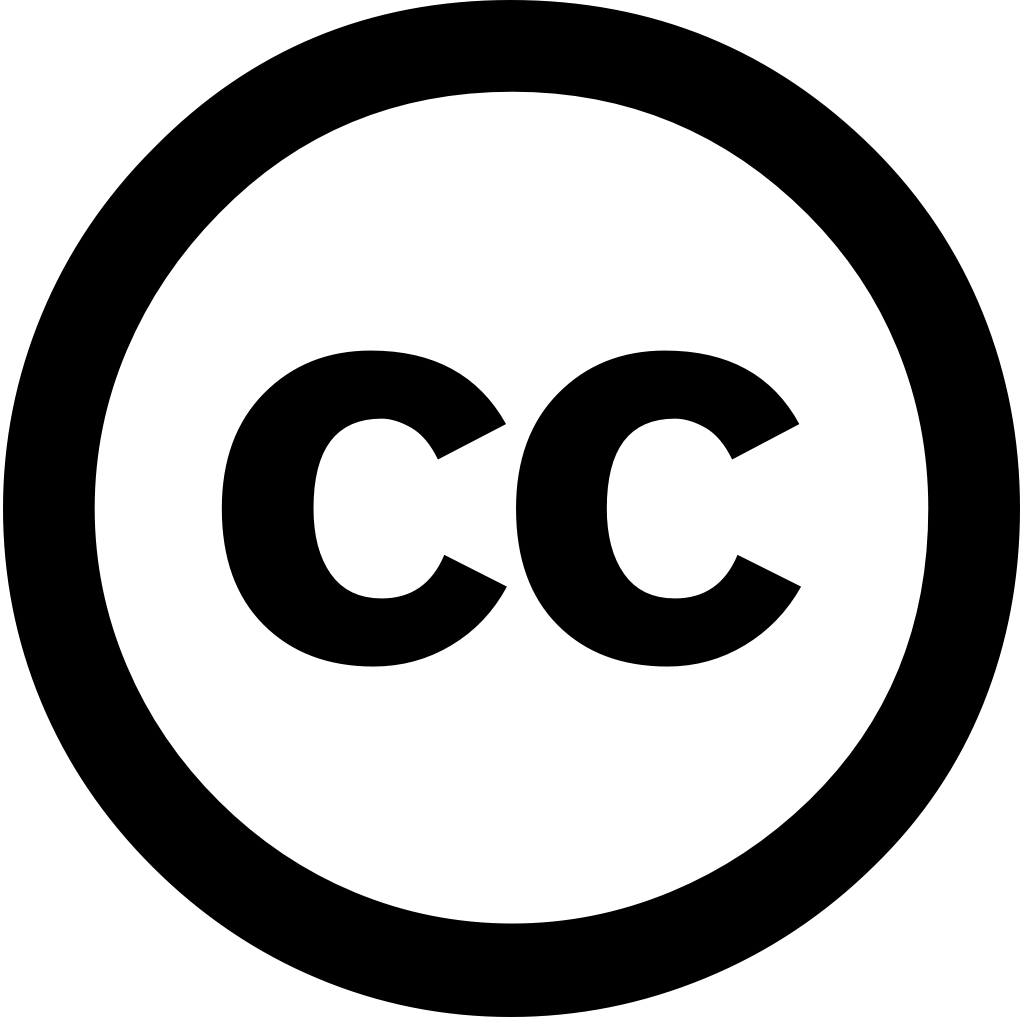
Energy Strategy Reviews, Journal Year: 2024, Volume and Issue: 56, P. 101602 - 101602
Published: Nov. 1, 2024
Language: Английский
Energy Strategy Reviews, Journal Year: 2024, Volume and Issue: 56, P. 101602 - 101602
Published: Nov. 1, 2024
Language: Английский
Acta Horticulturae, Journal Year: 2024, Volume and Issue: 1404, P. 277 - 284
Published: Sept. 1, 2024
Language: Английский
Citations
0Concurrency and Computation Practice and Experience, Journal Year: 2024, Volume and Issue: 37(2)
Published: Nov. 20, 2024
ABSTRACT 5G, 6G, and beyond networks promise to support vertical industrial services with strict QoS parameters, but the hardware‐based "one‐size‐fits‐all" model of legacy lacks flexibility needed for diverse services. The foundation 5G lies in softwarization, network slicing, Software Defined Networking (SDN), Network Function Virtualisation (NFV) serving as its core components. network‐slicing‐based shared environment necessitates an intelligent flexible resource management approach. In this case, traditional approaches are no longer suitable dealing a dynamic environment. With recent advancements, AI‐based have potential manage resources autonomously. This paradigm shift underscores need deep extensive investigation. However, existing literature on subject is fragmented cohesive overview slicing. To address these gaps, our review paper aims provide comprehensive scope slicing unified manner. sequence at first, presented conceptual enabling technologies, including SDN, NFV, edge computing. Secondly, identifies relevant phases presents traffic classification, admission, allocation, scheduling. Finally, it also discusses deployment slicing‐enabled key use cases their practical deployment, research gap, open challenges. best knowledge, first attempt critically analyze present consolidated state art modules cases. guide researchers developing innovative solutions assist players slices applications.
Language: Английский
Citations
0PeerJ Computer Science, Journal Year: 2024, Volume and Issue: 10, P. e2547 - e2547
Published: Nov. 22, 2024
Purpose This study aims to address the limitations of traditional data processing methods in predicting agricultural product prices, which is essential for advancing rural informatization enhance efficiency and support economic growth. Methodology The RL-CNN-GRU framework combines reinforcement learning (RL), convolutional neural network (CNN), gated recurrent unit (GRU) improve price predictions using multidimensional time series data, including historical weather, soil conditions, other influencing factors. Initially, model employs a 1D-CNN feature extraction, followed by GRUs capture temporal patterns data. Reinforcement further optimizes model, enhancing analysis accuracy inputs more reliable predictions. Results Testing on public proprietary datasets shows that significantly outperforms models with lower mean squared error (MSE) absolute (MAE) metrics. Conclusion contributes offering accurate prediction tool, thereby supporting improved decision-making processes fostering development.
Language: Английский
Citations
0Computers and Electronics in Agriculture, Journal Year: 2024, Volume and Issue: 228, P. 109665 - 109665
Published: Nov. 28, 2024
Language: Английский
Citations
0Energy Strategy Reviews, Journal Year: 2024, Volume and Issue: 56, P. 101602 - 101602
Published: Nov. 1, 2024
Language: Английский
Citations
0