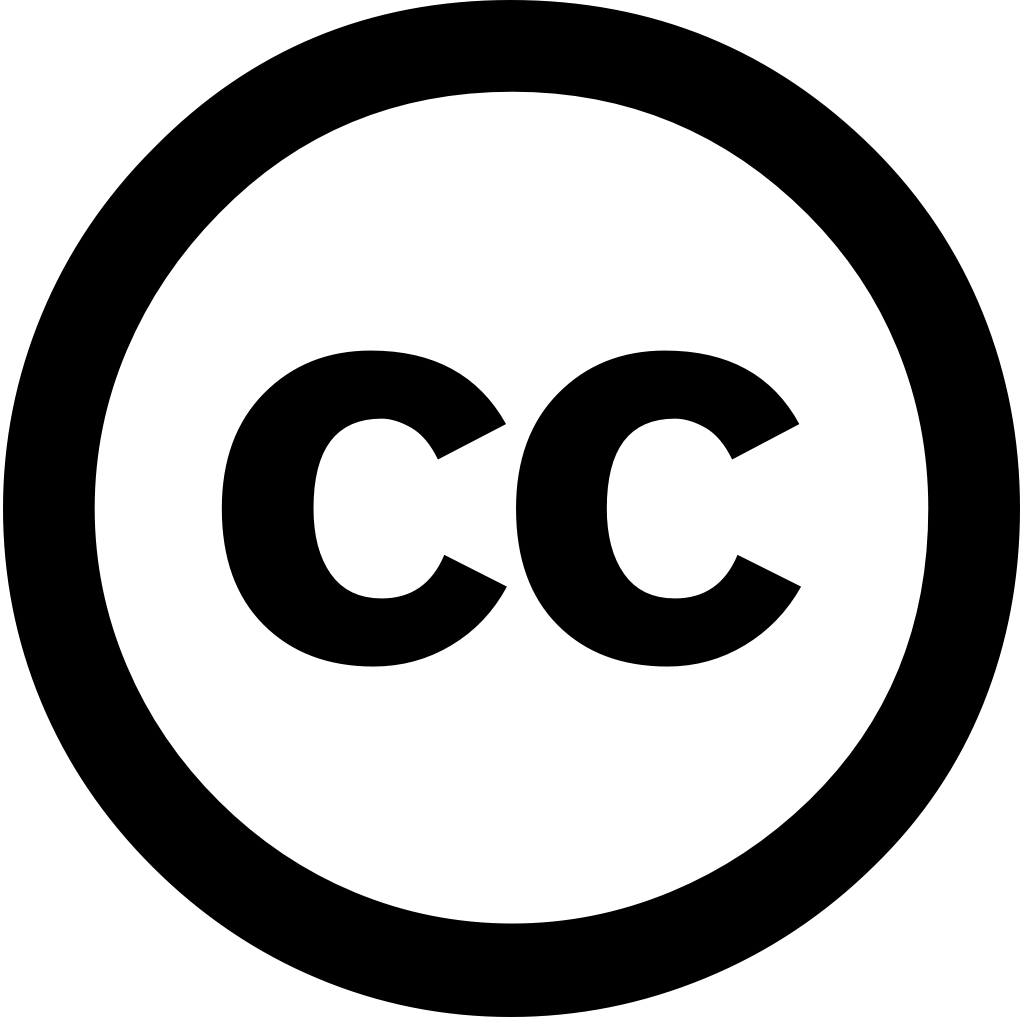
Frontiers in Artificial Intelligence, Journal Year: 2025, Volume and Issue: 8
Published: April 16, 2025
Accurate segmentation of anatomical structures in chest X-ray images remains challenging, especially for regions with low contrast and overlapping structures. This limitation significantly affects the diagnosis cardiothoracic diseases. Existing deep learning methods often struggle preserving structural boundaries, leading to artifacts. To address these challenges, I propose a novel approach that integrates contour detection techniques U-net architecture. Specifically, method employs Sobel Scharr edge filters enhance boundaries before segmentation. The pipeline involves pre-processing using detection, followed by model trained identify lungs, heart, clavicles. Experimental evaluation demonstrated edge-enhancing filters, particularly operator, leads marked improvement accuracy. For lung segmentation, achieved an accuracy 99.26%, Dice coefficient 98.88%, Jaccard index 97.54%. Heart results included 99.47% 94.14% index, while clavicle reached 99.79% 89.57% index. These consistently outperform baseline without enhancement. integration improves quality complex X-rays. Among tested operator proved be most effective enhancing boundary information reducing offers promising direction more accurate robust computer-aided systems radiology.
Language: Английский