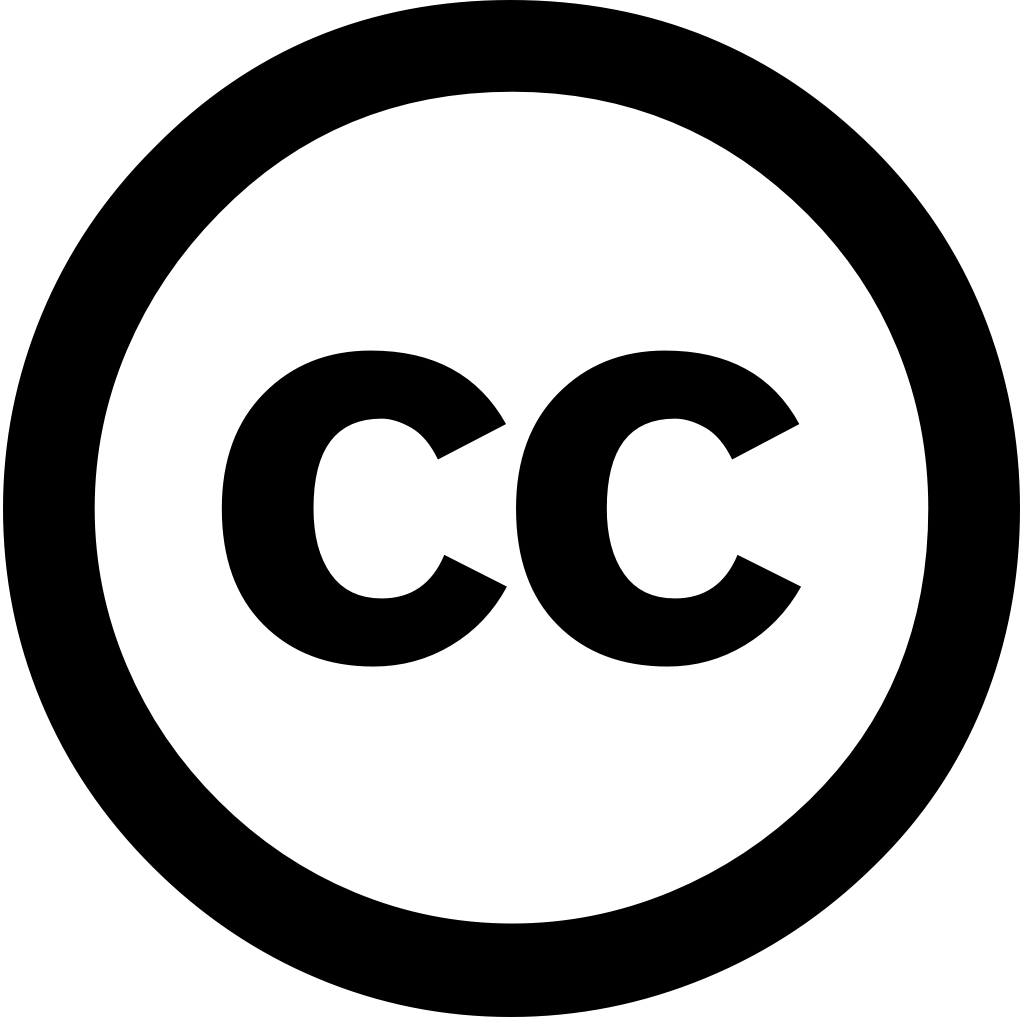
Research Square (Research Square), Journal Year: 2024, Volume and Issue: unknown
Published: Nov. 13, 2024
Language: Английский
Research Square (Research Square), Journal Year: 2024, Volume and Issue: unknown
Published: Nov. 13, 2024
Language: Английский
Applied Sciences, Journal Year: 2025, Volume and Issue: 15(10), P. 5725 - 5725
Published: May 20, 2025
Wind power plays a significant role in sustainable energy production, but the reliability of wind turbines depends heavily on integrity their gearboxes. Gearbox failures can lead to downtime and operational disruption. In this context, paper provides an overview evolution gearbox monitoring techniques, culminating emergence digital twin (DT) technology. We explore application DT technology condition monitoring, focusing two critical components: bearings gears. This includes comprehensive review methodologies involving model-based approaches data-driven techniques using signal processing (SP) artificial intelligence (AI) algorithms. address challenges “learning with minimal knowledge” propose framework for effective Finally, we discuss future research directions potential contributions advancing field through continued development implementation DT-based solutions.
Language: Английский
Citations
0Machines, Journal Year: 2024, Volume and Issue: 12(10), P. 729 - 729
Published: Oct. 15, 2024
The increasing complexity of modern mechanical systems, especially rotating machinery, demands effective condition monitoring techniques, particularly deep learning, to predict potential failures in a timely manner and enable preventative maintenance strategies. Health data analysis, widely used approach, faces challenges due randomness interpretation difficulties, highlighting the importance robust quality analysis for reliable monitoring. This paper presents two-part approach address these challenges. first part focuses on comprehensive preprocessing using only feature scaling selection via random forest (RF) algorithm, streamlining process by minimizing human intervention while managing complexity. second introduces Recurrent Expansion Network (RexNet) composed multiple layers built recursive expansion theories from multi-model learning. Unlike traditional Rex architectures, this unified framework allows fine tuning RexNet hyperparameters, simplifying their application. By combining with RexNet, methodology explores behaviors deeper interactions between dependent (e.g., health indicators) independent variables Remaining Useful Life (RUL)), offering richer insights than conventional methods. Both RF undergo hyperparameter optimization Bayesian methods under variability reduction (i.e., standard deviation) residuals, allowing algorithms reach optimal solutions enabling fair comparisons state-of-the-art approaches. Applied high-speed bearings large wind turbine dataset, achieves coefficient determination 0.9504, enhancing RUL prediction. more precise scheduling imperfect predictions, reducing downtime operational costs improving system reliability varying conditions.
Language: Английский
Citations
2Journal of Physics and Chemistry of Solids, Journal Year: 2024, Volume and Issue: unknown, P. 112526 - 112526
Published: Dec. 1, 2024
Language: Английский
Citations
2Journal of Imaging, Journal Year: 2024, Volume and Issue: 11(1), P. 2 - 2
Published: Dec. 24, 2024
Brain tumor detection is crucial in medical research due to high mortality rates and treatment challenges. Early accurate diagnosis vital for improving patient outcomes, however, traditional methods, such as manual Magnetic Resonance Imaging (MRI) analysis, are often time-consuming error-prone. The rise of deep learning has led advanced models automated brain feature extraction, segmentation, classification. Despite these advancements, comprehensive reviews synthesizing recent findings remain scarce. By analyzing over 100 papers past half-decade (2019-2024), this review fills that gap, exploring the latest methods paradigms, summarizing key concepts, challenges, datasets, offering insights into future directions using learning. This also incorporates an analysis previous targets three main aspects: results revealed primarily focuses on Convolutional Neural Networks (CNNs) their variants, with a strong emphasis transfer pre-trained models. Other Generative Adversarial (GANs) Autoencoders, used while Recurrent (RNNs) employed time-sequence modeling. Some integrate Internet Things (IoT) frameworks or federated real-time diagnostics privacy, paired optimization algorithms. However, adoption eXplainable AI (XAI) remains limited, despite its importance building trust diagnostics. Finally, outlines opportunities, focusing image quality, underexplored techniques, expanding deeper representations model behavior recurrent expansion advance imaging
Language: Английский
Citations
2Research Square (Research Square), Journal Year: 2024, Volume and Issue: unknown
Published: Nov. 13, 2024
Language: Английский
Citations
0