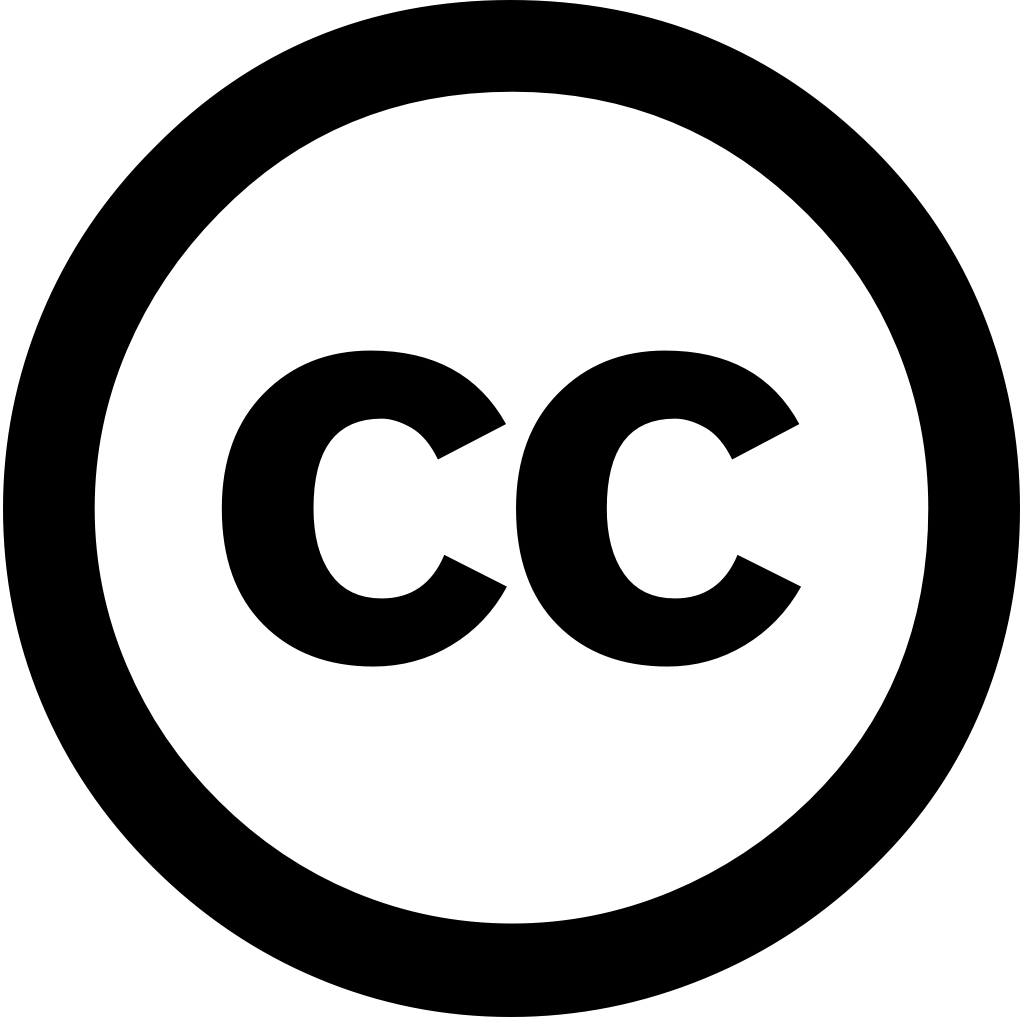
Drones, Journal Year: 2025, Volume and Issue: 9(4), P. 244 - 244
Published: March 25, 2025
Person re-identification (ReID) plays a crucial role in advancing UAV-based surveillance applications, enabling robust tracking and event analysis. However, existing methods UAV scenarios primarily focus on individual pedestrians, requiring cumbersome annotation efforts lacking seamless integration with ground-based systems. These limitations hinder the broader development of monitoring. To address these challenges, this paper proposes an Unsupervised Aerial–Ground Re-identification from Pedestrian to Group (UAGRPG) framework. Specifically, we introduce neighbor-aware collaborative learning (NCL) gradual graph matching (GGC) strategy uncover implicit associations between cross-modality groups unsupervised manner. Furthermore, develop association (CCAL) module bridge feature disparities achieve soft alignment across modalities. quantify optimal group similarity aerial ground domains, design minimum pedestrian distance transformation strategy. Additionally, new AG-GReID dataset, extensive experiments demonstrate that our approach achieves state-of-the-art performance both tasks aerial–ground scenarios, validating its effectiveness integrating surveillance.
Language: Английский