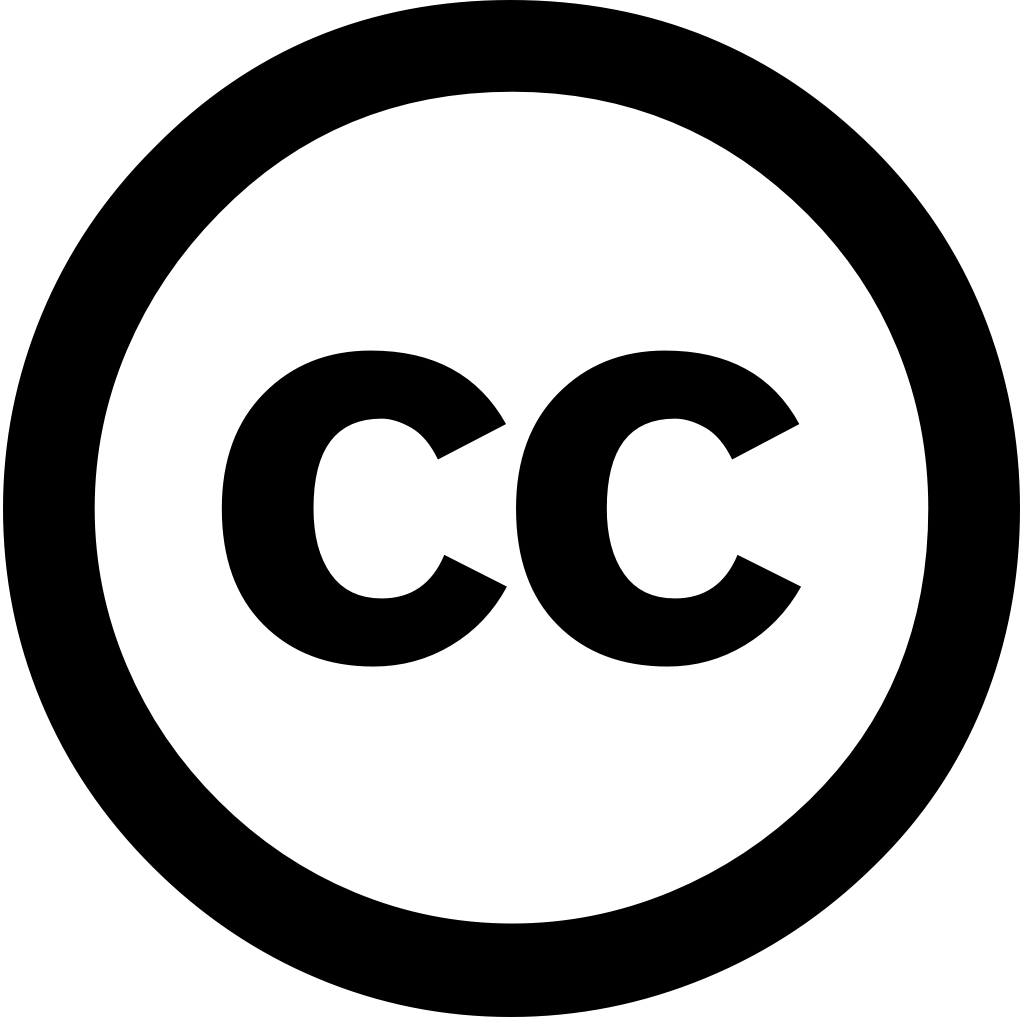
Research Square (Research Square), Journal Year: 2024, Volume and Issue: unknown
Published: Dec. 12, 2024
Language: Английский
Research Square (Research Square), Journal Year: 2024, Volume and Issue: unknown
Published: Dec. 12, 2024
Language: Английский
Energies, Journal Year: 2025, Volume and Issue: 18(2), P. 407 - 407
Published: Jan. 18, 2025
Advanced deep learning algorithms play a key role in optimizing energy usage smart cities, leveraging massive datasets to increase efficiency and sustainability. These analyze real-time data from sensors IoT devices predict demand, enabling dynamic load balancing reducing waste. Reinforcement models optimize power distribution by historical patterns adapting changes real time. Convolutional neural networks (CNNs) recurrent (RNNs) facilitate detailed analysis of spatial temporal better usage. Generative adversarial (GANs) are used simulate scenarios, supporting strategic planning anomaly detection. Federated ensures privacy-preserving sharing distributed systems, promoting collaboration without compromising security. technologies driving the transformation towards sustainable energy-efficient urban environments, meeting growing demands modern cities. However, there is view that if pace development maintained with large amounts data, computational/energy costs may exceed benefits. The article aims conduct comparative assess potential this group technologies, taking into account efficiency.
