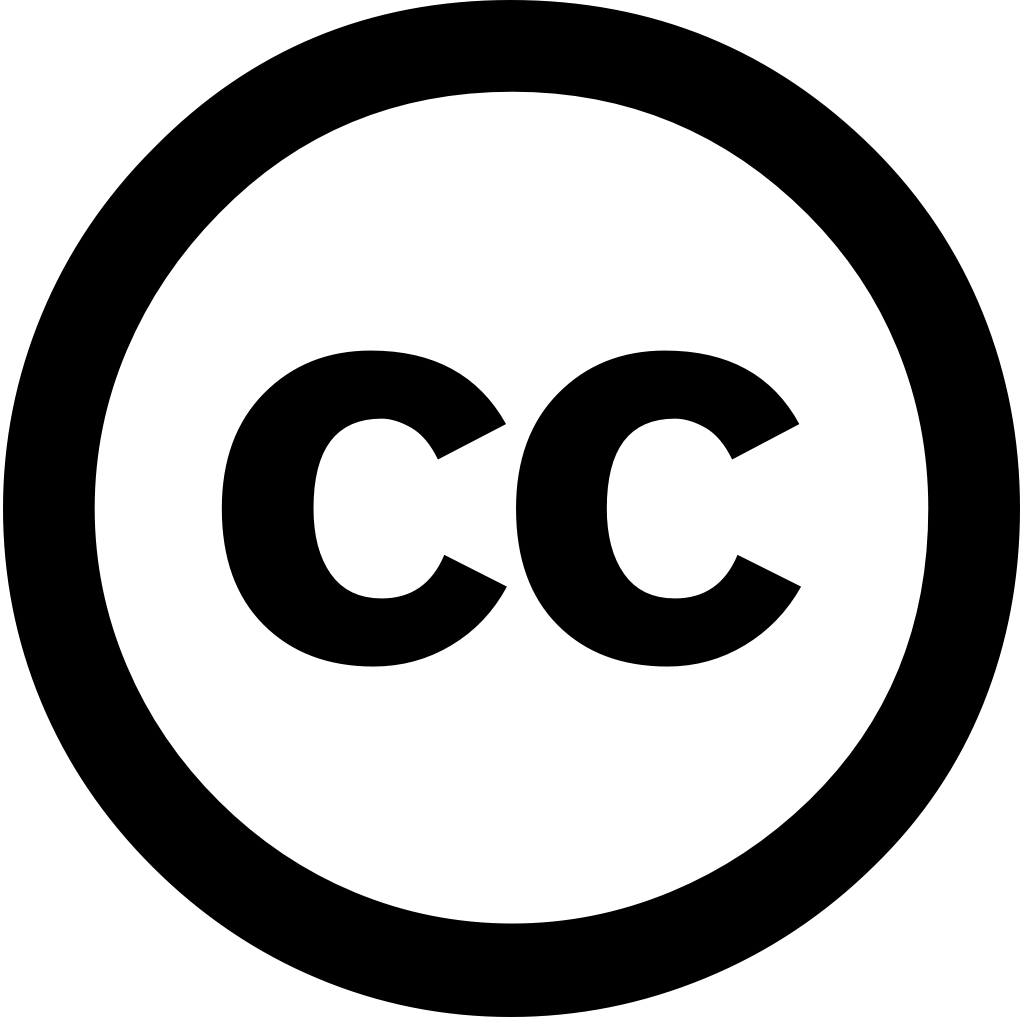
Journal of Computational Physics, Journal Year: 2024, Volume and Issue: unknown, P. 113656 - 113656
Published: Dec. 1, 2024
Language: Английский
Journal of Computational Physics, Journal Year: 2024, Volume and Issue: unknown, P. 113656 - 113656
Published: Dec. 1, 2024
Language: Английский
IEEE Sensors Journal, Journal Year: 2024, Volume and Issue: 24(19), P. 30690 - 30705
Published: Aug. 26, 2024
Language: Английский
Citations
38Physica Scripta, Journal Year: 2024, Volume and Issue: 99(6), P. 065215 - 065215
Published: April 18, 2024
Abstract The consequence of exothermic/endothermic chemical reactions and Arrhenius activation on the heat mass transport liquid flow past a cylinder in incidence magnetic dipole is considered current investigation. Magnetic dipoles are used medical applications such as magnotherapy spectroscopy, to produce static fields. Scientists engineers can improve effectiveness or transfer operations by analyzing impact building systems with optimized flows. modelled equations converted into non-dimensional ordinary differential (ODEs) using similarity variables. resultant solved employing physics-informed neural network (PINN) technique. Additionally, comparison PINN numerical method Runge–Kutta Fehlberg’s fourth-fifth order (RKF-45) studied. effects different parameters temperature, concentration, velocity profiles for endothermic/exothermic instances shown graphically. thermal, velocity, concentration get stronger curvature parameter values increase both endothermic exothermic cases. influence energy parameters, reaction thermal also depicted.
