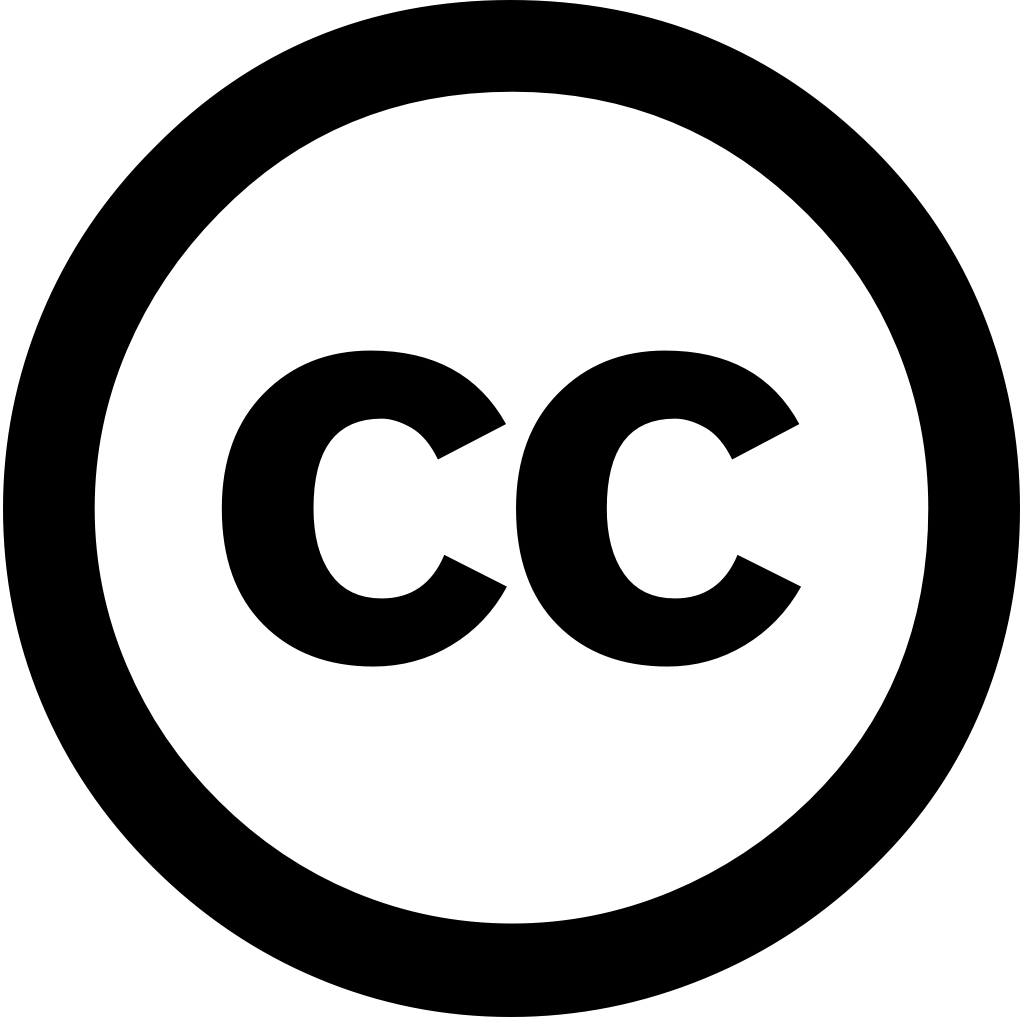
Clinical eHealth, Journal Year: 2024, Volume and Issue: unknown
Published: Dec. 1, 2024
Language: Английский
Clinical eHealth, Journal Year: 2024, Volume and Issue: unknown
Published: Dec. 1, 2024
Language: Английский
Applied Soft Computing, Journal Year: 2024, Volume and Issue: 156, P. 111491 - 111491
Published: March 11, 2024
Language: Английский
Citations
16Neural Computing and Applications, Journal Year: 2024, Volume and Issue: unknown
Published: Nov. 18, 2024
Language: Английский
Citations
10Progress in Artificial Intelligence, Journal Year: 2025, Volume and Issue: unknown
Published: Jan. 2, 2025
Language: Английский
Citations
1Electronics, Journal Year: 2024, Volume and Issue: 13(6), P. 1025 - 1025
Published: March 8, 2024
Machine learning is increasingly and ubiquitously being used in the medical domain. Evaluation metrics like accuracy, precision, recall may indicate performance of models but not necessarily reliability their outcomes. This paper assesses effectiveness a number machine algorithms applied to an important dataset domain, specifically, mental health, by employing explainability methodologies. Using multiple model techniques, this work provides insights into models’ workings help determine algorithm predictions. The results are intuitive. It was found that were focusing significantly on less relevant features and, at times, unsound ranking make therefore argues it for research provide addition other accuracy. particularly applications critical domains such as healthcare.
