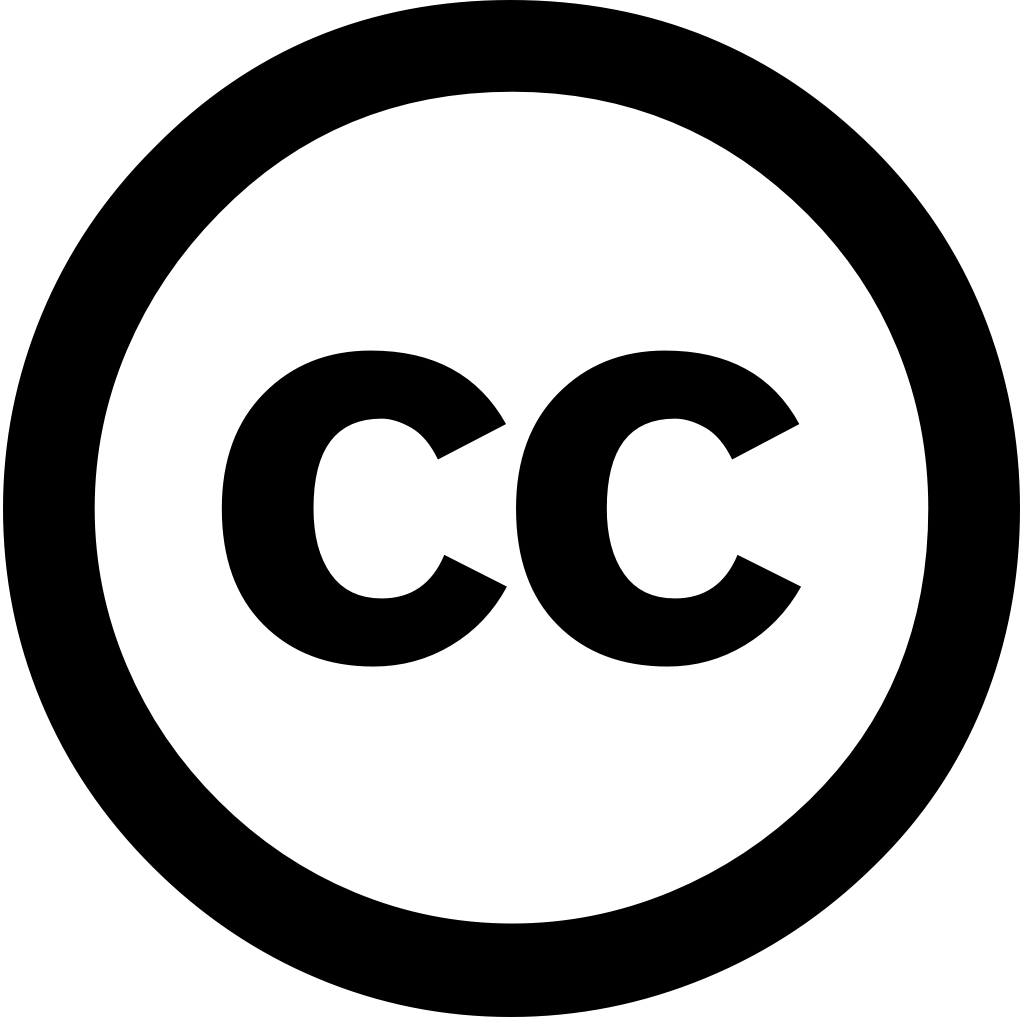
Journal of Computational Physics, Journal Year: 2024, Volume and Issue: unknown, P. 113656 - 113656
Published: Dec. 1, 2024
Language: Английский
Journal of Computational Physics, Journal Year: 2024, Volume and Issue: unknown, P. 113656 - 113656
Published: Dec. 1, 2024
Language: Английский
International Journal of Heat and Mass Transfer, Journal Year: 2024, Volume and Issue: 232, P. 125940 - 125940
Published: July 27, 2024
In this paper, a physics-informed neural network (PINN) technique is developed to study the heat and mass transfer for process of vapour bubble growth in superheated liquid domain tested using three working fluids including water, R-134a FC-72. The work represents novel step development PINNs phase change scenarios where surface tension effects dominate, acts as necessary validation stage before PINN techniques can be applied complex boiling analysis. Initially, forward analysis was performed water fluids. For each these investigations, algorithm trained on 50 % available CFD data. proposed able accurately infer velocity fields, particularly near-interfacial region. resultant circulatory flow found maintain desired circular shape growing bubbles. As result, when predicting evolution bubble, produced reduction peak error by 0.87 compared reference data, 3.42 prediction bubble. To test optimise learning capabilities methodology, an FC-72 predicted without supplying supporting observational scenario, within 1.3 unobserved approach confirms robustness methodologies method solving phase-change problems plays pivotal, promising expedite parametric studies practice. This pioneering effort applying current investigate domains, serving basis application benchmark inverse training strategy.
