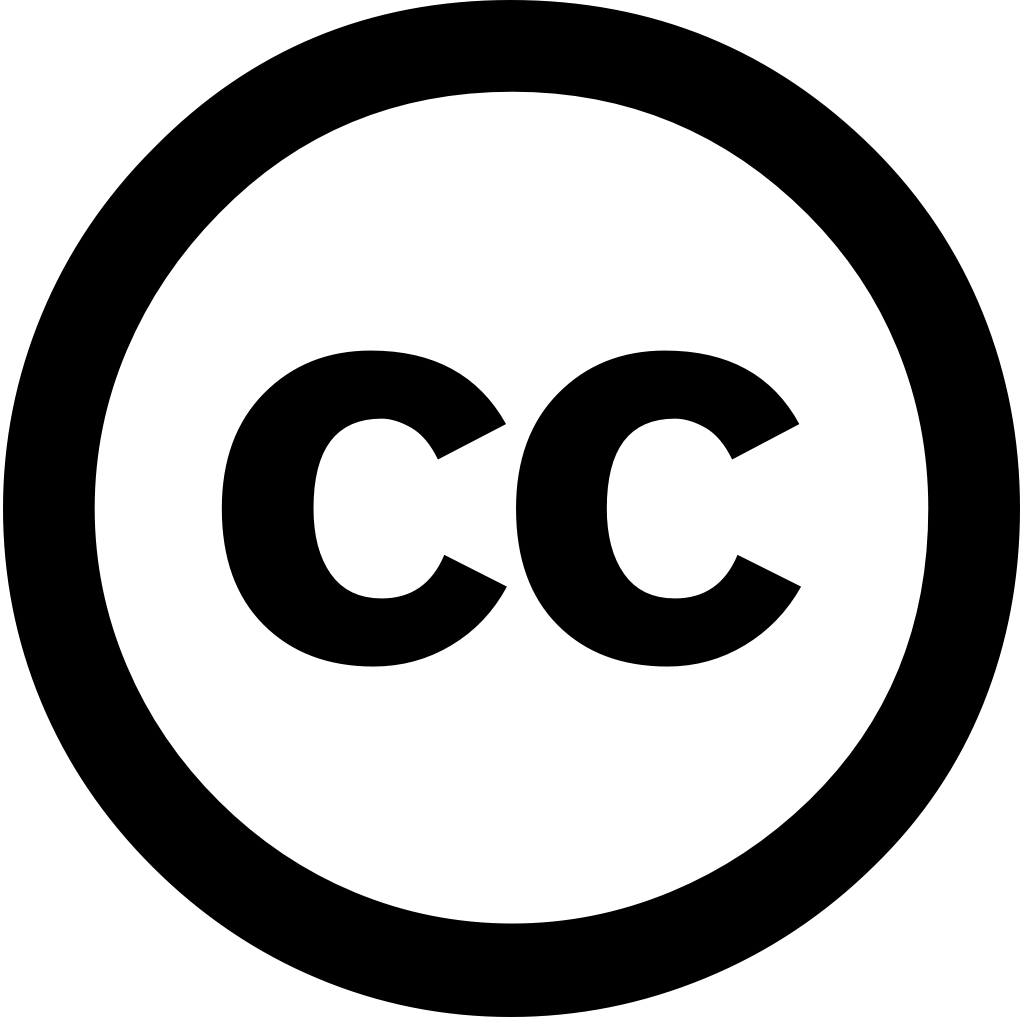
International Journal of Electrical and Electronics Engineering, Journal Year: 2024, Volume and Issue: 11(4), P. 198 - 205
Published: April 30, 2024
Underwater Wireless Sensor Networks (UWSNs) play a pivotal role in aquatic environments, facilitating data collection and communication for various applications. However, the limited energy resources of sensor nodes pose critical challenge, leading to emergence holes that can adversely impact network performance longevity. This research proposes novel two-part approach address this challenge by leveraging Neural both hole classification prediction UWSNs. The study begins with an in-depth literature review covering management UWSNs application deep learning techniques, particularly neural networks, predicting network-related issues. Through exploration, unique challenges associated underwater environments are identified, forming foundation proposed network-based solution. 1. Energy Hole Classification: Extensive simulations scenarios conducted classify instances holes. These generate rich dataset featuring crucial columns such as residual energy, hop distance from surface sink, zone, source address, destination etc. is meticulously prepared preprocessed ensure its suitability training model classification. 2. Prediction: phase then utilized train designed capture dependencies among features distance, addresses. trained evaluated on distinct test dataset, using metrics accuracy, precision, recall, F1 score measure success results showcase model's ability learn generalize extensive providing valuable insights into potential occurrences based specified features. paradigm, incorporating offers promising solution enhance UWSNs, ultimately improving longevity performance. concludes discussions implications results, real-world applications, avenues future intersection networks.
Language: Английский