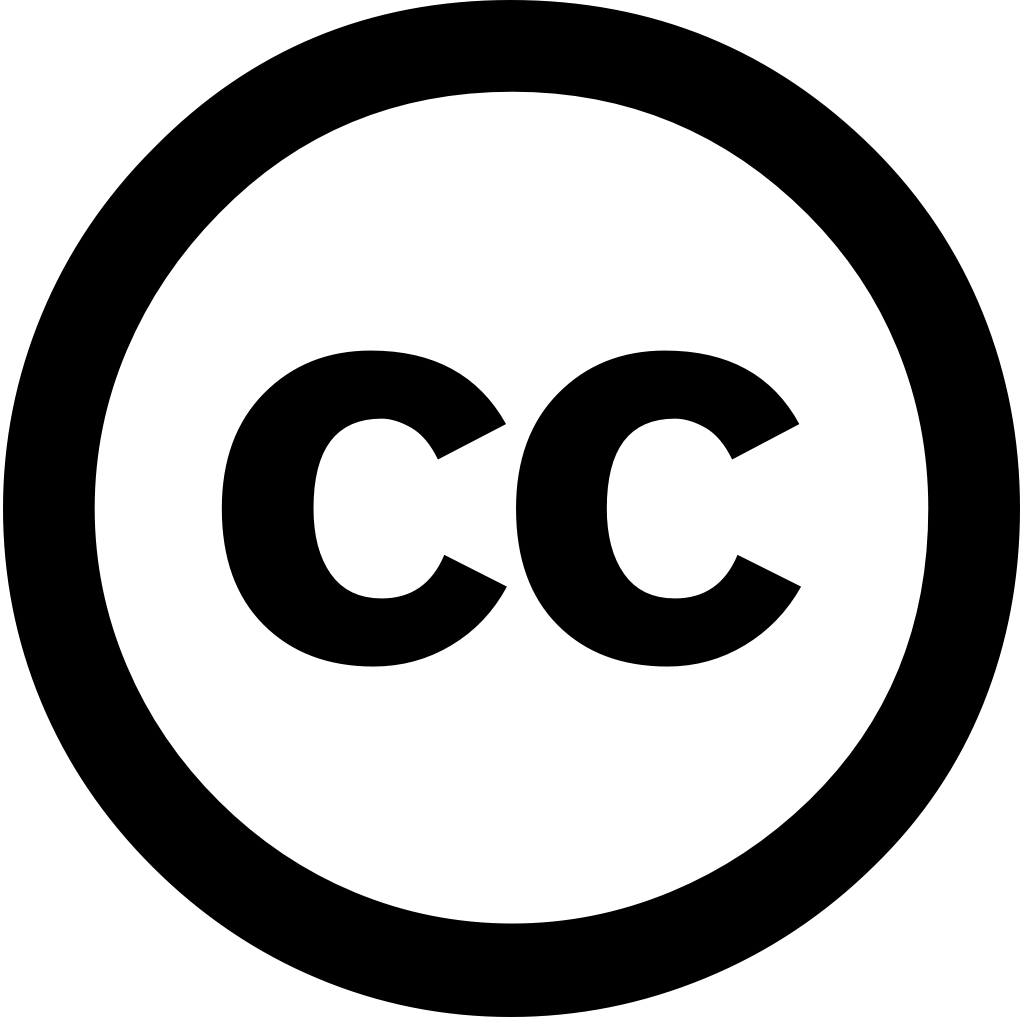
npj Precision Oncology, Journal Year: 2024, Volume and Issue: 8(1)
Published: Sept. 5, 2024
Language: Английский
npj Precision Oncology, Journal Year: 2024, Volume and Issue: 8(1)
Published: Sept. 5, 2024
Language: Английский
Computers in Biology and Medicine, Journal Year: 2023, Volume and Issue: 164, P. 107268 - 107268
Published: July 20, 2023
Language: Английский
Citations
59Diagnostics, Journal Year: 2023, Volume and Issue: 13(7), P. 1238 - 1238
Published: March 25, 2023
One of the most frequent cancers in women is breast cancer, and year 2022, approximately 287,850 new cases have been diagnosed. From them, 43,250 died from this cancer. An early diagnosis cancer can help to overcome mortality rate. However, manual using mammogram images not an easy process always requires expert person. Several AI-based techniques suggested literature. still, they are facing several challenges, such as similarities between non-cancer regions, irrelevant feature extraction, weak training models. In work, we proposed a automated computerized framework for classification. The improves contrast novel enhancement technique called haze-reduced local-global. enhanced later employed dataset augmentation. This step aimed at increasing diversity improving capability selected deep learning model. After that, pre-trained model named EfficientNet-b0 was fine-tuned add few layers. trained separately on original transfer concepts with static hyperparameters' initialization. Deep features were extracted average pooling layer next fused serial-based approach. optimized selection algorithm known Equilibrium-Jaya controlled Regula Falsi. Falsi termination function algorithm. finally classified machine classifiers. experimental conducted two publicly available datasets-CBIS-DDSM INbreast. For these datasets, achieved accuracy 95.4% 99.7%. A comparison state-of-the-art (SOTA) technology shows that obtained improved accuracy. Moreover, confidence interval-based analysis consistent results framework.
