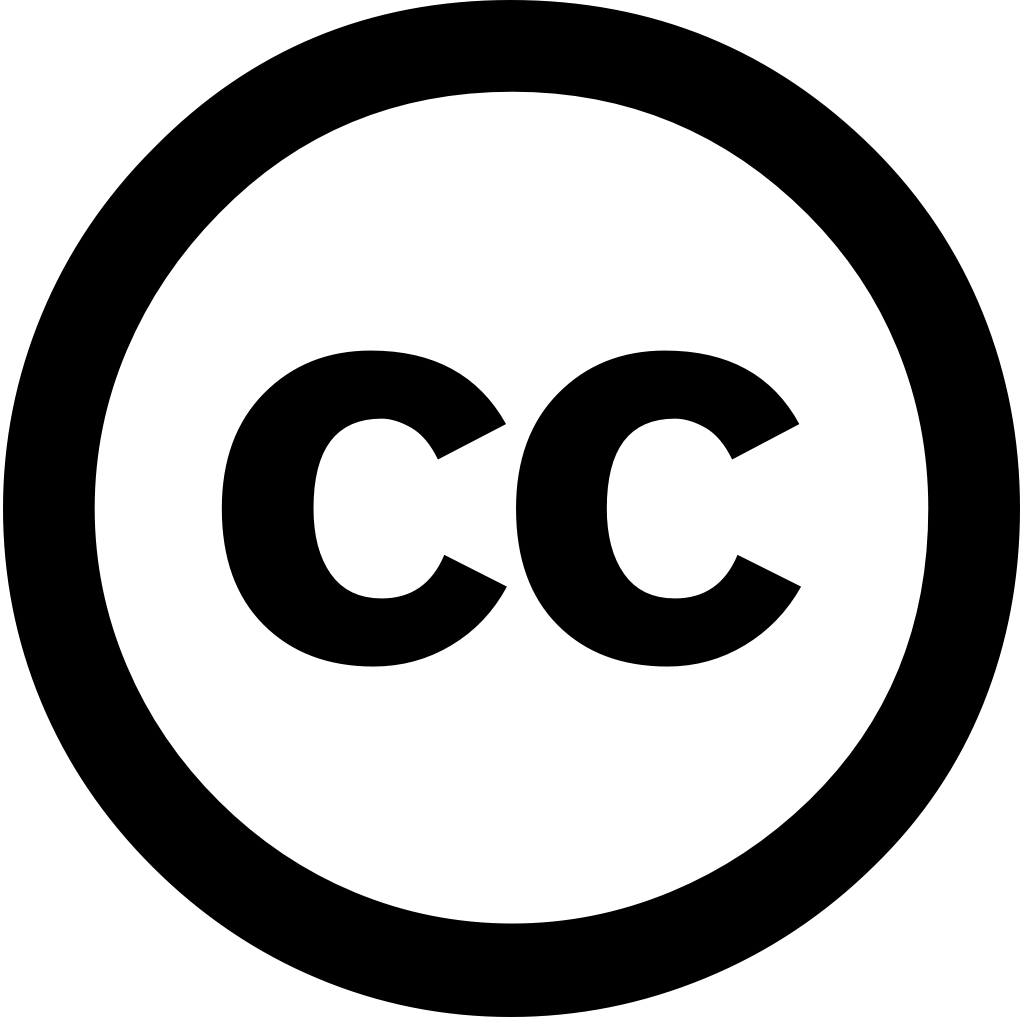
Computers in Biology and Medicine, Journal Year: 2024, Volume and Issue: 182, P. 109140 - 109140
Published: Sept. 12, 2024
Language: Английский
Computers in Biology and Medicine, Journal Year: 2024, Volume and Issue: 182, P. 109140 - 109140
Published: Sept. 12, 2024
Language: Английский
Frontiers in Immunology, Journal Year: 2025, Volume and Issue: 16
Published: April 16, 2025
This review highlights the critical role of radiologists in personalized cancer treatment, focusing on evaluation treatment outcomes using imaging tools like Computed Tomography (CT), Magnetic Resonance Imaging (MRI), and Ultrasound. Radiologists assess effectiveness complications therapies such as chemotherapy, immunotherapy, ablative treatments. Understanding mechanisms consistent protocols are essential for accurate evaluation, especially managing complex cases liver cancer. Collaboration between oncologists is key to optimizing patient through precise assessments.
Language: Английский
Citations
0World Journal of Gastrointestinal Oncology, Journal Year: 2024, Volume and Issue: 17(1)
Published: Dec. 11, 2024
BACKGROUND The liver, as the main target organ for hematogenous metastasis of colorectal cancer, early and accurate prediction liver is crucial diagnosis treatment patients. Herein, this study aims to investigate application value a combined machine learning (ML) based model on multiparameter magnetic resonance imaging rectal metachronous (MLM). AIM To efficacy radiomics multiparametric images preoperative first diagnosed cancer in predicting MLM from cancer. METHODS We retrospectively analyzed 301 patients with confirmed by surgical pathology at Jingzhou Central Hospital January 2017 December 2023. All participants were randomly assigned training or validation queue 7:3 ratio. apply generalized linear regression (GLRM) random forest (RFM) algorithm construct an queue, evaluate discriminative power using area under curve (AUC) decision analysis (DCA). Then, robustness generalizability evaluated internal set between groups. RESULTS Among included study, 16.28% ultimately through pathological examination. Multivariate showed that carcinoembryonic antigen, independent predictors MLM. GLRM was developed comprehensive nomogram achieve satisfactory differentiation. performance 0.765 [95% confidence interval (CI): 0.710-0.820] 0.767 (95%CI: 0.712-0.822), respectively. Compared GLRM, RFM achieved superior AUC 0.919 0.868-0.970) 0.901 0.850-0.952) DCA indicated predictive ability net profit clinical improved. CONCLUSION By combining effectiveness ML-based models, proposed can serve insight tool assessment risk stratification provide important information individual
Language: Английский
Citations
3Computers in Biology and Medicine, Journal Year: 2024, Volume and Issue: 182, P. 109140 - 109140
Published: Sept. 12, 2024
Language: Английский
Citations
2