Language: Английский
Citations
28International Journal of Heat and Mass Transfer, Journal Year: 2025, Volume and Issue: 241, P. 126680 - 126680
Published: Jan. 10, 2025
Language: Английский
Citations
2Journal of Energy Storage, Journal Year: 2025, Volume and Issue: 111, P. 115363 - 115363
Published: Jan. 13, 2025
Language: Английский
Citations
2Journal of Computational Physics, Journal Year: 2025, Volume and Issue: unknown, P. 113837 - 113837
Published: Feb. 1, 2025
Language: Английский
Citations
2Fluids, Journal Year: 2023, Volume and Issue: 8(7), P. 212 - 212
Published: July 19, 2023
The significant growth of artificial intelligence (AI) methods in machine learning (ML) and deep (DL) has opened opportunities for fluid dynamics its applications science, engineering medicine. Developing AI encompass different challenges than with massive data, such as the Internet Things. For many scientific, biomedical problems, data are not massive, which poses limitations algorithmic challenges. This paper reviews ML DL research dynamics, presents discusses potential future directions.
Language: Английский
Citations
32IEEE Access, Journal Year: 2023, Volume and Issue: 12, P. 4597 - 4617
Published: Dec. 28, 2023
Advancements in digital automation for smart grids have led to the installation of measurement devices like phasor units (PMUs), micro-PMUs (μ-PMUs), and meters. However, large amount data collected by these bring several challenges as control room operators need use this with models make confident decisions reliable resilient operation cyber-power systems. Machine-learning (ML) based tools can provide a interpretation deluge obtained from field. For decision-makers ensure network under all operating conditions, identify solutions that are feasible satisfy system constraints, while being efficient, trustworthy interpretable. This resulted increasing popularity physics-informed machine learning (PIML) approaches, methods overcome model-based or data-driven ML face silos. work aims at following: a) review existing strategies techniques incorporating underlying physical principles power grid into different types approaches (supervised/semi-supervised learning, unsupervised reinforcement (RL)); b) explore works on PIML anomaly detection, classification, localization, mitigation transmission distribution systems, c) discuss improvements through consideration potential also addressing limitations them suitable real-world applications.
Language: Английский
Citations
28Industrial & Engineering Chemistry Research, Journal Year: 2023, Volume and Issue: 62(44), P. 18178 - 18204
Published: Oct. 26, 2023
Physics-Informed Machine Learning (PIML) is an emerging computing paradigm that offers a new approach to tackle multiphysics modeling problems prevalent in the field of chemical engineering. These often involve complex transport processes, nonlinear reaction kinetics, and coupling. This Review provides detailed account main contributions PIML with specific emphasis on momentum transfer, heat mass reactions. The progress method development (e.g., algorithm architecture), software libraries, applications coupling surrogate modeling) are detailed. On this basis, future challenges highlight importance developing more practical solutions strategies for PIML, including turbulence models, domain decomposition, training acceleration, modeling, hybrid geometry module creation.
Language: Английский
Citations
25AI, Journal Year: 2024, Volume and Issue: 5(3), P. 1534 - 1557
Published: Aug. 29, 2024
Physics-informed neural networks (PINNs) represent a significant advancement at the intersection of machine learning and physical sciences, offering powerful framework for solving complex problems governed by laws. This survey provides comprehensive review current state research on PINNs, highlighting their unique methodologies, applications, challenges, future directions. We begin introducing fundamental concepts underlying motivation integrating physics-based constraints. then explore various PINN architectures techniques incorporating laws into network training, including approaches to partial differential equations (PDEs) ordinary (ODEs). Additionally, we discuss primary challenges faced in developing applying such as computational complexity, data scarcity, integration Finally, identify promising Overall, this seeks provide foundational understanding PINNs within rapidly evolving field.
Language: Английский
Citations
15Physics of Fluids, Journal Year: 2024, Volume and Issue: 36(3)
Published: March 1, 2024
The physics-informed neural network (PINN) method is extended to learn and predict compressible steady-state aerodynamic flows with a high Reynolds number. To better the thin boundary layer, sampling distance function hard condition are explicitly introduced into input output layers of deep network, respectively. A gradient weight factor considered in loss implement PINN methods based on averaged Navier–Stokes (RANS) Euler equations, respectively, denoted as PINN–RANS PINN–Euler. Taking transonic flow around cylinder an example, these first verified for ability complex then applied global part physical data. When predicting velocity data local key regions, can always accurately field including layer wake, while PINN–Euler inviscid region. subsonic under different freestream Mach numbers (Ma∞= 0.3–0.7), fields predicted by both avoid inconsistency real phenomena pure data-driven method. insufficient shock identification capabilities. Since does not need second derivative, training time only 1/3 times that at same point network.
Language: Английский
Citations
13