Language: Английский
Citations
10Measurement, Journal Year: 2025, Volume and Issue: unknown, P. 116728 - 116728
Published: Jan. 1, 2025
Language: Английский
Citations
1Journal of Computational Physics, Journal Year: 2025, Volume and Issue: unknown, P. 113843 - 113843
Published: Feb. 1, 2025
Language: Английский
Citations
1Scientific Reports, Journal Year: 2025, Volume and Issue: 15(1)
Published: Feb. 19, 2025
A better mechanical resistance and improved thermal conductivity, as compared to mono nano-liquids, can be attained by the ethylene glycol-based hybrid nanofluids. These fluids have substantial uses in several engineering systems. The main focus, recent work, is assess dynamics of nano-structured fluid via computational (CFD) machine learning (ML) approaches. nano-composition single-walled carbon nanotubes (SWCNTs) titanium dioxide (TiO2) glycol causes mixture SWCNTs-TiO2/EG. CFD model, for simulation procedure, developed incorporating similarity coordinates governing partial differential equations. This model comprises a dimensionless system having prime parameters problem. numerical results are appraised means comparison between present existing results. levenberg marquardt (LM) technique powerful tool predict flow properties. complex correlations input properties interpreted with help well LM neural network. this work might provide basis design development high-performance heat exchangers management
Language: Английский
Citations
1Physics of Fluids, Journal Year: 2025, Volume and Issue: 37(3)
Published: March 1, 2025
Computational fluid dynamics (CFD) is a powerful tool for modeling turbulent flow and commonly used urban microclimate simulations. However, traditional CFD methods are computationally intensive, requiring substantial hardware resources high-fidelity Deep learning (DL) models becoming popular as efficient alternatives, less computational to model complex non-linear interactions in A major drawback of DL that they prone error accumulation long-term temporal predictions, often compromising their accuracy reliability. To address this shortcoming, study investigates the use denoising diffusion probabilistic (DDPM) novel post-processing technique mitigate propagation models' sequential predictions. this, we employ convolutional autoencoder (CAE) U-Net architectures predict airflow around cubic structure. The DDPM then applied model's refining reconstructed fields better align with statistical results from large-eddy Results demonstrate that, although deep provide significant advantages over numerical solvers, susceptible predictions; however, utilizing step enhances by up 65% while maintaining three times speedup compared solvers. These findings highlight potential integrating transformative approach improving reliability learning-based simulations, paving way more scalable modeling.
Language: Английский
Citations
1Sensors, Journal Year: 2023, Volume and Issue: 23(19), P. 8124 - 8124
Published: Sept. 27, 2023
Prognostic and health management (PHM) plays a vital role in ensuring the safety reliability of aircraft systems. The process entails proactive surveillance evaluation state functional effectiveness crucial subsystems. principal aim PHM is to predict remaining useful life (RUL) subsystems proactively mitigate future breakdowns order minimize consequences. achievement this objective helped by employing predictive modeling techniques doing real-time data analysis. incorporation prognostic methodologies utmost importance execution condition-based maintenance (CBM), strategic approach that emphasizes prioritization repairing components have experienced quantifiable damage. Multiple are employed support advancement prognostics for aviation systems, encompassing physics-based modeling, data-driven techniques, hybrid prognosis. These enable prediction mitigation failures identifying relevant indicators. Despite promising outcomes sector pertaining implementation PHM, there exists deficiency research concerning efficient integration applications. primary paper provide thorough analysis current advancements with specific focus on prominent algorithms their practical applications challenges. concludes providing detailed prospective directions within field.
