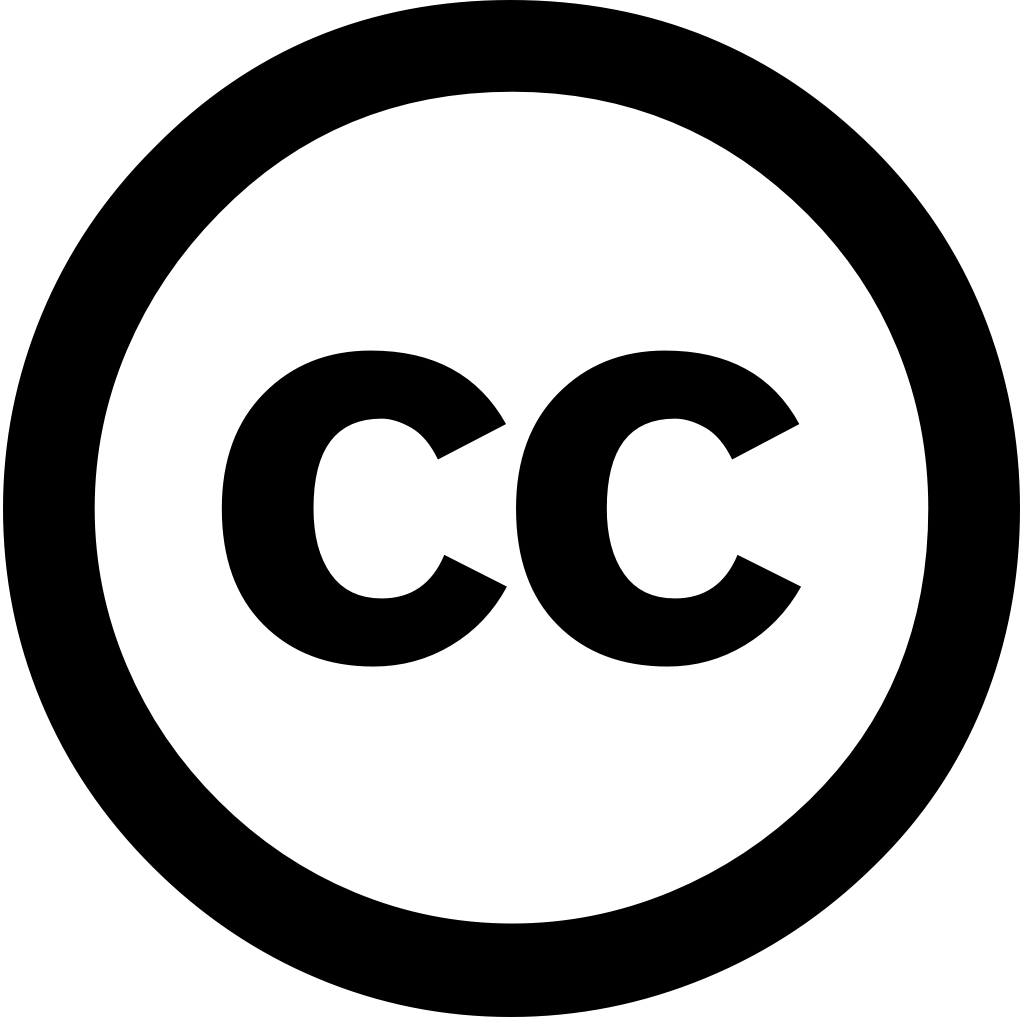
Energy and Buildings, Journal Year: 2025, Volume and Issue: unknown, P. 115729 - 115729
Published: April 1, 2025
Language: Английский
Energy and Buildings, Journal Year: 2025, Volume and Issue: unknown, P. 115729 - 115729
Published: April 1, 2025
Language: Английский
Engineering Applications of Computational Fluid Mechanics, Journal Year: 2024, Volume and Issue: 18(1)
Published: Aug. 23, 2024
This paper investigates the application of three nature-inspired optimisation algorithms – SHO, MFO, and GOA combined with four machine learning methods Gaussian Processes, Linear Regression, MLP, Random Forest to enhance carbon dioxide emission prediction in OECD Asia Oceania region. The study uses historical emissions data, socioeconomic indicators such as GDP, population density, energy consumption, urbanisation rates, environmental temperature, precipitation, forest cover. Through comprehensive experimentation, evaluates performance each combination, revealing varying effectiveness levels. MFO-MLP combination achieved highest accuracy R2 values 0.9996 0.9995 RMSE 11.7065 12.8890 for training testing datasets, respectively. GOA-MLP configuration 0.9994 0.99934 15.01306 14.59333. SHO-MLP while effective, showed lower 0.9915 0.9946 55.4516 41.575. findings suggest hybrid techniques can significantly compared conventional methods. research provides valuable insights policymakers stakeholders, indicating that optimised models support more informed effective policy-making sustainability efforts Future should explore additional ensemble improve robustness accuracy. These offer a robust tool forecast accurately, aiding developing targeted strategies reduce footprints achieve climate goals.