Language: Английский
Citations
4Frontiers in Medicine, Journal Year: 2024, Volume and Issue: 11
Published: July 5, 2024
Global Cardiovascular disease (CVD) is still one of the leading causes death and requires enhancement diagnostic methods for effective detection early signs prediction outcomes. The current tools are cumbersome imprecise especially with complex diseases, thus emphasizing incorporation new machine learning applications in differential diagnosis.
Language: Английский
Citations
4Computers and Electronics in Agriculture, Journal Year: 2024, Volume and Issue: 225, P. 109348 - 109348
Published: Aug. 22, 2024
Language: Английский
Citations
4Scientific Reports, Journal Year: 2025, Volume and Issue: 15(1)
Published: March 21, 2025
Accurately identifying bone fractures from the X-ray image is essential to prompt timely and appropriate medical treatment. This research explores impact of hyperparameters data augmentation techniques on performance You Only Look Once (YOLO) V10 architecture for fracture detection. While YOLO architectures have been widely employed in object detection tasks, recognizing fractures, which can appear as subtle complicated patterns images, requires rigorous model tuning. Image was done using unsharp masking approach contrast-limited adaptive histogram equalization before training model. The augmented images assist feature identification contribute overall current study has performed extensive experiments analyze influence like number epochs learning rate, along with analysis input data. experimental outcome proven that particular hyperparameter combinations, when paired targeted strategies, improve accuracy precision It observed proposed yielded an 0.964 evaluation over statistical classification across raw 0.98 0.95, respectively. In comparison other deep models, empirical clearly demonstrates its superior conventional approaches
Language: Английский
Citations
0Applied Computational Intelligence and Soft Computing, Journal Year: 2025, Volume and Issue: 2025(1)
Published: Jan. 1, 2025
Stroke disease has been the leading cause of death globally for last several decades. Thus, rate can be decreased by early recognition and ongoing surveillance. However, largest obstacle to perform advanced analytics using conventional approach is growth massive amount data from various sources, including patient histories, wearable sensor devices, medical data. The current technology that could have a large impact on healthcare sector integration machine learning with big (scalable learning), particularly in diagnosis this disease. To address issue, scalable stroke prediction model multinode distributed environment, which was developed combining concepts handle extensive datasets, an aspect not seen prior literature detection, presented work. We implemented four algorithms: logistic regression, random forest, gradient‐boosting tree, decision dataset collected Medical Quality Improvement Consortium database. As result, two worker nodes one master node were used analyze dataset. model’s performance assessed metrics area under curve (AUC) confusion matrix. With accuracy 94.3% AUC score 99%, forest determined better based experimental results. It also shown main risk factor diabetes, followed hypertension. This study demonstrated effectiveness Spark’s techniques forecast identify factors earlier. findings utilized physicians as clinical aids aid more accurate identification
Language: Английский
Citations
0BMC Medical Informatics and Decision Making, Journal Year: 2025, Volume and Issue: 25(1)
Published: Feb. 7, 2025
The relevance of the study is due to growing number diseases cerebrovascular system, in particular stroke, which one leading causes disability and mortality world. To improve stroke risk prediction models terms efficiency interpretability, we propose integrate modern machine learning algorithms data dimensionality reduction methods, XGBoost optimized principal component analysis (PCA), provide structuring increase processing speed, especially for large datasets. For first time, explainable artificial intelligence (XAI) integrated into PCA process, increases transparency interpretation, providing a better understanding factors medical professionals. proposed approach was tested on two datasets, with accuracy 95% 98%. Cross-validation yielded an average value 0.99, high values Matthew's correlation coefficient (MCC) metrics 0.96 Cohen's Kappa (CK) confirmed generalizability reliability model. speed increased threefold OpenMP parallelization, makes it possible apply practice. Thus, method innovative can potentially forecasting systems healthcare industry.
Language: Английский
Citations
0Studies in computational intelligence, Journal Year: 2025, Volume and Issue: unknown, P. 227 - 252
Published: Jan. 1, 2025
Language: Английский
Citations
0