Language: Английский
Citations
55Diagnostics, Journal Year: 2024, Volume and Issue: 14(8), P. 848 - 848
Published: April 19, 2024
The rapid advancement of artificial intelligence (AI) has significantly impacted various aspects healthcare, particularly in the medical imaging field. This review focuses on recent developments application deep learning (DL) techniques to breast cancer imaging. DL models, a subset AI algorithms inspired by human brain architecture, have demonstrated remarkable success analyzing complex images, enhancing diagnostic precision, and streamlining workflows. models been applied diagnosis via mammography, ultrasonography, magnetic resonance Furthermore, DL-based radiomic approaches may play role risk assessment, prognosis prediction, therapeutic response monitoring. Nevertheless, several challenges limited widespread adoption clinical practice, emphasizing importance rigorous validation, interpretability, technical considerations when implementing solutions. By examining fundamental concepts synthesizing latest advancements trends, this narrative aims provide valuable up-to-date insights for radiologists seeking harness power care.
Language: Английский
Citations
31Journal of Applied Biomedicine, Journal Year: 2024, Volume and Issue: 44(1), P. 119 - 148
Published: Jan. 1, 2024
The second-leading cause of death for women is breast cancer. Consequently, a precise early diagnosis essential. With the rapid development artificial intelligence, computer-aided can efficiently assist radiologists in diagnosing problems. Mammography images, thermal and ultrasound images are three ways to diagnose paper will discuss some recent developments machine learning deep different cancer methods. components conventional methods image preprocessing, segmentation, feature extraction, classification. Deep includes convolutional neural networks, transfer learning, other Additionally, benefits drawbacks thoroughly contrasted. Finally, we also provide summary challenges potential futures diagnosis.
Language: Английский
Citations
17Visual Computing for Industry Biomedicine and Art, Journal Year: 2025, Volume and Issue: 8(1)
Published: Jan. 8, 2025
Abstract The vision transformer (ViT) architecture, with its attention mechanism based on multi-head layers, has been widely adopted in various computer-aided diagnosis tasks due to effectiveness processing medical image information. ViTs are notably recognized for their complex which requires high-performance GPUs or CPUs efficient model training and deployment real-world diagnostic devices. This renders them more intricate than convolutional neural networks (CNNs). difficulty is also challenging the context of histopathology analysis, where images both limited complex. In response these challenges, this study proposes a TokenMixer hybrid-architecture that combines strengths CNNs ViTs. hybrid architecture aims enhance feature extraction classification accuracy shorter time fewer parameters by minimizing number input patches employed during training, while incorporating tokenization using layers encoder process across all network fast accurate breast cancer tumor subtype classification. inspired ConvMixer TokenLearner models. First, dynamically generates spatial maps enabling from minimize used training. Second, extracts relevant regions selected patches, tokenizes improve extraction, trains tokenized an network. We evaluated BreakHis public dataset, comparing it ViT-based other state-of-the-art methods. Our approach achieved impressive results binary multi-classification subtypes magnification levels (40×, 100×, 200×, 400×). demonstrated accuracies 97.02% 93.29% multi-classification, decision times 391.71 1173.56 s, respectively. These highlight potential our deep ViT-CNN advancing histopathological images. source code accessible: https://github.com/abimouloud/TokenMixer .
Language: Английский
Citations
2European Journal of Radiology, Journal Year: 2024, Volume and Issue: 173, P. 111356 - 111356
Published: Feb. 5, 2024
Language: Английский
Citations
11Journal of Electrical Engineering and Technology, Journal Year: 2025, Volume and Issue: unknown
Published: March 19, 2025
Language: Английский
Citations
1Biomimetics, Journal Year: 2023, Volume and Issue: 8(3), P. 270 - 270
Published: June 26, 2023
Breast cancer is one of the most common cancers in women, with an estimated 287,850 new cases identified 2022. There were 43,250 female deaths attributed to this malignancy. The high death rate associated type can be reduced early detection. Nonetheless, a skilled professional always necessary manually diagnose malignancy from mammography images. Many researchers have proposed several approaches based on artificial intelligence. However, they still face obstacles, such as overlapping cancerous and noncancerous regions, extracting irrelevant features, inadequate training models. In paper, we developed novel computationally automated biological mechanism for categorizing breast cancer. Using optimization approach Advanced Al-Biruni Earth Radius (ABER) algorithm, boosting classification realized. stages framework include data augmentation, feature extraction using AlexNet transfer learning, optimized convolutional neural network (CNN). learning CNN improved accuracy when results are compared recent approaches. Two publicly available datasets utilized evaluate framework, average 97.95%. To ensure statistical significance difference between methodology, additional tests conducted, analysis variance (ANOVA) Wilcoxon, addition evaluating various metrics. these emphasized effectiveness methodology current methods.
Language: Английский
Citations
19Expert Systems with Applications, Journal Year: 2024, Volume and Issue: 252, P. 124113 - 124113
Published: May 1, 2024
Language: Английский
Citations
7Multimedia Tools and Applications, Journal Year: 2024, Volume and Issue: 83(39), P. 86833 - 86868
Published: July 3, 2024
Language: Английский
Citations
7