Language: Английский
Citations
5Polymers, Journal Year: 2024, Volume and Issue: 16(23), P. 3368 - 3368
Published: Nov. 29, 2024
The integration of machine learning (ML) into material manufacturing has driven advancements in optimizing biopolymer production processes. ML techniques, applied across various stages production, enable the analysis complex data generated throughout identifying patterns and insights not easily observed through traditional methods. As sustainable alternatives to petrochemical-based plastics, biopolymers present unique challenges due their reliance on variable bio-based feedstocks processing conditions. This review systematically summarizes current applications techniques aiming provide a comprehensive reference for future research while highlighting potential enhance efficiency, reduce costs, improve product quality. also shows role algorithms, including supervised, unsupervised, deep
Language: Английский
Citations
5E3S Web of Conferences, Journal Year: 2024, Volume and Issue: 531, P. 02001 - 02001
Published: Jan. 1, 2024
This paper focuses on the analysis of shift towards smart energy technologies in a post-COVID era marking and describing it as process transition from crisis to an immense opportunity. The COVID-19 pandemic with its lockdowns social distancing made people spend more time indoors helping them realize extent climate change global warming their effect human lives. In addition, also caused noticeable consumer behaviour consumption re-thinking efficiency. Central this transformation is increasing adoption technologies, which are playing pivotal role enhancing efficiency within households across communities. Our demonstrates how not only lead significant savings utility bills but reduce environmental impacts by lowering carbon emissions increase acceptance novel technologies. Additionally, they make consumers worldwide aware ways solutions behavioural trends minimize negative economic activities environment via adapting green shifting direction renewable solutions.
Language: Английский
Citations
4Journal of Hazardous Materials Advances, Journal Year: 2024, Volume and Issue: unknown, P. 100480 - 100480
Published: Sept. 1, 2024
Language: Английский
Citations
4International Journal of Pressure Vessels and Piping, Journal Year: 2025, Volume and Issue: unknown, P. 105428 - 105428
Published: Jan. 1, 2025
Language: Английский
Citations
0International Journal of Innovative Research in Computer Science & Technology, Journal Year: 2025, Volume and Issue: 13(1), P. 12 - 18
Published: Jan. 1, 2025
The rapid proliferation of Internet Things (IoT) devices within cloud environments has introduced unprecedented challenges in securing network infrastructures against anomalies and cyber threats. Traditional detection mechanisms often struggle to meet the dynamic complex demands these integrated ecosystems. This review paper focuses on potential deep learning (DL)-based optimized models for effective anomaly IoT-enabled environments. It examines fundamental role DL techniques addressing key challenges, including scalability, adaptability, real-time threat identification. systematically explores state-of-the-art models, highlighting their architectures, optimization strategies, performance metrics. A comparative analysis is provided underscore strengths, limitations, suitability across diverse use cases. Furthermore, emerging trends, such as lightweight federated learning, are discussed context resource-constrained IoT networks. aims offer researchers practitioners insights into current advancements while identifying gaps future directions research enhancing security reliability IoT-cloud highlights detecting IoT-integrated environments, focusing strategies handle like heterogeneity, detection. We provide a concise existing approaches methods, identify suggest research.
Language: Английский
Citations
0Frontiers in Energy Research, Journal Year: 2025, Volume and Issue: 12
Published: Jan. 16, 2025
This paper proposes a short-term wind and photovoltaic power forecasting framework considering time-frequency decomposition based on bidirectional long memory networks. First, the seasonal trend using loess is applied to original data for time domain decomposition, obtaining trend, seasonal, residual components. Then, component undergoes variational mode further extract features of different frequencies. Next, maximum information coefficient used select features, which highly correlated with as input prediction model. Finally, selected are into networks training prediction. Experimental validation actual from station in Hebei Province, China July August 2023, shows that proposed method achieves high accuracy reliability output The smallest root mean square error 0.92 absolute 0.58 prediction, at same time, 67.5 48.16 significantly outperforming other methods.
Language: Английский
Citations
0IGI Global eBooks, Journal Year: 2025, Volume and Issue: unknown, P. 1 - 26
Published: Feb. 7, 2025
Climate change and the rapid depletion of natural resources present significant global challenges that demand innovative sustainable solutions. Traditional resource management approaches are increasingly inadequate in addressing these complexities, creating a pressing need for advanced technologies. Artificial Intelligence (AI) Data Science have emerged as powerful tools to revolutionize green technologies, enhancing their efficiency effectiveness promoting sustainability. This chapter provides comprehensive exploration applications AI discussing potential impacts, challenges, ethical considerations. By examining aspects, aims illuminate how technologies can be harnessed address environmental support future.
Language: Английский
Citations
0Lecture notes on data engineering and communications technologies, Journal Year: 2025, Volume and Issue: unknown, P. 889 - 901
Published: Jan. 1, 2025
Language: Английский
Citations
0Energy Informatics, Journal Year: 2025, Volume and Issue: 8(1)
Published: Feb. 27, 2025
Language: Английский
Citations
0