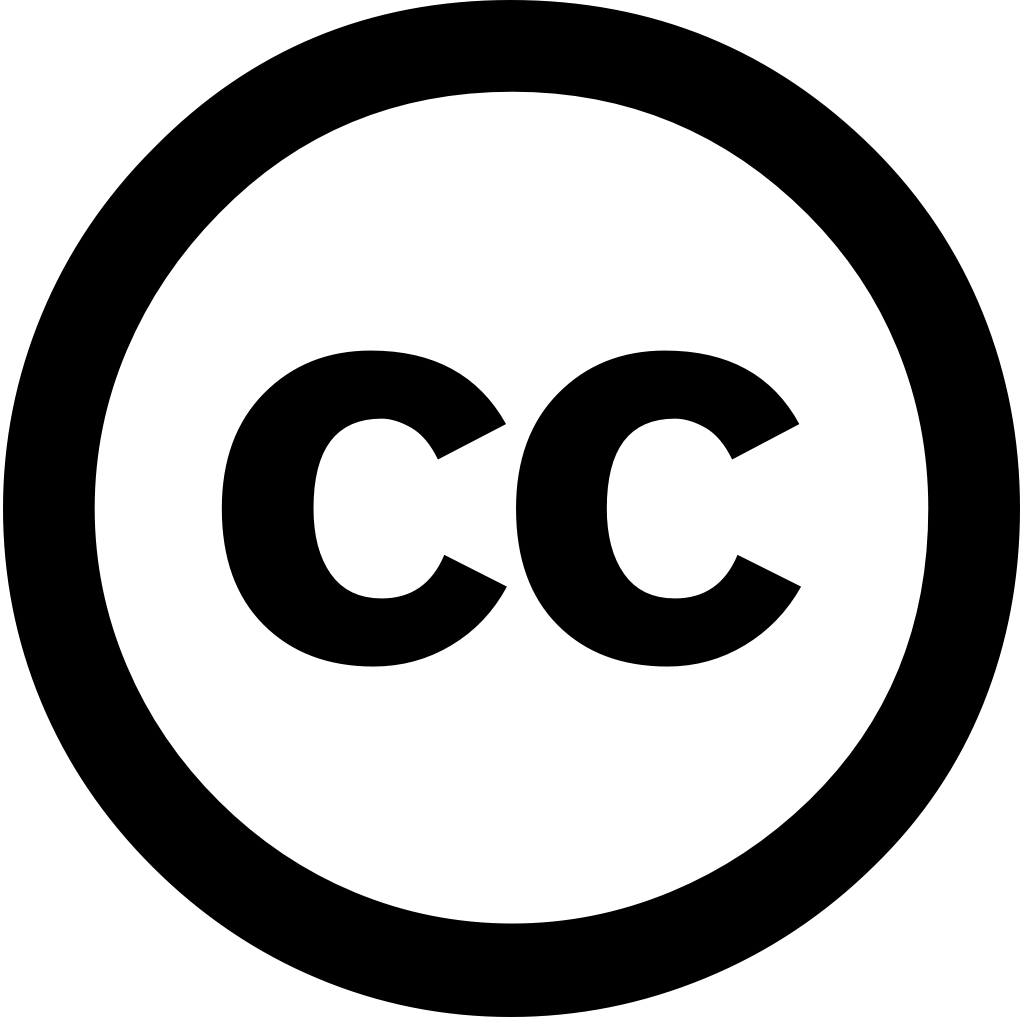
Algorithms, Journal Year: 2025, Volume and Issue: 18(3), P. 132 - 132
Published: March 2, 2025
This paper presents an adaptive Machine Learning (ML)-based framework for automatic load optimization in Connected Smart Green Townhouses (CSGTs) The system dynamically optimizes consumption and transitions between grid-connected island modes. Automatic mode reduce the need manual changes, ensuring reliable operation. Actual occupancy, demand, weather, energy price data are used to manage loads which improves efficiency, cost savings, sustainability. An is employed that combines processing ML. A hybrid Long Short-Term Memory-Convolutional Neural Network (LSTM-CNN) model analyze time series spatial data. Multi-Objective Particle Swarm Optimization (MOPSO) balance costs, carbon emissions, efficiency. results obtained show a 3–5% improvement efficiency 10–12% mode, as well 4–6% reduction emissions.
Language: Английский