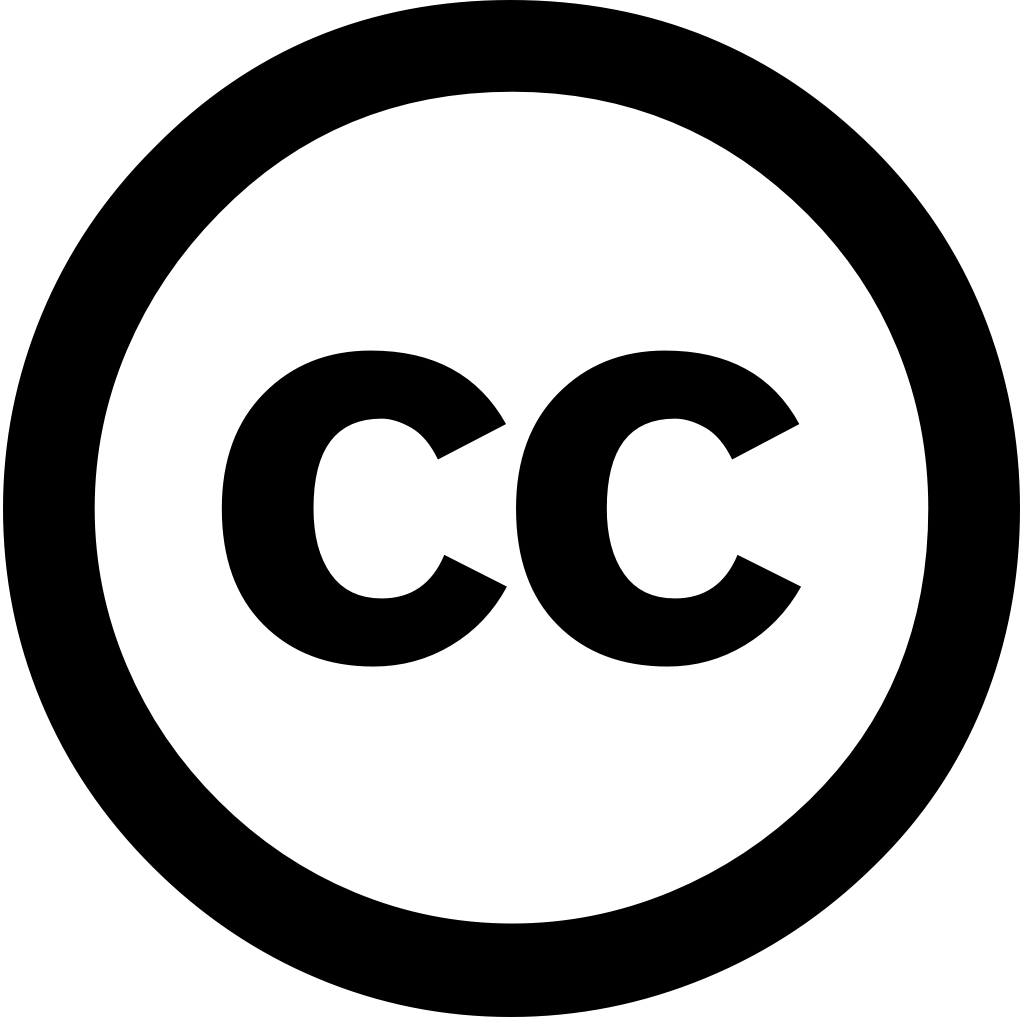
Biomedical Signal Processing and Control, Journal Year: 2024, Volume and Issue: 99, P. 106855 - 106855
Published: Sept. 12, 2024
Language: Английский
Biomedical Signal Processing and Control, Journal Year: 2024, Volume and Issue: 99, P. 106855 - 106855
Published: Sept. 12, 2024
Language: Английский
Scientific Reports, Journal Year: 2024, Volume and Issue: 14(1)
Published: Feb. 21, 2024
Abstract Skin cancer is a frequently occurring and possibly deadly disease that necessitates prompt precise diagnosis in order to ensure efficacious treatment. This paper introduces an innovative approach for accurately identifying skin by utilizing Convolution Neural Network architecture optimizing hyperparameters. The proposed aims increase the precision efficacy of recognition consequently enhance patients' experiences. investigation tackle various significant challenges recognition, encompassing feature extraction, model design, utilizes advanced deep-learning methodologies extract complex features patterns from images. We learning procedure deep integrating Standard U-Net Improved MobileNet-V3 with optimization techniques, allowing differentiate malignant benign cancers. Also substituted crossed-entropy loss function Mobilenet-v3 mathematical framework bias accuracy. model's squeeze excitation component was replaced practical channel attention achieve parameter reduction. Integrating cross-layer connections among Mobile modules has been leverage synthetic effectively. dilated convolutions were incorporated into receptive field. hyperparameters utmost importance improving efficiency models. To fine-tune hyperparameter, we employ sophisticated methods such as Bayesian method using pre-trained CNN MobileNet-V3. compared existing models, i.e., MobileNet, VGG-16, MobileNet-V2, Resnet-152v2 VGG-19 on “HAM-10000 Melanoma Cancer dataset". empirical findings illustrate optimized hybrid outperforms detection segmentation techniques based high 97.84%, sensitivity 96.35%, accuracy 98.86% specificity 97.32%. enhanced performance this research resulted timelier more diagnoses, potentially contributing life-saving outcomes mitigating healthcare expenditures.
