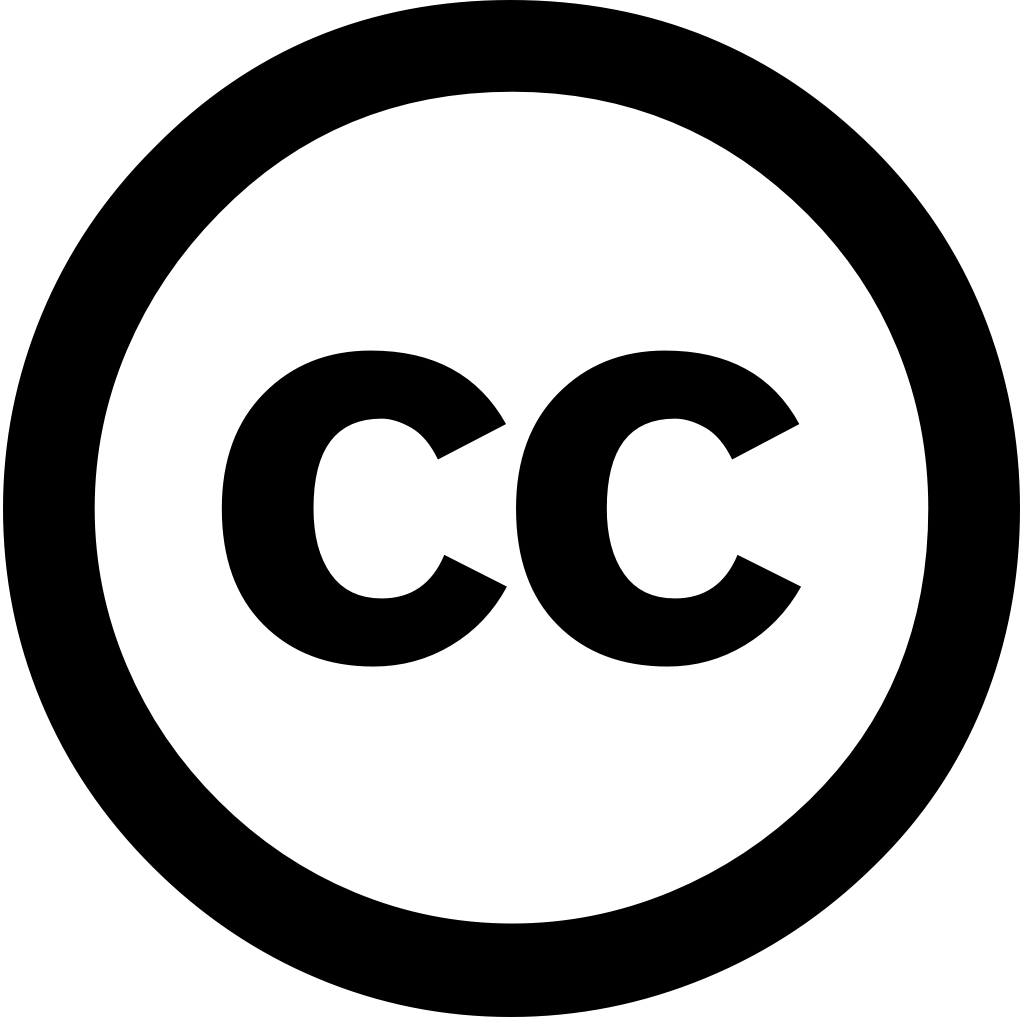
Clinical eHealth, Journal Year: 2024, Volume and Issue: unknown
Published: Dec. 1, 2024
Language: Английский
Clinical eHealth, Journal Year: 2024, Volume and Issue: unknown
Published: Dec. 1, 2024
Language: Английский
Studies in computational intelligence, Journal Year: 2025, Volume and Issue: unknown, P. 111 - 125
Published: Jan. 1, 2025
Language: Английский
Citations
0Lecture notes in networks and systems, Journal Year: 2025, Volume and Issue: unknown, P. 355 - 365
Published: Jan. 1, 2025
Language: Английский
Citations
0Journal of Ambient Intelligence and Humanized Computing, Journal Year: 2025, Volume and Issue: unknown
Published: April 26, 2025
Language: Английский
Citations
0Interdisciplinary Sciences Computational Life Sciences, Journal Year: 2025, Volume and Issue: unknown
Published: May 10, 2025
Language: Английский
Citations
0Procedia Computer Science, Journal Year: 2025, Volume and Issue: 259, P. 250 - 259
Published: Jan. 1, 2025
Language: Английский
Citations
0Eng—Advances in Engineering, Journal Year: 2024, Volume and Issue: 5(1), P. 282 - 300
Published: Feb. 5, 2024
This paper discusses a methodology to improve the prevention processes of chronic diseases such as diabetes and strokes. The research motivation is find new methodological approach design advanced Diagnostic Therapeutic Care Pathways (PDTAs) based on prediction disease using telemedicine technologies machine learning (ML) data processing techniques. aim decrease health risk avoid hospitalizations through prevention. proposed method defines Process Mining Organization (PMO) model, managing risks PDTA structured prevent risk. Specifically, analysis focused stroke First, we applied compared Random Forest (RF) Gradient Boosted Trees (GBT) supervised algorithms predict risk, then, Fuzzy c-Means unsupervised algorithm cluster information predicted results. application able increase efficiency healthcare human resources drastically care costs.
Language: Английский
Citations
2Published: March 4, 2024
Machine Learning is increasingly and ubiquitously being used in the medical domain. Evaluation metrics like accuracy, precision, recall may indicate performance of models but not necessarily reliability their outcomes. This paper assesses effectiveness a number machine learning algorithms applied to an important dataset domain, specifically, mental health, by employing explainability methodologies. Using multiple model techniques, project provides insights into workings help determine algorithm predictions. The results are intuitive. It was found that were focusing significantly on less relevant features at times, unsound ranking make therefore argues it for research provide addition other accuracy. particularly applications critical domains such as healthcare. A future direction investigate methods quantify terms from explainability.
Language: Английский
Citations
2International Journal of Information Technology, Journal Year: 2024, Volume and Issue: 16(7), P. 4489 - 4503
Published: July 5, 2024
Language: Английский
Citations
2Diagnostics, Journal Year: 2024, Volume and Issue: 14(22), P. 2514 - 2514
Published: Nov. 9, 2024
Background/Objectives: Stroke stands as a prominent global health issue, causing con-siderable mortality and debilitation. It arises when cerebral blood flow is compromised, leading to irreversible brain cell damage or death. Leveraging the power of machine learning, this paper presents systematic approach predict stroke patient survival based on comprehensive set factors. These factors include demographic attributes, medical history, lifestyle elements, physiological metrics. Method: An effective random sampling method proposed handle highly biased data stroke. The pre-diction using optimized boosting learning algorithms supported with explainable AI LIME SHAP. This enables models discern intricate patterns establish correlations between selected features survival. Results: performance three studied for prediction, which Gradient Boosting (GB), AdaBoost (ADB), XGBoost (XGB) XGB achieved best outcome overall training accuracy 96.97% testing 92.13%. Conclusions: Through approach, study seeks uncover actionable insights guide healthcare practitioners in devising personalized treatment strategies patients.
Language: Английский
Citations
2IEEE Access, Journal Year: 2024, Volume and Issue: 12, P. 168328 - 168338
Published: Jan. 1, 2024
Language: Английский
Citations
1