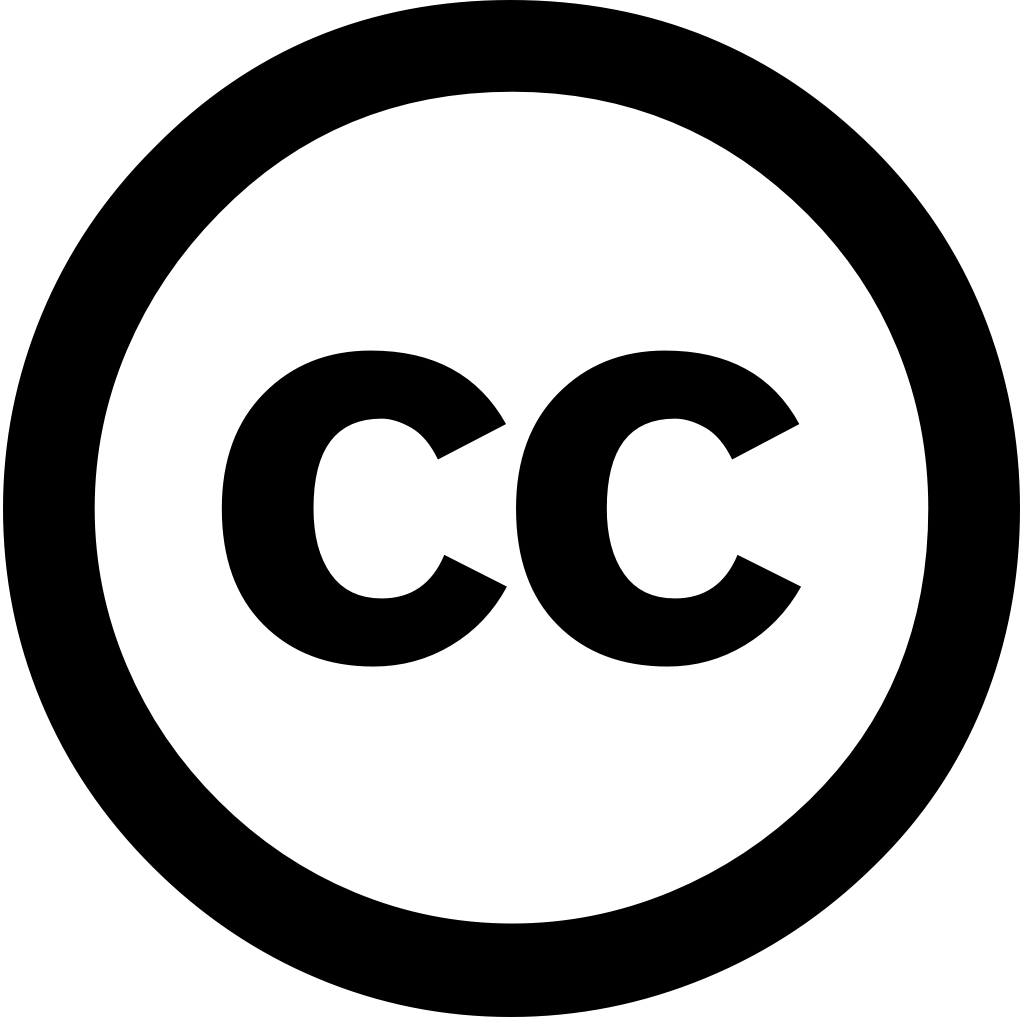
Tomography, Journal Year: 2025, Volume and Issue: 11(3), P. 38 - 38
Published: March 20, 2025
Background: Predictive models like Residual Neural Networks (ResNets) can use Magnetic Resonance Imaging (MRI) data to identify cervix tumors likely recur after radiotherapy (RT) with high accuracy. However, there persists a lack of insight into model selections (explainability). In this study, we explored whether features could be used generate simulated images as method explainability. Methods: T2W MRI were collected for twenty-seven women cancer who received RT from the TCGA-CESC database. Simulated generated follows: [A] ResNet was trained recurrent cancer; [B] evaluated on subjects obtain corresponding feature maps; [C] most important maps determined each image; [D] combined across all [E] final image reviewed by radiation oncologist and an initial algorithm likelihood recurrence. Results: (93% accuracy) images. passed through identified non-recurrent radiotherapy. A characteristics aggressive Cervical Cancer. These also contained multiple not considered clinically relevant. Conclusion: This simple able that mimicked tumor useful evaluating explainability predictive assist radiologists identification predict disease course.
Language: Английский