Language: Английский
Citations
25Deleted Journal, Journal Year: 2024, Volume and Issue: unknown
Published: June 5, 2024
Abstract Skin cancer is one of the most frequently occurring cancers worldwide, and early detection crucial for effective treatment. Dermatologists often face challenges such as heavy data demands, potential human errors, strict time limits, which can negatively affect diagnostic outcomes. Deep learning–based systems offer quick, accurate testing enhanced research capabilities, providing significant support to dermatologists. In this study, we Swin Transformer architecture by implementing hybrid shifted window-based multi-head self-attention (HSW-MSA) in place conventional (SW-MSA). This adjustment enables model more efficiently process areas skin overlap, capture finer details, manage long-range dependencies, while maintaining memory usage computational efficiency during training. Additionally, study replaces standard multi-layer perceptron (MLP) with a SwiGLU-based MLP, an upgraded version gated linear unit (GLU) module, achieve higher accuracy, faster training speeds, better parameter efficiency. The modified model-base was evaluated using publicly accessible ISIC 2019 dataset eight classes compared against popular convolutional neural networks (CNNs) cutting-edge vision transformer (ViT) models. exhaustive assessment on unseen test dataset, proposed Swin-Base demonstrated exceptional performance, achieving accuracy 89.36%, recall 85.13%, precision 88.22%, F1-score 86.65%, surpassing all previously reported deep learning models documented literature.
Language: Английский
Citations
20Computers in Biology and Medicine, Journal Year: 2024, Volume and Issue: 178, P. 108798 - 108798
Published: June 25, 2024
Language: Английский
Citations
19Cluster Computing, Journal Year: 2024, Volume and Issue: unknown
Published: June 17, 2024
Abstract
Skin
cancer
is
one
of
the
most
dangerous
types
due
to
its
immediate
appearance
and
possibility
rapid
spread.
It
arises
from
uncontrollably
growing
cells,
rapidly
dividing
cells
in
area
body,
invading
other
bodily
tissues,
spreading
throughout
body.
Early
detection
helps
prevent
progress
reaching
critical
levels,
reducing
risk
complications
need
for
more
aggressive
treatment
options.
Convolutional
neural
networks
(CNNs)
revolutionize
skin
diagnosis
by
extracting
intricate
features
images,
enabling
an
accurate
classification
lesions.
Their
role
extends
early
detection,
providing
a
powerful
tool
dermatologists
identify
abnormalities
their
nascent
stages,
ultimately
improving
patient
outcomes.
This
study
proposes
novel
deep
convolutional
network
(DCNN)
approach
classifying
The
proposed
DCNN
model
evaluated
using
two
unbalanced
datasets,
namely
HAM10000
ISIC-2019.
compared
with
transfer
learning
models,
including
VGG16,
VGG19,
DenseNet121,
DenseNet201,
MobileNetV2.
Its
performance
assessed
four
widely
used
evaluation
metrics:
accuracy,
recall,
precision,
F1-score,
specificity,
AUC.
experimental
results
demonstrate
that
outperforms
(DL)
models
utilized
these
datasets.
achieved
highest
accuracy
ISIC-2019
$$98.5\%$$
Language: Английский
Citations
11Heliyon, Journal Year: 2024, Volume and Issue: 10(5), P. e26415 - e26415
Published: Feb. 18, 2024
Skin cancer is a prevalent form of that necessitates prompt and precise detection. However, current diagnostic methods for skin are either invasive, time-consuming, or unreliable. Consequently, there demand an innovative efficient approach to diagnose utilizes non-invasive automated techniques. In this study, unique method has been proposed diagnosing by employing Xception neural network optimized using Boosted Dipper Throated Optimization (BDTO) algorithm. The deep learning model capable extracting high-level features from dermoscopy images, while the BDTO algorithm bio-inspired optimization technique can determine optimal parameters weights network. To enhance quality diversity ISIC dataset utilized, widely accepted benchmark system diagnosis, various image preprocessing data augmentation techniques were implemented. By comparing with several contemporary approaches, it demonstrated outperforms others in detecting cancer. achieves average precision 94.936%, accuracy 94.206%, recall 97.092% surpassing performance alternative methods. Additionally, 5-fold ROC curve error have presented validation showcase superiority robustness method.