Language: Английский
Citations
18Scientific Reports, Journal Year: 2024, Volume and Issue: 14(1)
Published: Dec. 28, 2024
The rapid development of urbanization has led to a continuous rise in number elevators. This elevator failures from time time. At present, although there are some studies on fault diagnosis, they more or less limited by the lack data make research superficial. For such complex special equipment as elevator, it is difficult obtain reliable and sufficient train diagnosis model. To address this issue, paper first establishes numerical model vertical vibration for elevators with three degrees freedom. obtained motion equations then used constraints acquire simulated through PINNs. Next, proposed e-RGCN employed diagnosis. Finally, experimental validation shows that accuracy participation digital twins exceeds 90%, reaches 96.61%, significantly higher than other comparative models.
Language: Английский
Citations
8Journal of Chemical Information and Modeling, Journal Year: 2024, Volume and Issue: 64(3), P. 597 - 620
Published: Jan. 29, 2024
Artificial Neural Networks (ANNs) are transforming how we understand chemical mixtures, providing an expressive view of the space and multiscale processes. Their hybridization with physical knowledge can bridge gap between predictivity understanding underlying This overview explores recent progress in ANNs, particularly their potential 'recomposition' mixtures. Graph-based representations reveal patterns among mixture components, deep learning models excel capturing complexity symmetries when compared to traditional Quantitative Structure–Property Relationship models. Key such as Hamiltonian networks convolution operations, play a central role representing The integration ANNs Chemical Reaction Physics-Informed for inverse kinetic problems is also examined. combination sensors shows promise optical biomimetic applications. A common ground identified context statistical physics, where ANN-based methods iteratively adapt by blending initial states training data. concept recomposition unveils reciprocal inspiration reactive highlighting behaviors influenced environment.
Language: Английский
Citations
7Energies, Journal Year: 2023, Volume and Issue: 16(14), P. 5366 - 5366
Published: July 14, 2023
More than 3.5 billion people live in rural areas, where water and energy resources play an important role ensuring sustainable productive economies. This article reviews critically analyses the recent advances field of analogue reservoir computing that have been driven by unique physical properties waves. It also demonstrates computing, taken as independent research field, holds potential to bring artificial intelligence closer living outside large cities, thus enabling them enjoy benefits novel technologies are already place cities but not readily available or suitable for regional communities. In particular, although systems discussed main text universal terms processing input data making forecasts, they can be used design optimise power grid networks forecast consumption, both at local global scales. Thus, this review will interest a broad readership interested concepts machine learning their innovative practical applications diverse areas science technology.
Language: Английский
Citations
16ACM Transactions on Graphics, Journal Year: 2023, Volume and Issue: 42(6), P. 1 - 21
Published: Dec. 5, 2023
We introduce Neural Flow Maps, a novel simulation method bridging the emerging paradigm of implicit neural representations with fluid based on theory flow maps, to achieve state-of-the-art in-viscid phenomena. devise hybrid field representation, Spatially Sparse Fields (SSNF), which fuses small networks pyramid overlapping, multi-resolution, and spatially sparse grids, compactly represent long-term spatiotemporal velocity fields at high accuracy. With this buffer in hand, we compute long-term, bidirectional maps their Jacobians mechanistically symmetric manner, facilitate drastic accuracy improvement over existing solutions. These long-range, enable advection low dissipation, turn facilitates high-fidelity incompressible simulations that manifest intricate vortical structures. demonstrate efficacy our variety challenging scenarios, including leapfrogging vortices, colliding vortex reconnections, as well generation from moving obstacles density differences. Our examples show increased performance methods terms energy conservation, visual complexity, adherence experimental observations, preservation detailed
Language: Английский
Citations
14