Language: Английский
Citations
2Energies, Journal Year: 2023, Volume and Issue: 16(24), P. 7988 - 7988
Published: Dec. 9, 2023
This paper provides a comprehensive review of solutions based on artificial intelligence (AI) in the urban energy sector, with focus their applications and impacts. The study employed literature methodology to analyze recent research AI’s role energy-related solutions, covering years 2019 2023. authors classified publications according main focus, resulting two key areas AI implementation: residential individual user applications, infrastructure integration for society. objectives this are following: O1: identify trends, emerging technologies, using field; O2: provide up-to-date insights into use applications; O3: gain understanding current state AI-driven solutions; O4: explore future directions, challenges field solutions. contributes deeper transformative potential management, providing valuable directions researchers practitioners field. Based results, it can be claimed that connected at homes is used following areas: heating cooling, lighting, windows blinds, home devices, management systems. integrating through solutions: enhancement electric vehicle charging infrastructure, reduction emissions, development smart grids, efficient storage. What more, latest associated implementation include need balance resident comfort efficiency homes, ensuring compatibility cooperation among various preventing unintended consumption increases due constant connectivity, renewable sources, coordination consumption.
Language: Английский
Citations
40Internet of Things, Journal Year: 2024, Volume and Issue: 26, P. 101148 - 101148
Published: March 2, 2024
Language: Английский
Citations
12Applied Sciences, Journal Year: 2025, Volume and Issue: 15(4), P. 1781 - 1781
Published: Feb. 10, 2025
Three-dimensional (3D) printing techniques already enable the precise deposition of many materials, becoming a promising approach for materials engineering, mechanical or biomedical engineering. Recent advances in 3D scientists and engineers to create models with precisely controlled complex microarchitecture, shapes, surface finishes, including multi-material printing. The incorporation artificial intelligence (AI) at various stages has made it possible reconstruct objects from images (including, example, medical images), select optimize process, monitor lifecycle products. New emerging opportunities are provided by ability machine learning (ML) analyze data sets learn previous (historical) experience predictions dynamically individuate products processes. This includes synergistic capabilities ML development personalized
Language: Английский
Citations
1Applied Sciences, Journal Year: 2025, Volume and Issue: 15(6), P. 3166 - 3166
Published: March 14, 2025
Generative AI (GenAI) is revolutionizing digital twins (DTs) for fault diagnosis and predictive maintenance in Industry 4.0 5.0 by enabling real-time simulation, data augmentation, improved anomaly detection. DTs, virtual replicas of physical systems, already use generative models to simulate various failure scenarios rare events, improving system resilience prediction accuracy. They create synthetic datasets that improve training quality while addressing scarcity imbalance. The aim this paper was present the current state art perspectives using AI-based DTs 4.0/5.0. With GenAI, enable proactive minimize downtime, their latest implementations combine multimodal sensor generate more realistic actionable insights into performance. This provides operational profiles, identifying potential traditional methods may miss. New area include incorporation Explainable (XAI) increase transparency decision-making reliability key industries such as manufacturing, energy, healthcare. As emphasizes a human-centric approach, DT can seamlessly integrate with human operators support collaboration decision-making. implementation edge computing increases scalability capabilities smart factories industrial Internet Things (IoT) systems. Future advances federated learning ensure privacy exchange between enterprises diagnostics, evolution GenAI alongside ensuring long-term validity. However, challenges remain managing computational complexity, security, ethical issues during implementation.
