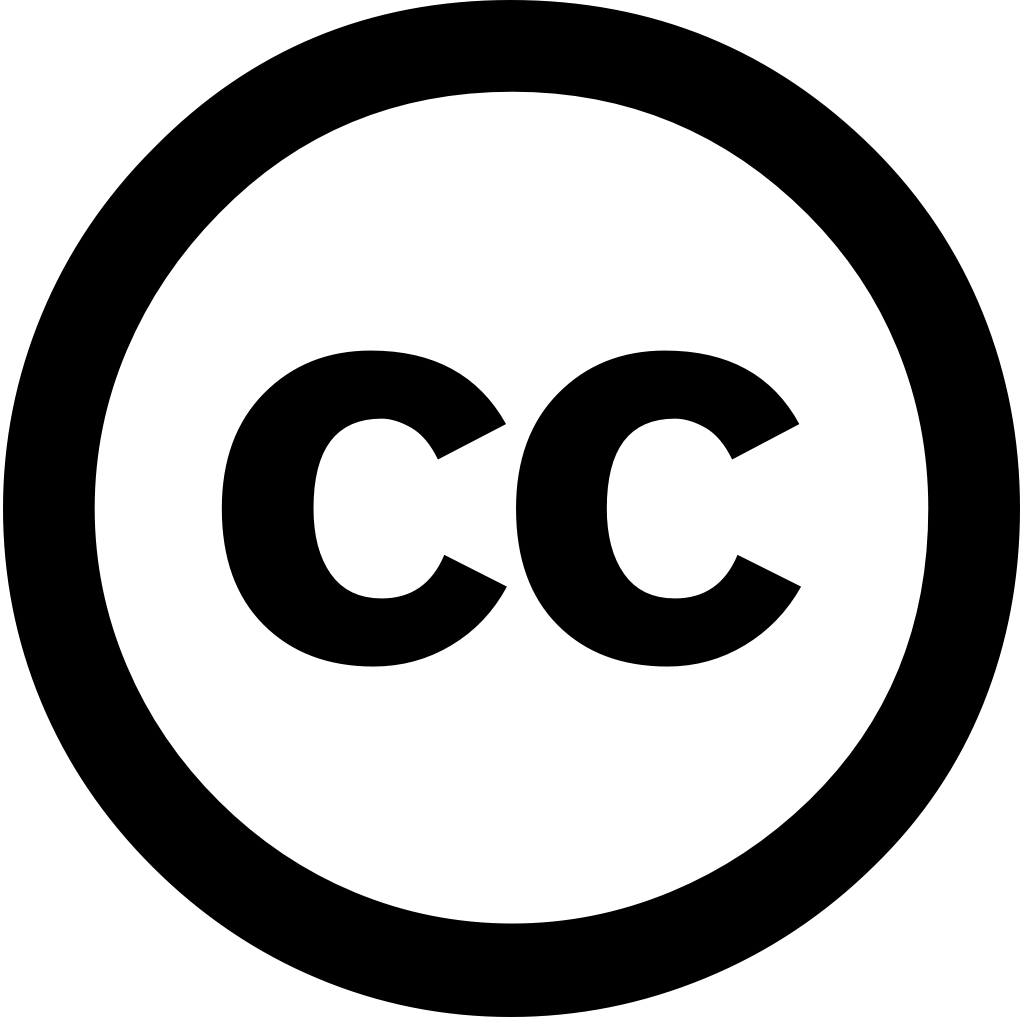
Scientific Reports, Journal Year: 2025, Volume and Issue: 15(1)
Published: May 15, 2025
Language: Английский
Scientific Reports, Journal Year: 2025, Volume and Issue: 15(1)
Published: May 15, 2025
Language: Английский
Diagnostics, Journal Year: 2025, Volume and Issue: 15(3), P. 363 - 363
Published: Feb. 4, 2025
Background\Objectives: Solving the secrets of brain is a significant challenge for researchers. This work aims to contribute this area by presenting new explainable feature engineering (XFE) architecture designed obtain results related stress and mental performance using electroencephalography (EEG) signals. Materials Methods: Two EEG datasets were collected detect stress. To achieve classification results, XFE model was developed, incorporating novel extraction function called Cubic Pattern (CubicPat), which generates three-dimensional vector coding channels. Classification obtained cumulative weighted iterative neighborhood component analysis (CWINCA) selector t-algorithm-based k-nearest neighbors (tkNN) classifier. Additionally, generated CWINCA Directed Lobish (DLob). Results: The CubicPat-based demonstrated both interpretability. Using 10-fold cross-validation (CV) leave-one-subject-out (LOSO) CV, introduced CubicPat-driven achieved over 95% 75% accuracies, respectively, datasets. Conclusions: interpretable deploying DLob statistical analysis.
Language: Английский
Citations
1Scientific Reports, Journal Year: 2025, Volume and Issue: 15(1)
Published: May 1, 2025
This paper presents a novel, explainable feature engineering framework for classifying EEG and ECG signals with high accuracy. The proposed method employs the Order Transition Pattern (OTPat) extractor. presented OTPat extractor captures both channel/column-based patterns (spatial features) using all channels each point signal/row-based (temporal by extracting features from individual overlapping blocks. extracted are then refined cumulative weighted iterative neighborhood component analysis (CWINCA) selection classified t‑algorithm k‑nearest neighbors (tkNN) classifier. Finally, two symbolic languages, Directed Lobish (DLob) Cardioish, generate interpretable results in form of cortical cardiac connectome diagrams. OTPat-based XFE model achieves over 95% accuracy on several datasets reaches 86.07% an 8‑class artifact dataset. These demonstrate performance clear interpretability, highlighting model's potential robust biomedical signal classification.
Language: Английский
Citations
0Scientific Reports, Journal Year: 2025, Volume and Issue: 15(1)
Published: May 15, 2025
Language: Английский
Citations
0