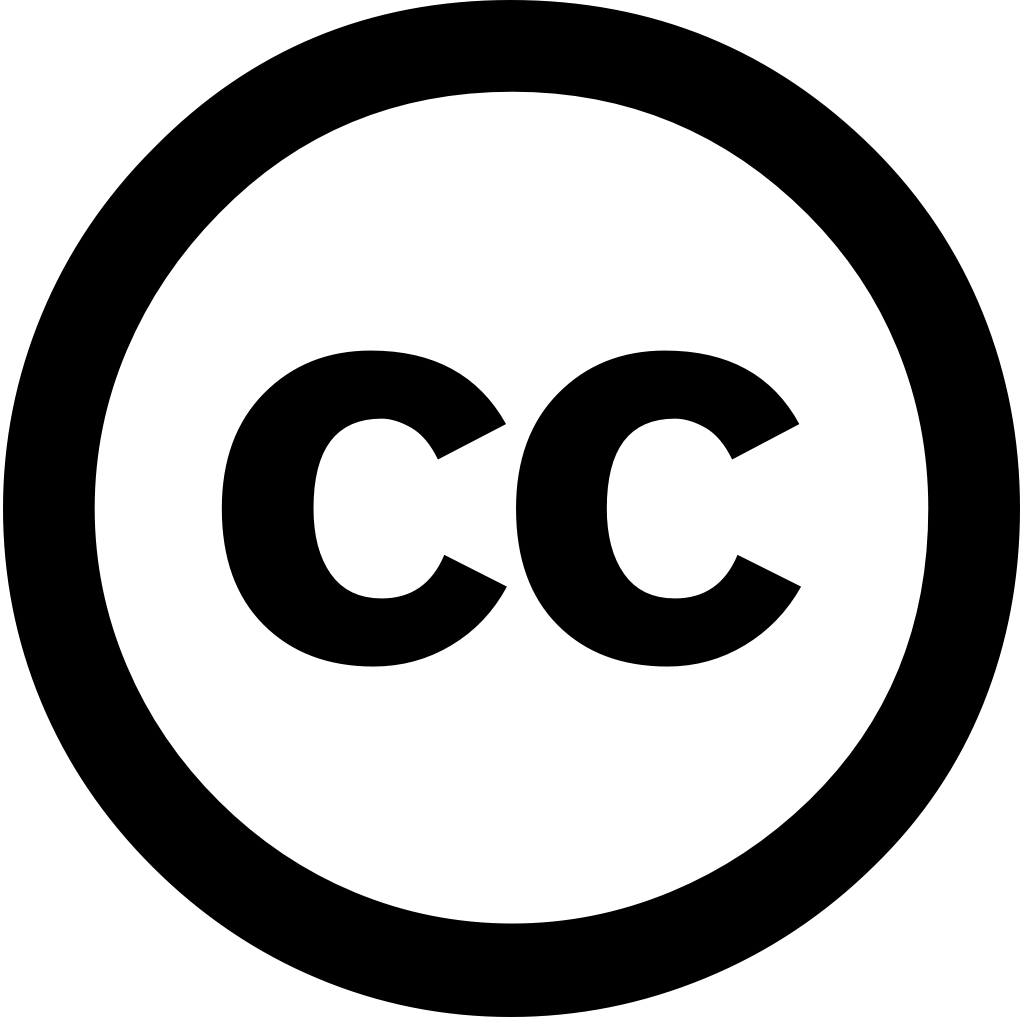
PeerJ Computer Science, Journal Year: 2025, Volume and Issue: 11, P. e2795 - e2795
Published: April 15, 2025
This study presents an augmented hybrid approach for improving the diagnosis of malignant skin lesions by combining convolutional neural network (CNN) predictions with selective human interventions based on prediction confidence. The algorithm retains high-confidence CNN while replacing low-confidence outputs expert assessments to enhance diagnostic accuracy. A model utilizing EfficientNetB3 backbone is trained datasets from ISIC-2019 and ISIC-2020 SIIM-ISIC melanoma classification challenges evaluated a 150-image test set. model’s are compared against 69 experienced medical professionals. Performance assessed using receiver operating characteristic (ROC) curves area under curve (AUC) metrics, alongside analysis resource costs. baseline achieves AUC 0.822, slightly below performance experts. However, improves true positive rate 0.782 reduces false 0.182, delivering better minimal involvement. offers scalable, resource-efficient solution address variability in image analysis, effectively harnessing complementary strengths humans CNNs.
Language: Английский