Language: Английский
Citations
8Healthcare Analytics, Journal Year: 2024, Volume and Issue: 5, P. 100326 - 100326
Published: April 2, 2024
Skin cancer can be detected through visual examination and confirmed dermoscopic analysis various diagnostic tests. This is because observation enables early detection of unique skin images by artificial intelligence. Promising outcomes are shown several Convolution Neural Network (CNN)–based lesion classification systems that employ tagged images. study suggests a practical approach for identifying cancers using dermoscopy pictures, improving specialists' ability to distinguish benign from malignant tumors. The Swarm Intelligence (SI) used photographs locate lesions on the areas Region interest (ROI). Grasshopper Optimization technique produced best segmentation outcomes. Speed-Up Robust Features (SURF) applied extract features based these findings. Two groups were created ISIC-2017, ISIC-2018, PH-2 databases categorize With an estimated accuracy in 98.52%, preciseness 96.73%, Matthews Correlation Coefficient (MCC) 97.04%, suggested methodologies have been evaluated efficacy, specificity, sensitivity, F-measure, preciseness, MCC, dice coefficient, Jaccard's index. In every performance indicator, method we suggest outperformed state-of-the-art methods.
Language: Английский
Citations
5Sensors, Journal Year: 2025, Volume and Issue: 25(2), P. 394 - 394
Published: Jan. 10, 2025
Skin cancer accounts for over 40% of all diagnoses worldwide. However, accurately diagnosing skin remains challenging dermatologists, as multiple types often appear visually similar. The diagnostic accuracy dermatologists ranges between 62% and 80%. Although AI models have shown promise in assisting with classification various studies, obtaining the large-scale medical image datasets required model training is not straightforward. To address this limitation, study proposes AGS network, designed to overcome challenges small enhance performance classifiers. network integrates three key modules: Augmentation (A), GAN (G), Segmentation (S). It was evaluated using eight deep learning classifiers—GoogLeNet, DenseNet201, ResNet50, MobileNet V3, EfficientNet B0, ViT, V2, Swin Transformers—on HAM10000 dataset. Five configurations were also tested assess contribution each module. results showed that classifiers demonstrated consistent improvements network. In particular, V2 + achieved most significant gains baseline model, an increase +0.1808 Accuracy +0.1674 F1-Score. Among configurations, ResNet50+AGS best overall performance, 95.87% F1-Score 95.73%. While previous studies focused on single augmentation methods, demonstrates effectiveness combining techniques within integrated framework. how integrating diverse methods can improve models.
Language: Английский
Citations
0Digital Health, Journal Year: 2025, Volume and Issue: 11
Published: Jan. 1, 2025
To improve the accuracy and explainability of skin lesion detection classification, particularly for several types cancers, through a novel approach based on convolutional neural networks with attention-integrated customized ResNet variants (CRVs) an optimized ensemble learning (EL) strategy. Our utilizes all combined three attention mechanisms: channel attention, soft squeeze-excitation attention. These are aggregated unique multi-level EL We propose innovative weight optimization method, inverse Gini indexed averaging (IGIA), which is further extended to multi-leveled IGIA (ML-IGIA) determine optimal weights each model within multiple levels. For interpretability, we employ gradient class activation map highlight regions responsible classification dominance, enhancing model's transparency. method was evaluated Human Against Machines 10000 dataset, achieving superior 94.52% ML-IGIA approach, outperforming existing methods. The proposed CRV-based demonstrates robust performance in offering both high enhanced interpretability. This addresses current research gap effective supports timely, automated disease detection.
Language: Английский
Citations
0Lecture notes in computer science, Journal Year: 2025, Volume and Issue: unknown, P. 124 - 134
Published: Jan. 1, 2025
Language: Английский
Citations
0Communications in computer and information science, Journal Year: 2025, Volume and Issue: unknown, P. 26 - 37
Published: Jan. 1, 2025
Language: Английский
Citations
0