Language: Английский
Citations
1Energy, Journal Year: 2024, Volume and Issue: 298, P. 131312 - 131312
Published: April 15, 2024
Language: Английский
Citations
7Electronics, Journal Year: 2024, Volume and Issue: 13(16), P. 3338 - 3338
Published: Aug. 22, 2024
Energy efficiency in production systems and processes is a key global research topic, especially light of the Green Deal, Industry 4.0/5.0 paradigms, rising energy prices. Research on improving based artificial intelligence (AI) analysis brings promising solutions, digital transformation industry towards green slowly becoming reality. New planning rules, optimization use Industrial Internet Things (IIoT), industrial cyber-physical (ICPSs), effective data their with AI bring further opportunities for sustainable, energy-efficient production. The aim this study to systematically evaluate quantify results, trends, impact management AI-based demand forecasting. value includes broader which will reduce observed environmental economic problems areas reducing consumption, forecasting accuracy, efficiency. In addition, technologies creating sustainable environment, accuracy forecasts, including area electricity storage, increase. A emerging trend manufacturing optimize waste, increase sustainability. An innovative perspective that leverages AI’s ability accurately forecast allows manufacturers align consumption schedules, minimizing excess emissions. Advanced machine learning (ML) algorithms can integrate real-time from various sources, such as weather patterns market demand, improve accuracy. This supports both sustainability enable more dynamic responsive systems, paving way smarter, resilient processes. paper’s contribution goes beyond mere description, making analyses, comparisons, generalizations leading current literature, logical conclusions state-of-the-art, authors’ knowledge experience renewable energy, AI, mechatronics.
Language: Английский
Citations
7Energies, Journal Year: 2023, Volume and Issue: 16(24), P. 8059 - 8059
Published: Dec. 14, 2023
The ability of artificial intelligence (AI) to process large amounts data, analyze complex patterns, and make predictions is driving innovation in the energy sector transformation markets. It helps optimize operations, improve efficiency, reduce costs, accelerate transition cleaner more sustainable sources. AI playing an increasingly important role transforming markets various aspects industry different ways, including smart grids management, renewable integration, forecasting trading, demand response load efficiency conservation, maintenance asset storage optimization, carbon emission reduction, market analytics risk exploration production, regulatory compliance, safety. aim this article discuss our own AI-based computational model lay foundations for further harmonious development based on a (AI/ML-based) models, with particular reference current limitations priority directions research. Such approach may encourage new research practical application algorithms critical domains sector.
Language: Английский
Citations
12Journal of Cleaner Production, Journal Year: 2025, Volume and Issue: unknown, P. 144752 - 144752
Published: Jan. 1, 2025
Language: Английский
Citations
0Applied Sciences, Journal Year: 2025, Volume and Issue: 15(4), P. 2219 - 2219
Published: Feb. 19, 2025
The integration of artificial intelligence (AI) with additive manufacturing (AM) is driving breakthroughs in personalized rehabilitation and physical therapy solutions, enabling precise customization to individual patient needs. This article presents the current state knowledge perspectives using solutions for physiotherapy thanks introduction AI AM. Advanced algorithms analyze patient-specific data such as body scans, movement patterns, medical history design customized assistive devices, orthoses, prosthetics. synergy enables rapid prototyping production highly optimized improving comfort, functionality, therapeutic outcomes. Machine learning (ML) models further streamline process by anticipating biomechanical needs adapting designs based on feedback, providing iterative refinement. Cutting-edge techniques leverage generative topology optimization create lightweight yet durable structures that are ideally suited patient’s anatomy goals .AI-based AM also facilitates multi-material devices combine flexibility, strength, sensory capabilities, improved monitoring support during therapy. New include integrating smart sensors printed real-time collection feedback loops adaptive Additionally, these becoming increasingly accessible technology lowers costs improves, democratizing healthcare. Future advances could lead widespread use digital twins simulation before production. AI-based virtual reality (VR) augmented (AR) tools expected provide immersive, training environments along aids. Collaborative platforms federated can enable healthcare providers researchers securely share insights, accelerating innovation. However, challenges regulatory approval, security, ensuring equity access technologies must be addressed fully realize their potential. One major gaps lack large, diverse datasets train models, which limits ability span different demographics conditions. Integration AI–AM systems into should focus processing techniques.
Language: Английский
Citations
0